Distributed Equivalent Substitution Training for Large-Scale Recommender Systems
SIGIR '20: The 43rd International ACM SIGIR conference on research and development in Information Retrieval Virtual Event China July, 2020(2020)
摘要
We present Distributed Equivalent Substitution (DES) training, a novel distributed training framework for large-scale recommender systems with dynamic sparse features. DES introduces fully synchronous training to large-scale recommendation system for the first time by reducing communication, thus making the training of commercial recommender systems converge faster and reach better CTR. DES requires much less communication by substituting the weights-rich operators with the computationally equivalent sub-operators and aggregating partial results instead of transmitting the huge sparse weights directly through the network. Due to the use of synchronous training on large-scale Deep Learning Recommendation Models (DLRMs), DES achieves higher AUC(Area Under ROC). We successfully apply DES training on multiple popular DLRMs of industrial scenarios. Experiments show that our implementation outperforms the state-of-the-art PS-based training framework, achieving up to 68.7% communication savings and higher throughput compared to other PS-based recommender systems.
更多查看译文
关键词
recommender systems, ranking systems, dynamic sparse features, synchronous training
AI 理解论文
溯源树
样例
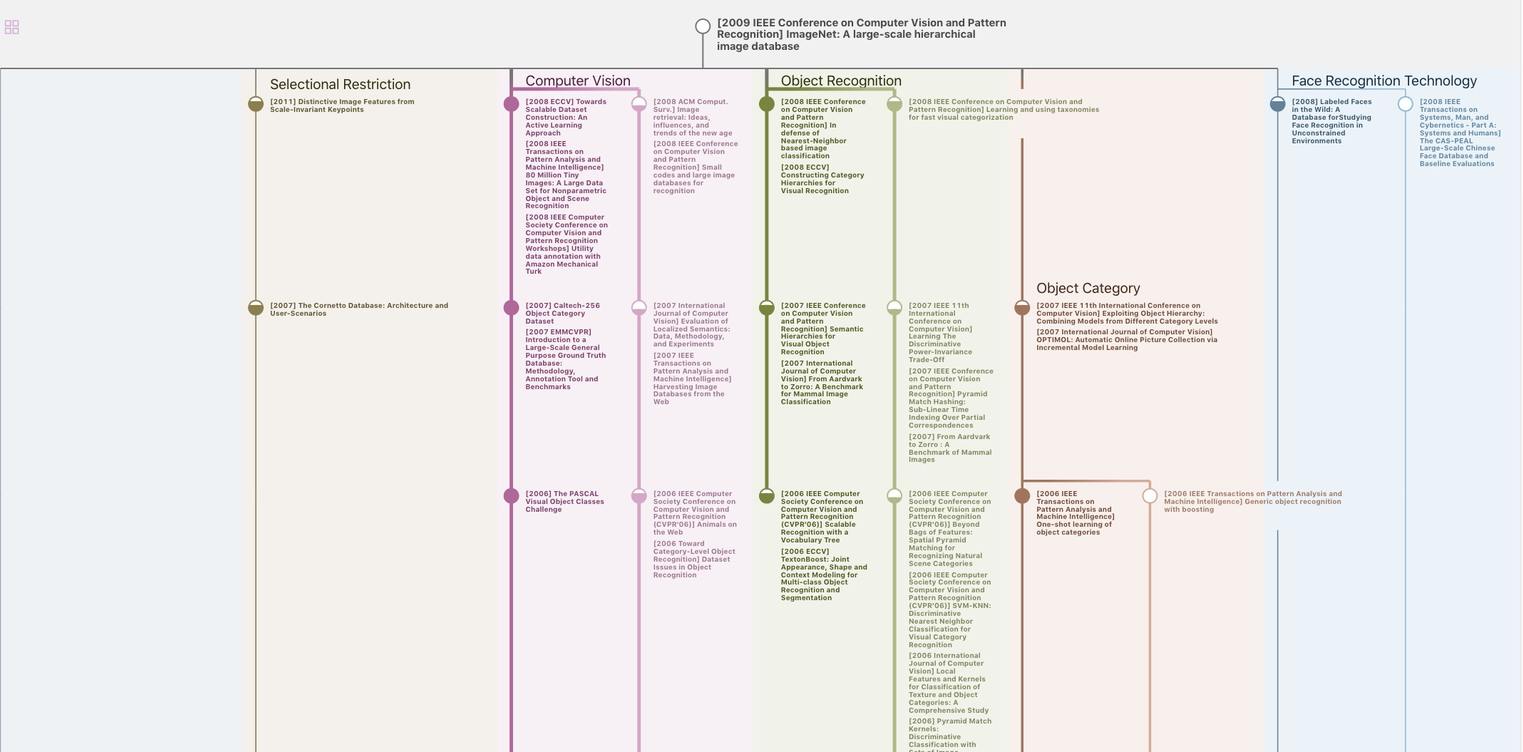
生成溯源树,研究论文发展脉络
Chat Paper
正在生成论文摘要