Online ranking combination
Proceedings of the 13th ACM Conference on Recommender Systems(2019)
摘要
As a task of high importance for recommender systems, we consider the problem of learning the convex combination of ranking algorithms by online machine learning. In the case of two base rankers, we show that the exponentially weighted combination achieves near optimal performance. However, the number of required points to be evaluated may be prohibitive with more base models in a real application. We propose a gradient based stochastic optimization algorithm that uses finite differences. Our new algorithm achieves similar empirical performance for two base rankers, while scaling well with an increased number of models. In our experiments with five real-world recommendation data sets, we show that the combination offers significant improvement over previously known stochastic optimization techniques. Our algorithm is the first effective stochastic optimization method for combining ranked recommendation lists by online machine learning.
更多查看译文
关键词
RFDSA, combination, ranking
AI 理解论文
溯源树
样例
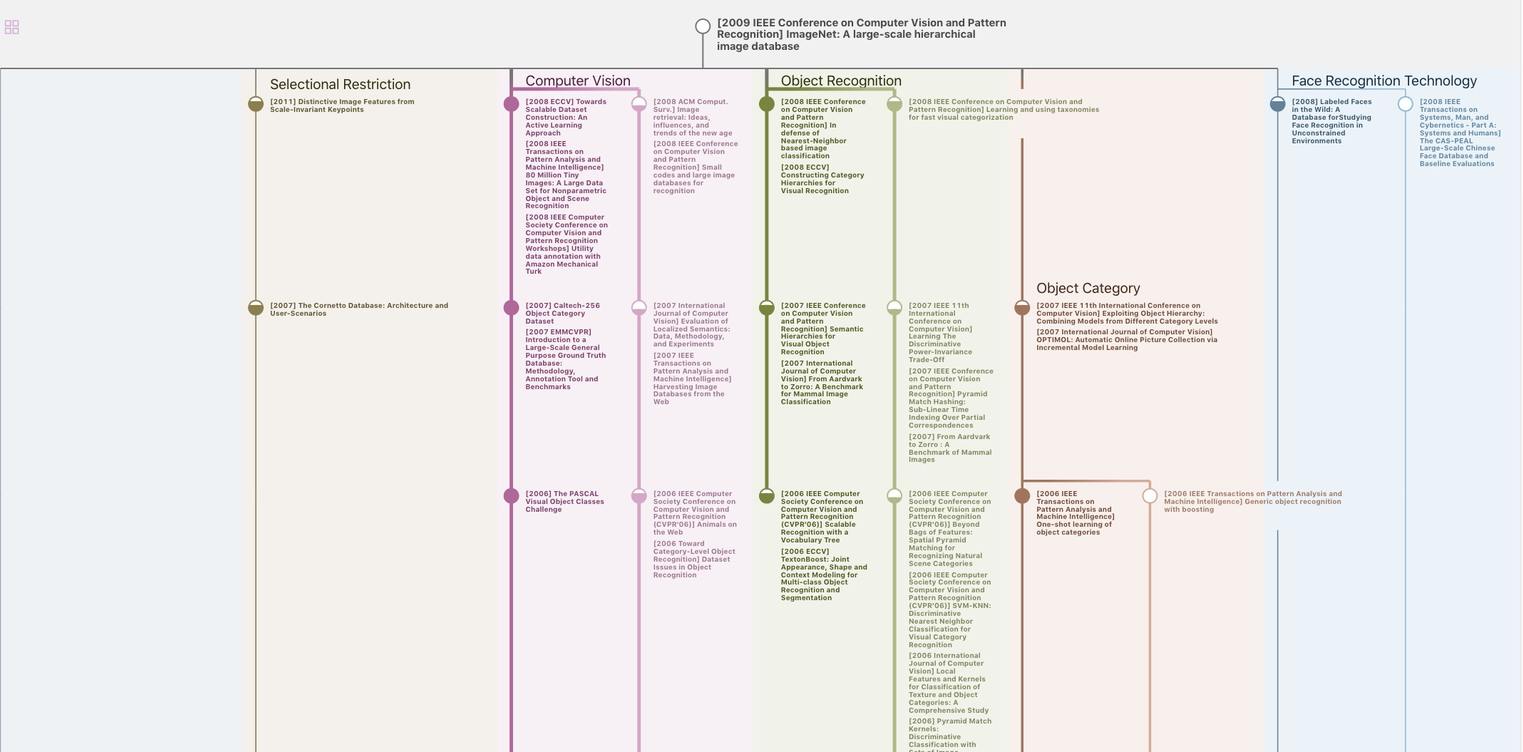
生成溯源树,研究论文发展脉络
Chat Paper
正在生成论文摘要