EEG-Based Adaptive Driver-Vehicle Interface Using Variational Autoencoder and PI-TSVM.
IEEE Transactions on Neural Systems and Rehabilitation Engineering(2019)
摘要
Event-related potential (ERP)-based driver-vehicle interfaces (DVIs) have been developed to provide a communication channel for people with disabilities to drive a vehicle. However, they require a tedious and time-consuming training procedure to build the decoding model, which can translate EEG signals into commands. In this paper, to address this problem, we propose an adaptive DVI by using a new semi-supervised algorithm. The decoding model of the proposed DVI is first built with a small labeled training set, and then gradually improved by updating the proposed semi-supervised decoding model with new collected unlabeled EEG signals. In our semi-supervised algorithm, independent component analysis (ICA) and Kalman smoother are first used to improve the signal-to-noise ratio (SNR). After that, variational autoencoder is applied to provide a robust feature representation of EEG signals. Finally, a prior information-based transductive support vector machine (PI-TSVM) classifier is developed to translate these features into commands. Experimental results show that the proposed DVI can significantly reduce the training effort. After a short updating, its performance can be close to that of the supervised DVI requiring a lengthy training procedure. This work is vital for advancing the application of these DVIs.
更多查看译文
关键词
Electroencephalography,Decoding,Brain modeling,Training,Adaptation models,Kalman filters,Integrated circuits
AI 理解论文
溯源树
样例
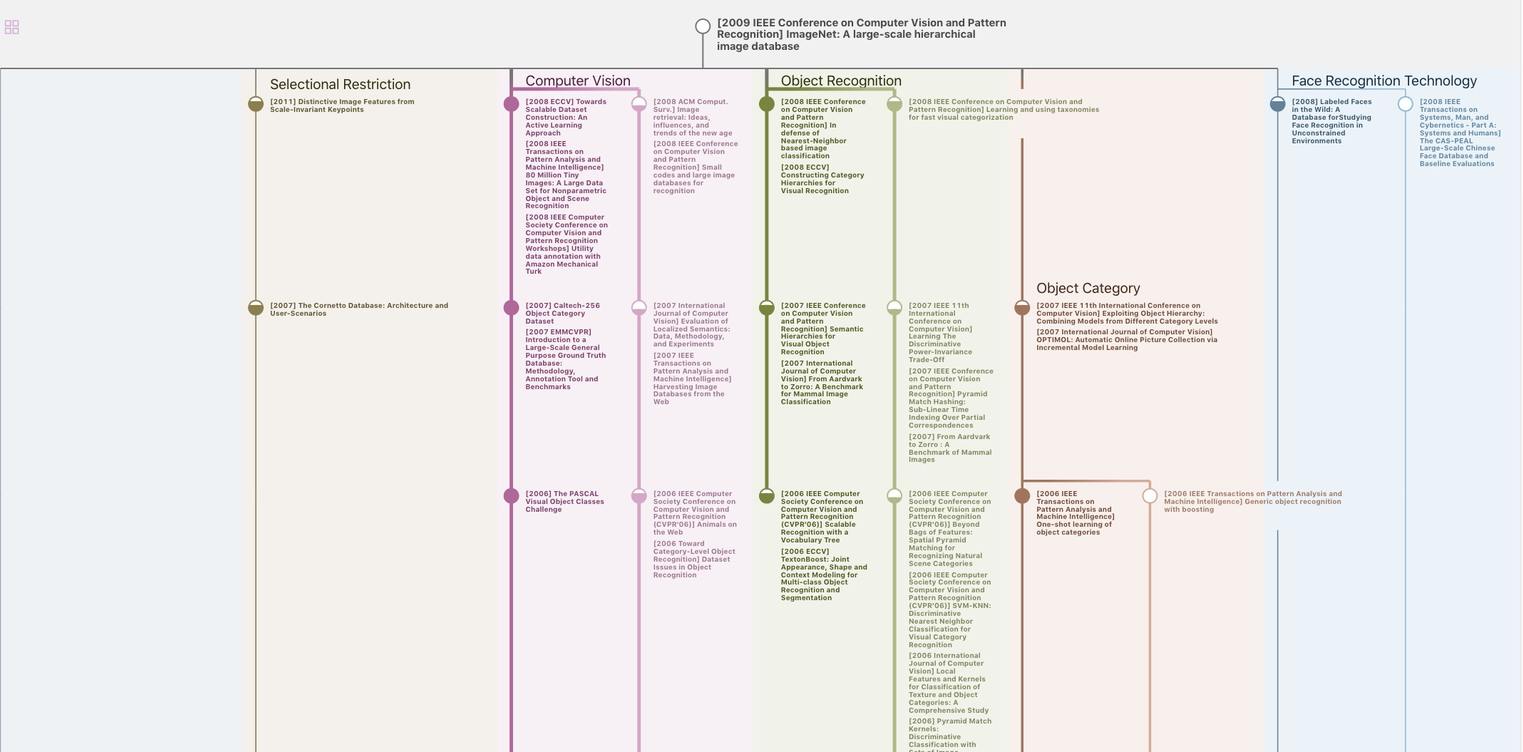
生成溯源树,研究论文发展脉络
Chat Paper
正在生成论文摘要