Block-Decentralized Model-Free Reinforcement Learning Control of Two Time-Scale Networks.
ACC(2019)
摘要
In this paper, we present a cluster-wise decentralized model-free reinforcement learning (RL) based control design for a linear time-invariant consensus network. We assume that the fast dynamics of the network is stable and design the control to shape the slow dynamics. The design exploits timescale separation properties inherent in the slow dynamics of the clusters and the weak couplings between the clusters. The aggregated slow variable from each cluster is used for feedback and decentralized controllers are learned for each cluster. Using singular perturbation theory, we show the sub-optimality of the learned controller and provide closed-loop stability conditions. We prove that this decentralized learning design will produce close-to-optimal performance if the clustering is strong with weak inter-cluster couplings. This design reduces the learning time and the amount of communication links required. The effectiveness of the design is demonstrated using a numerical example.
更多查看译文
关键词
Clustered network,Reinforcement learning,Adaptive dynamic programming,Block-decentralized control,Two time-scale
AI 理解论文
溯源树
样例
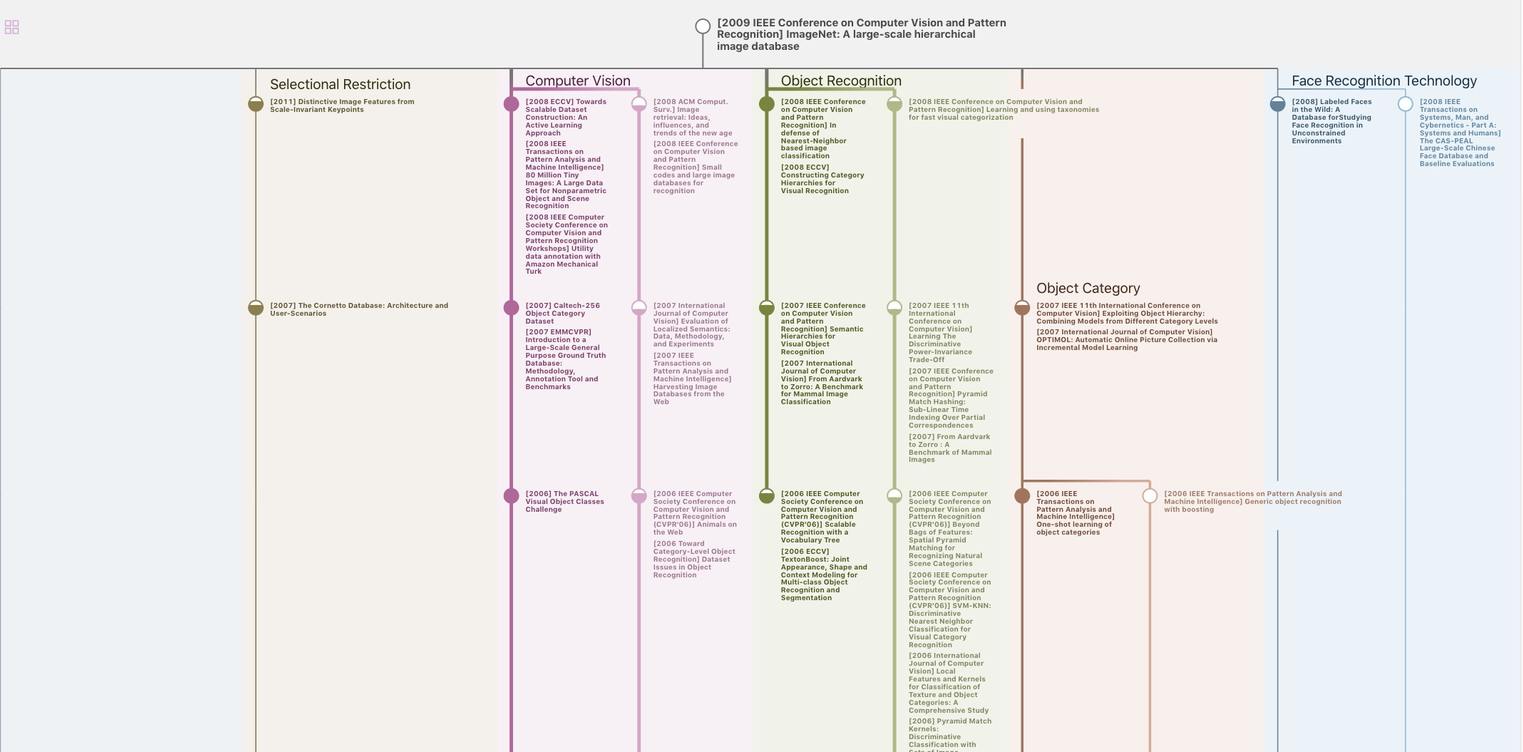
生成溯源树,研究论文发展脉络
Chat Paper
正在生成论文摘要