Real Time Attack Detection with Deep Learning
2019 16th Annual IEEE International Conference on Sensing, Communication, and Networking (SECON)(2019)
摘要
The Internet of Things (IoT) significantly extends the attack surface of the Internet, making the use of an Anomaly-based Intrusion Detection System of paramount importance. Despite in the last years big research efforts have focused on the application of Deep Learning techniques to attack detection, an ultimate real-time solution, able to provide a high detection rate with an acceptable false alarm rate while processing raw network traffic in real time, has still to be identified. For this reason, in this paper we propose an Intrusion Detection System that, leveraging on probabilistic data structures and Deep Learning, is able to process in real time the traffic collected in a backbone network, offering almost optimal detection performance and low false alarm rate. Indeed, the extensive experimental tests, run to validate and evaluate our system, confirm that, with a proper parameter setting, we can achieve about 90% of detection rate, with an accuracy of 0.871.
更多查看译文
关键词
IoT,attack surface,raw network traffic,probabilistic data structures,backbone network,optimal detection performance,anomaly-based intrusion detection system,deep learning techniques,false alarm rate,Internet of Things
AI 理解论文
溯源树
样例
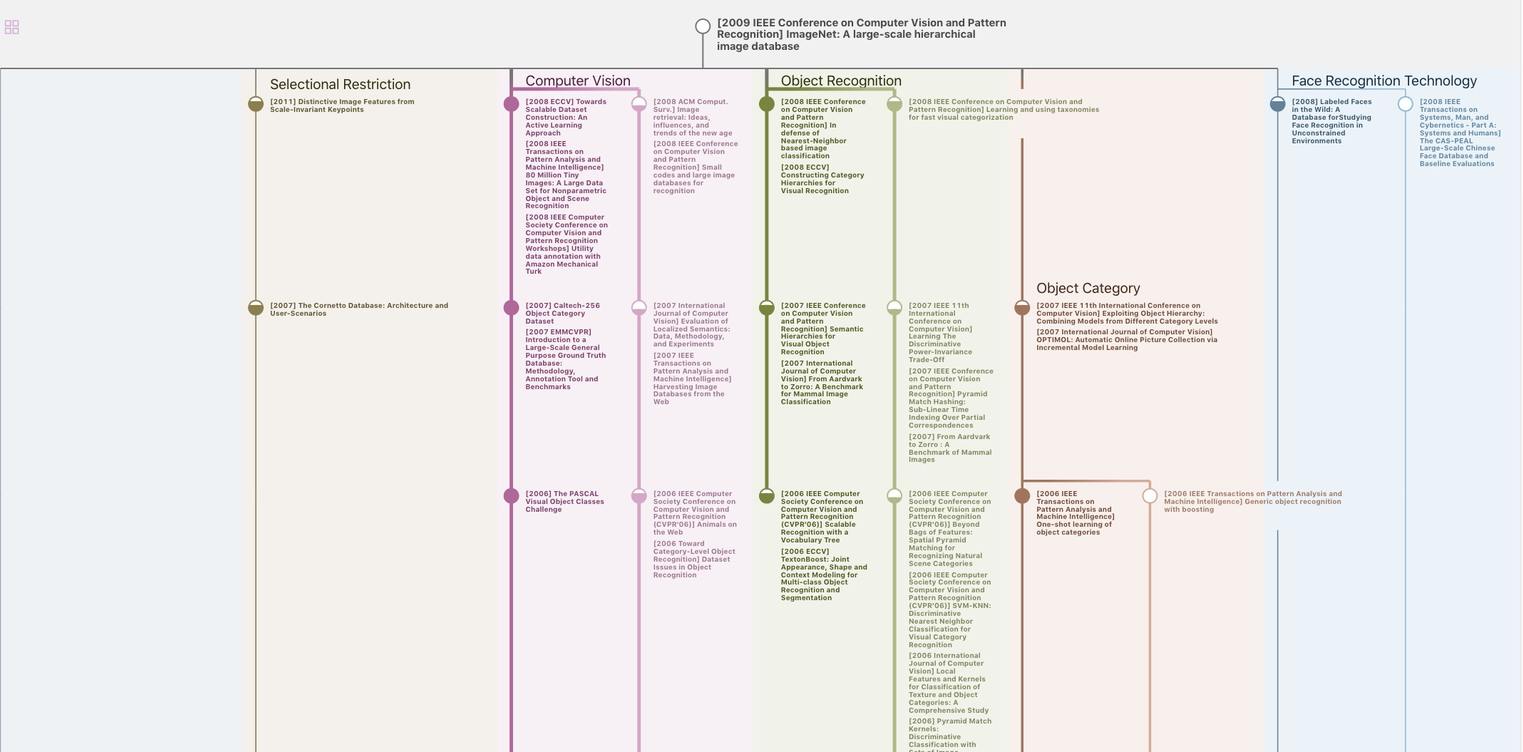
生成溯源树,研究论文发展脉络
Chat Paper
正在生成论文摘要