Homophily Preserving Community Detection
IEEE Transactions on Neural Networks and Learning Systems(2020)
摘要
As a fundamental problem in social network analysis, community detection has recently attracted wide attention, accompanied by the output of numerous community detection methods. However, most existing methods are developed by only exploiting link topology, without taking node homophily (i.e., node similarity) into consideration. Thus, much useful information that can be utilized to improve the quality of detected communities is ignored. To overcome this limitation, we propose a new community detection approach based on nonnegative matrix factorization (NMF), namely, homophily preserving NMF (HPNMF), which models not only link topology but also node homophily of networks. As such, HPNMF is able to better reflect the inherent properties of community structure. In order to capture node homophily from scratch, we provide three similarity measurements that naturally reveal the association relationships between nodes. We further present an efficient learning algorithm with convergence guarantee to solve the proposed model. Finally, extensive experiments are conducted, and the results demonstrate that HPNMF has strong ability to outperform the state-of-the-art baseline methods.
更多查看译文
关键词
Community detection,graph clustering,node homophily,nonnegative matrix factorization (NMF)
AI 理解论文
溯源树
样例
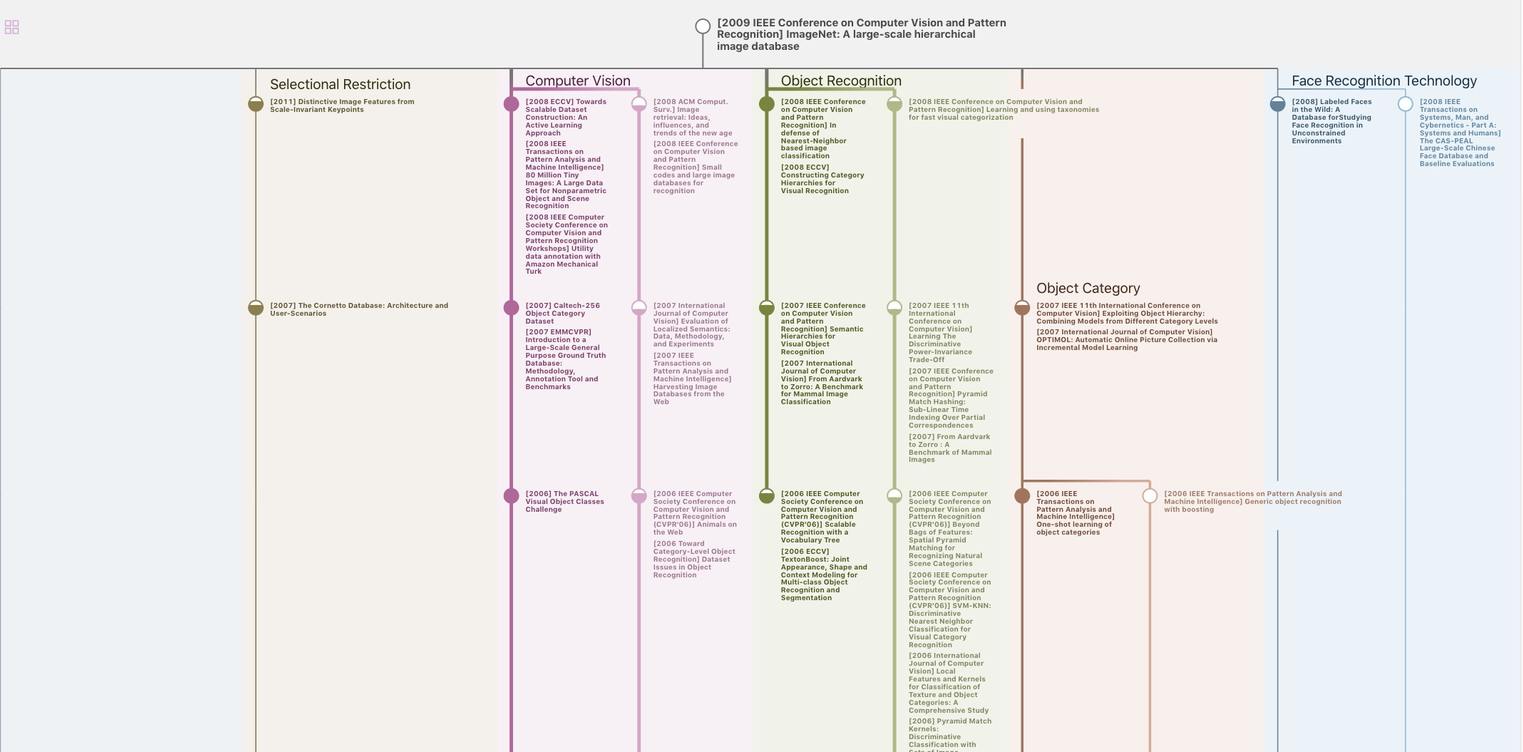
生成溯源树,研究论文发展脉络
Chat Paper
正在生成论文摘要