Dear: Deep Reinforcement Learning For Online Advertising Impression In Recommender Systems
arxiv(2021)
摘要
With the recent prevalence of Reinforcement Learning (RL), there have been tremendous interests in utilizing RL for online advertising in recommendation platforms (e.g., e-commerce and news feed sites). However, most RL-based advertising algorithms focus on optimizing ads' revenue while ignoring the possible negative influence of ads on user experience of recommended items (products, articles and videos). Developing an optimal advertising algorithm in recommendations faces immense challenges because interpolating ads improperly or too frequently may decrease user experience, while interpolating fewer ads will reduce the advertising revenue. Thus, in this paper, we propose a novel advertising strategy for the rec/ads trade-off. To be specific, we develop an RL-based framework that can continuously update its advertising strategies and maximize reward in the long run. Given a recommendation list, we design a novel Deep Q-network architecture that can determine three internally related tasks jointly, i.e., (i) whether to interpolate an ad or not in the recommendation list, and if yes, (ii) the optimal ad and (iii) the optimal location to interpolate. The experimental results based on real-world data demonstrate the effectiveness of the proposed framework.
更多查看译文
关键词
deep reinforcement learning,online advertising,recommender systems
AI 理解论文
溯源树
样例
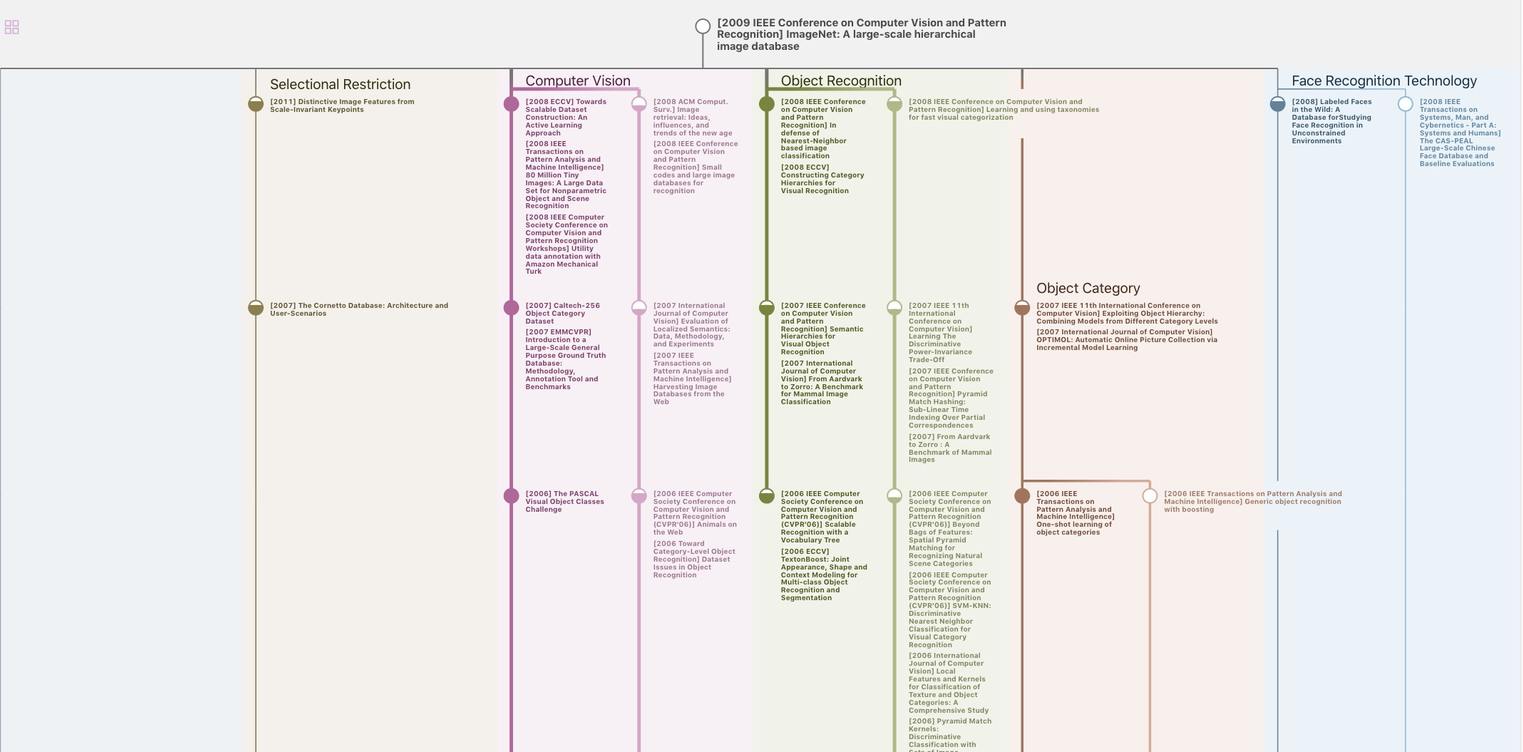
生成溯源树,研究论文发展脉络
Chat Paper
正在生成论文摘要