Integrating Cross-modality Hallucinated MRI with CT to Aid Mediastinal Lung Tumor Segmentation
MEDICAL IMAGE COMPUTING AND COMPUTER ASSISTED INTERVENTION - MICCAI 2019, PT VI(2019)
摘要
Lung tumors, especially those located close to or surrounded by soft tissues like the mediastinum, are difficult to segment due to the low soft tissue contrast on computed tomography images. Magnetic resonance images contain superior soft-tissue contrast information that can be leveraged if both modalities were available for training. Therefore, we developed a cross-modality educed learning approach where MR information that is educed from CT is used to hallucinate MRI and improve CT segmentation. Our approach, called cross-modality educed deep learning segmentation (CMEDL) combines CT and pseudo MR produced from CT by aligning their features to obtain segmentation on CT. Features computed in the last two layers of parallelly trained CT and MR segmentation networks are aligned. We implemented this approach on U-net and dense fully convolutional networks (dense-FCN). Our networks were trained on unrelated cohorts from open-source the Cancer Imaging Archive CT images (N = 377), an internal archive T2-weighted MR (N = 81), and evaluated using separate validation (N = 304) and testing (N = 333) CT-delineated tumors. Our approach using both networks were significantly more accurate (U-net \(P <0.001\); denseFCN \(P <0.001\)) than CT-only networks and achieved an accuracy (Dice similarity coefficient) of \(0.71\pm 0.15\) (U-net), \(0.74\pm 0.12\) (denseFCN) on validation and \(0.72\pm 0.14\) (U-net), \(0.73\pm 0.12\) (denseFCN) on the testing sets. Our novel approach demonstrated that educing cross-modality information through learned priors enhances CT segmentation performance.
更多查看译文
关键词
Hallucinating MRI from CT for segmentation,Lung tumors,Adversarial cross-domain deep learning
AI 理解论文
溯源树
样例
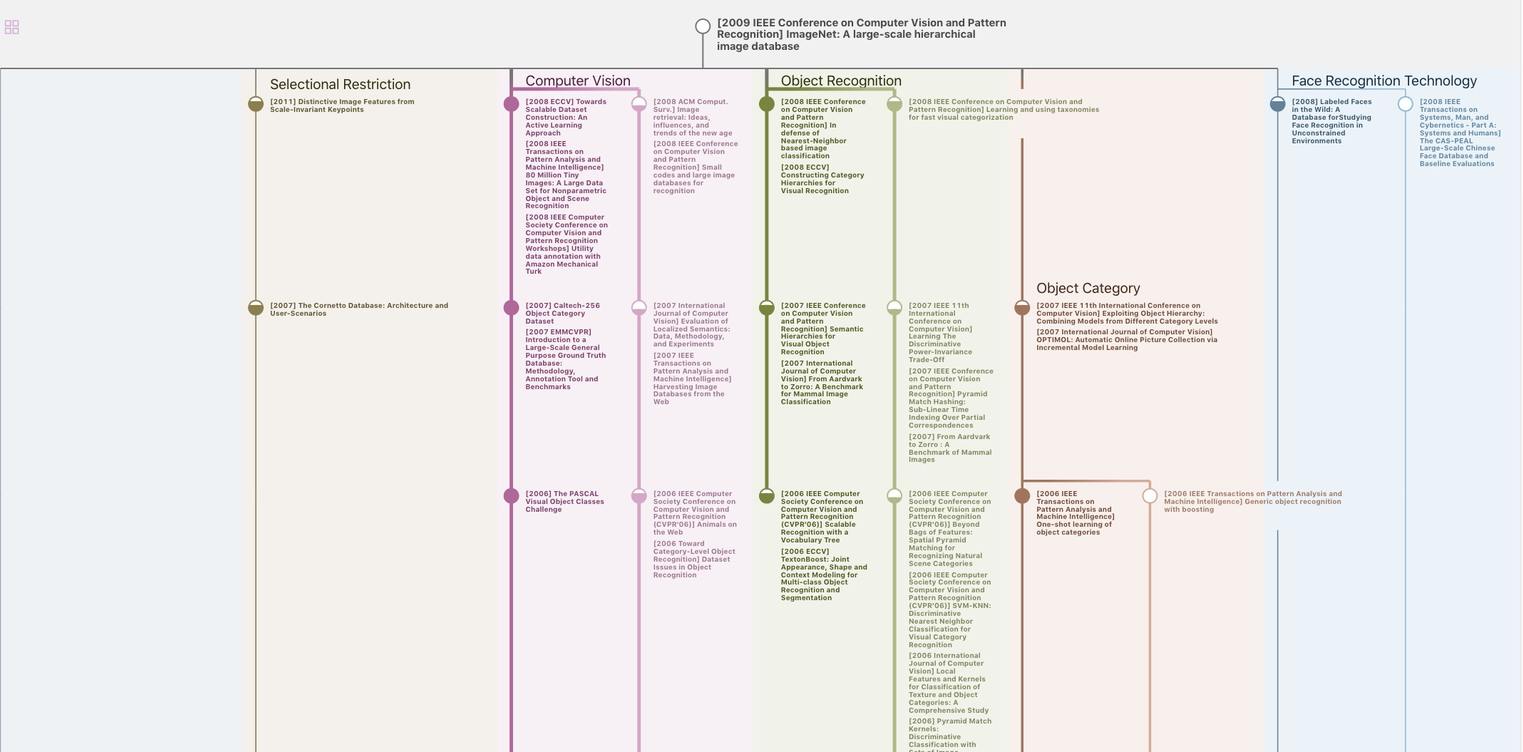
生成溯源树,研究论文发展脉络
Chat Paper
正在生成论文摘要