Leveraging Active Learning and Conditional Mutual Information to Minimize Data Annotation in Human Activity Recognition
Proceedings of the ACM on Interactive, Mobile, Wearable and Ubiquitous Technologies(2019)
摘要
A difficulty in human activity recognition (HAR) with wearable sensors is the acquisition of large amounts of annotated data for training models using supervised learning approaches. While collecting raw sensor data has been made easier with advances in mobile sensing and computing, the process of data annotation remains a time-consuming and onerous process. This paper explores active learning as a way to minimize the labor-intensive task of labeling data. We train models with active learning in both offline and online settings with data from 4 publicly available activity recognition datasets and show that it performs comparably to or better than supervised methods while using around 10% of the training data. Moreover, we introduce a method based on conditional mutual information for determining when to stop the active learning process while maximizing recognition performance. This is an important issue that arises in practice when applying active learning to unlabeled datasets.
更多查看译文
关键词
Active Learning, Conditional Mutual Information, Data Annotation, Human Activity Recognition, Stopping Criterion
AI 理解论文
溯源树
样例
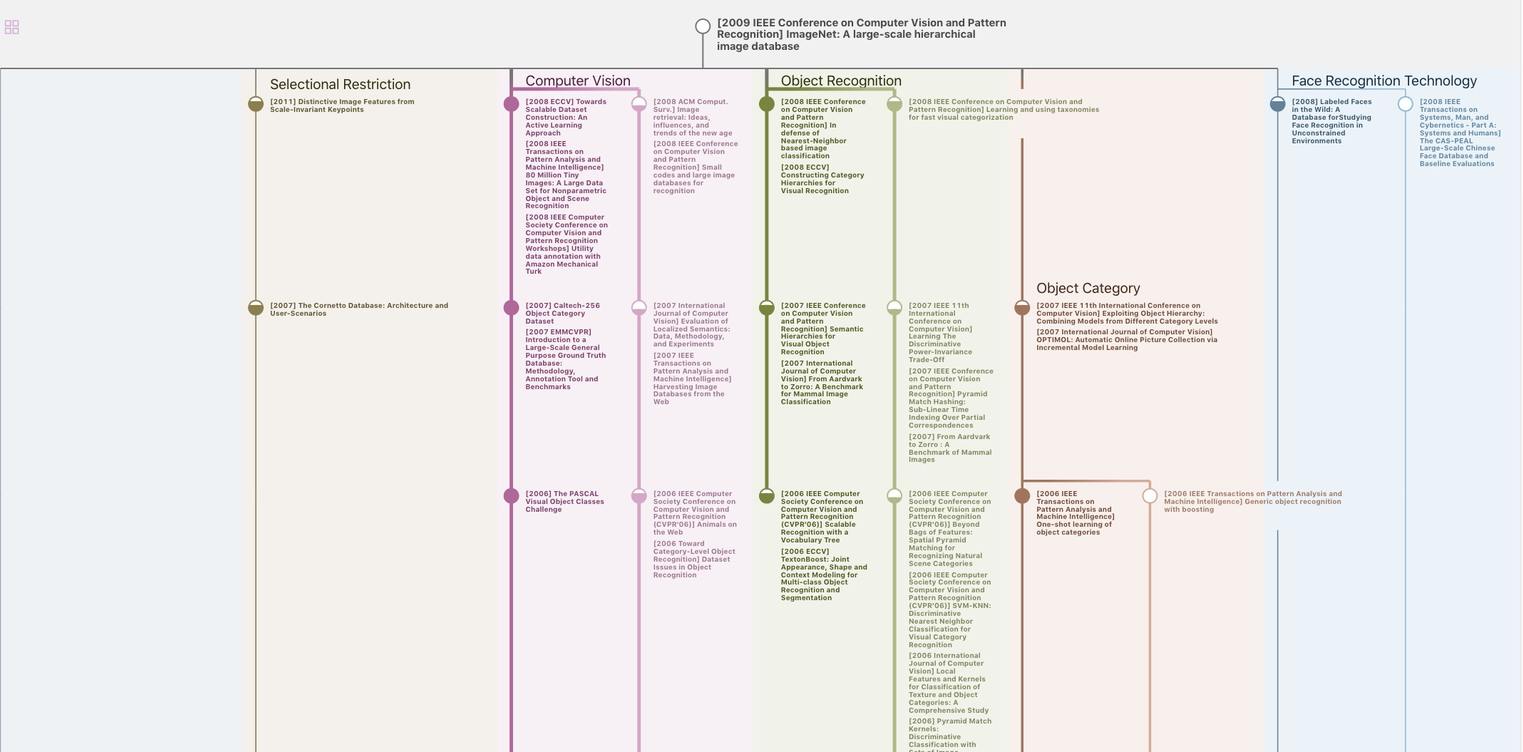
生成溯源树,研究论文发展脉络
Chat Paper
正在生成论文摘要