An Intersectional Definition Of Fairness
2020 IEEE 36TH INTERNATIONAL CONFERENCE ON DATA ENGINEERING (ICDE 2020)(2020)
摘要
We propose differential fairness, a multi-attribute definition of fairness in machine learning which is informed by intersectionality, a critical lens arising from the humanities literature, leveraging connections between differential privacy and legal notions of fairness. We show that our criterion behaves sensibly for any subset of the set of protected attributes, and we prove economic, privacy, and generalization guarantees. We provide a learning algorithm which respects our differential fairness criterion. Experiments on the COMPAS criminal recidivism dataset and census data demonstrate the utility of our methods.
更多查看译文
关键词
fairness in AI, AI and society, 80% rule, privacy
AI 理解论文
溯源树
样例
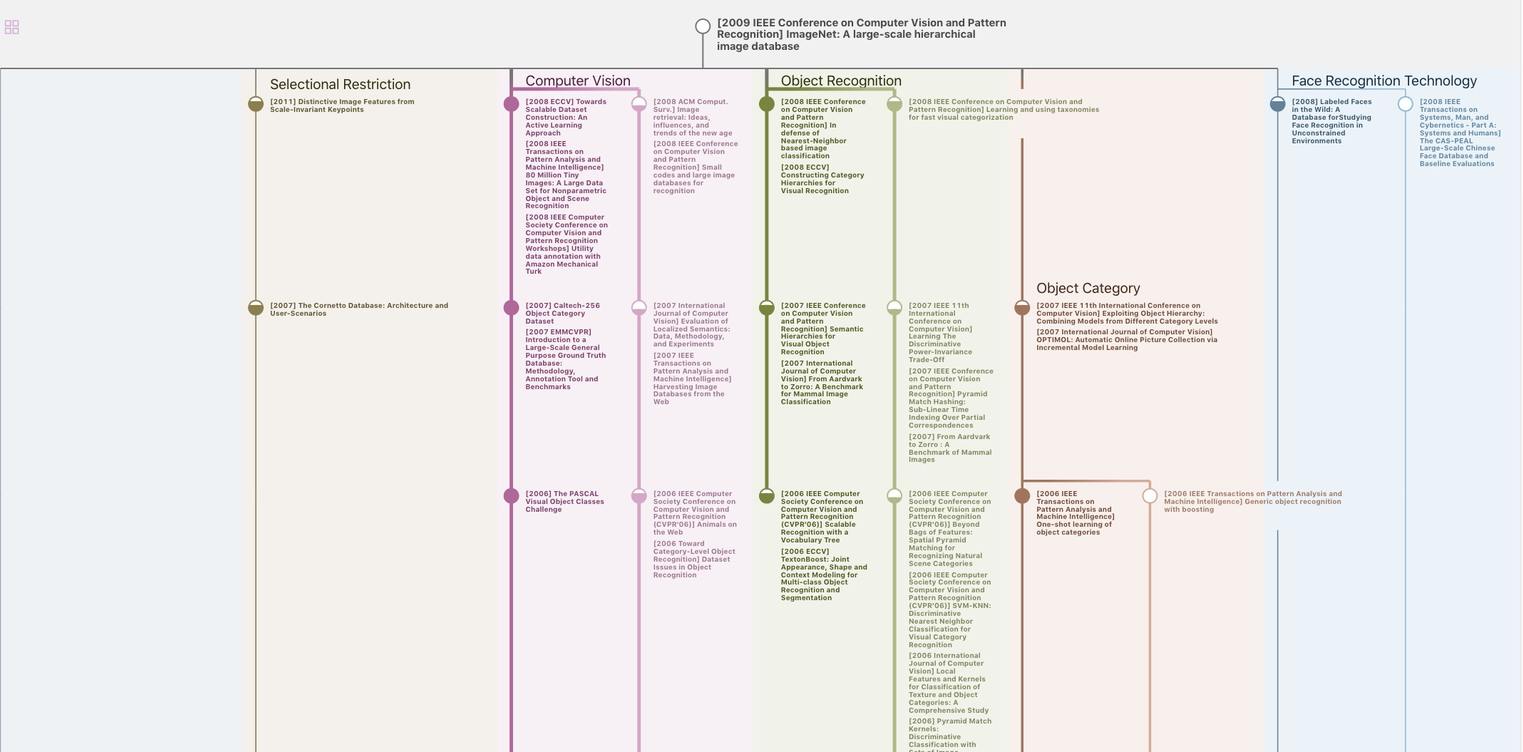
生成溯源树,研究论文发展脉络
Chat Paper
正在生成论文摘要