Belief Regulated Dual Propagation Nets for Learning Action Effects on Groups of Articulated Objects.
ICRA(2020)
摘要
In recent years, graph neural networks have been successfully applied for learning the dynamics of complex and partially observable physical systems. However, their use in the robotics domain is, to date, still limited. In this paper, we introduce Belief Regulated Dual Propagation Networks (BRDPN), a general-purpose learnable physics engine, which enables a robot to predict the effects of its actions in scenes containing groups of articulated multi-part objects. Specifically, our framework extends recently proposed propagation networks (PropNets) and consists of two complementary components, a physics predictor and a belief regulator. While the former predicts the future states of the object(s) manipulated by the robot, the latter constantly corrects the robot’s knowledge regarding the objects and their relations. Our results showed that after training in a simulator, the robot can reliably predict the consequences of its actions in object trajectory level and exploit its own interaction experience to correct its belief about the state of the environment, enabling better predictions in partially observable environments. Furthermore, the trained model was transferred to the real world and verified in predicting trajectories of pushed interacting objects whose joint relations were initially unknown. We compared BRDPN against PropNets, and showed that BRDPN performs consistently well. Moreover, BRDPN can adapt its physic predictions, since the relations can be predicted online.
更多查看译文
关键词
groups of articulated objects,learning action effects,complex robotic systems,graph neural networks,Belief Regulated Dual Propagation nets,object interaction,object trajectory level,belief regulator,physics predictor,PropNets,general-purpose learnable physics engine,BRDPN,robotics domain
AI 理解论文
溯源树
样例
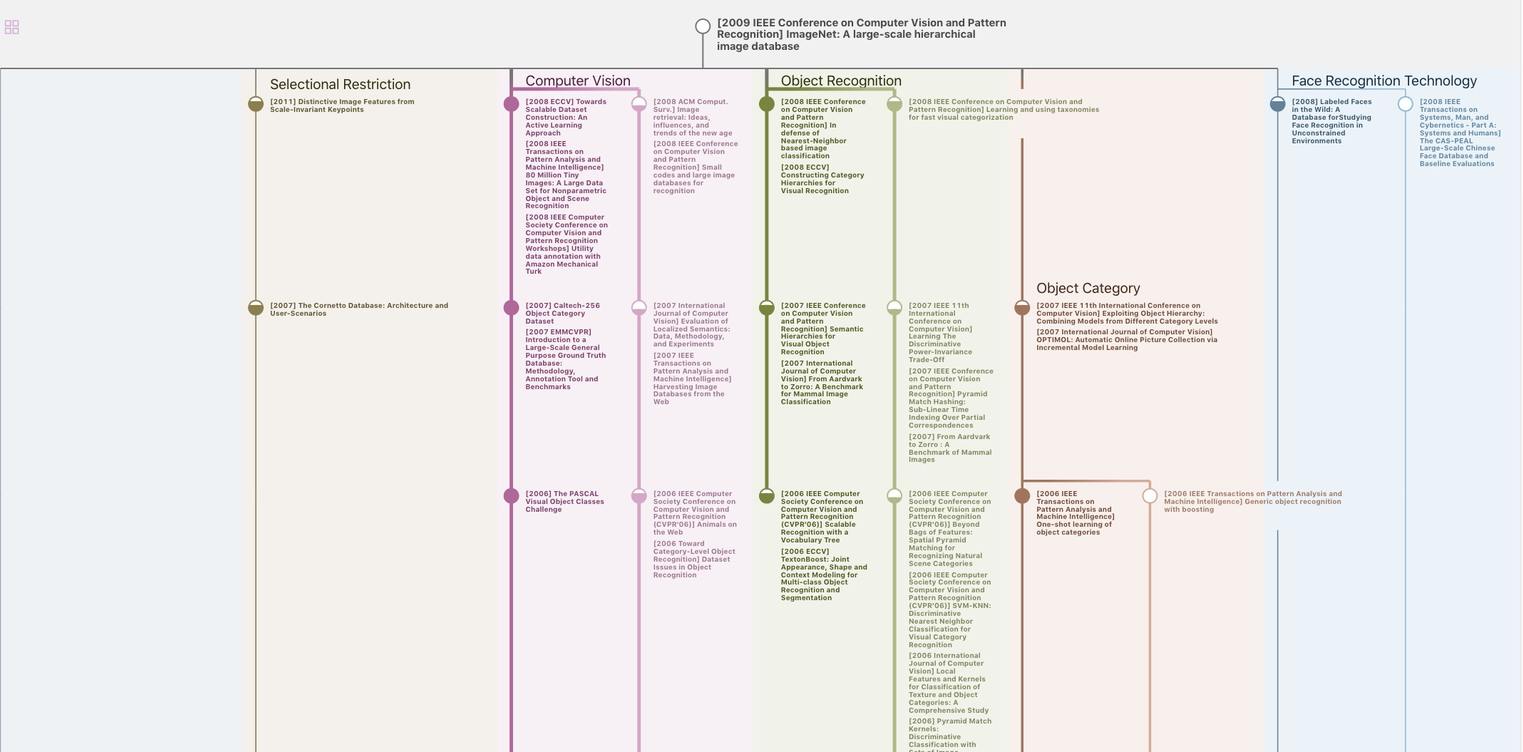
生成溯源树,研究论文发展脉络
Chat Paper
正在生成论文摘要