Coarse-to-fine salient object detection with low-rank matrix recovery
Neurocomputing(2020)
摘要
Low-rank matrix recovery (LRMR) has recently been applied to saliency detection by decomposing image features into a low-rank component associated with background and a sparse component associated with visual salient regions. Despite its great potential, existing LRMR-based saliency detection methods seldom consider the inter-relationship among elements within these two components, thus are prone to generating scattered or incomplete saliency maps. In this paper, we introduce a novel and efficient LRMR-based saliency detection model under a coarse-to-fine framework to circumvent this limitation. First, we roughly measure the saliency of image regions with a baseline LRMR model that integrates a ℓ1-norm sparsity constraint and a Laplacian regularization smooth term. Given samples from the coarse saliency map, we then learn a projection that maps image features to refined saliency values, to significantly sharpen the object boundaries and to preserve the object entirety. We evaluate our framework against existing LRMR-based methods on three benchmark datasets. Experimental results validate the superiority of our method as well as the effectiveness of our suggested coarse-to-fine framework, especially for images containing multiple objects. MATLAB code for implementing our method is available at https://github.com/qizhust/HLRSaliency.
更多查看译文
关键词
Salient object detection,Coarse-to-fine,Low-rank matrix recovery,Learning-based refinement
AI 理解论文
溯源树
样例
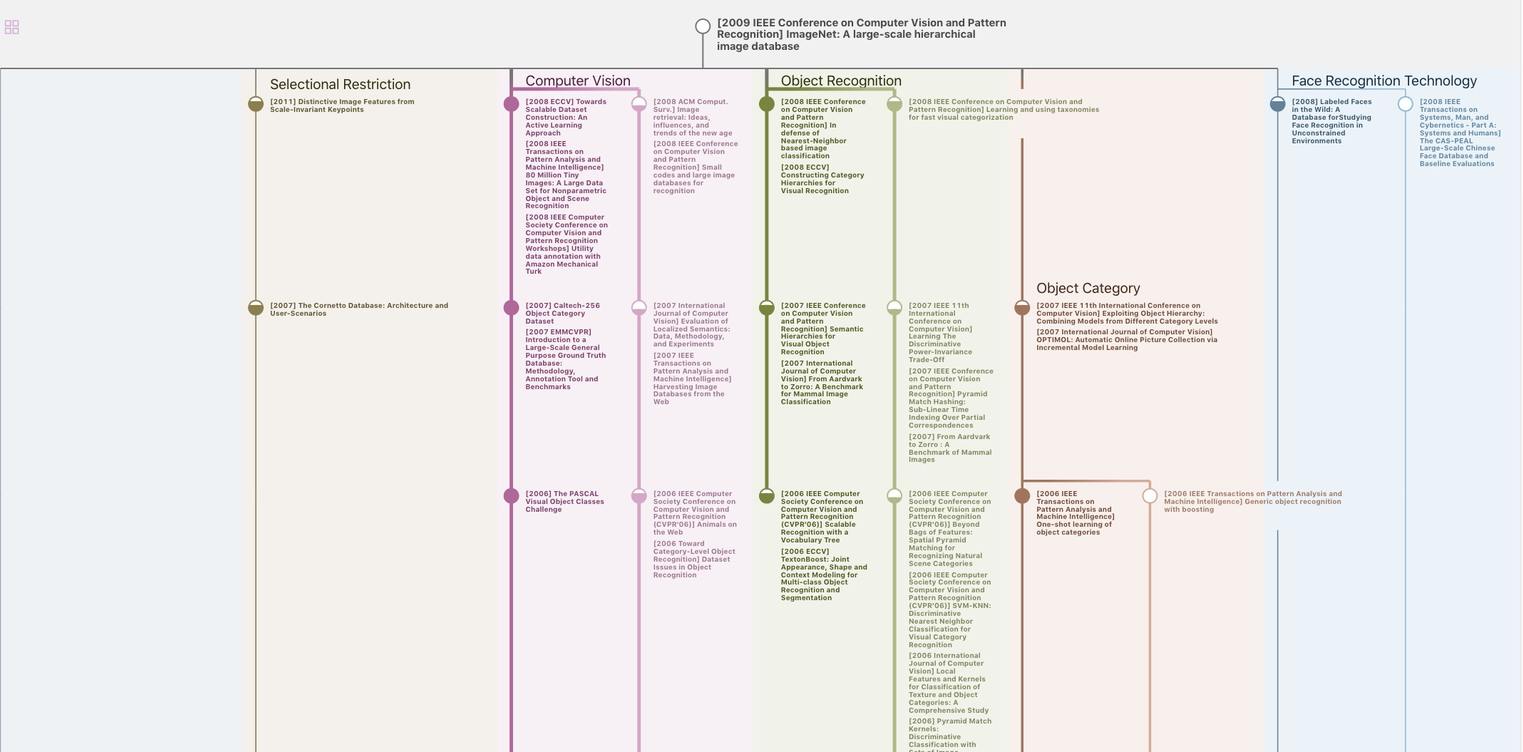
生成溯源树,研究论文发展脉络
Chat Paper
正在生成论文摘要