Parallel Computation of Graph Embeddings
arxiv(2019)
摘要
Graph embedding aims at learning a vector-based representation of vertices that incorporates the structure of the graph. This representation then enables inference of graph properties. Existing graph embedding techniques, however, do not scale well to large graphs. We therefore propose a framework for parallel computation of a graph embedding using a cluster of compute nodes with resource constraints. We show how to distribute any existing embedding technique by first splitting a graph for any given set of constrained compute nodes and then reconciling the embedding spaces derived for these subgraphs. We also propose a new way to evaluate the quality of graph embeddings that is independent of a specific inference task. Based thereon, we give a formal bound on the difference between the embeddings derived by centralised and parallel computation. Experimental results illustrate that our approach for parallel computation scales well, while largely maintaining the embedding quality.
更多查看译文
关键词
graph embeddings,parallel computation
AI 理解论文
溯源树
样例
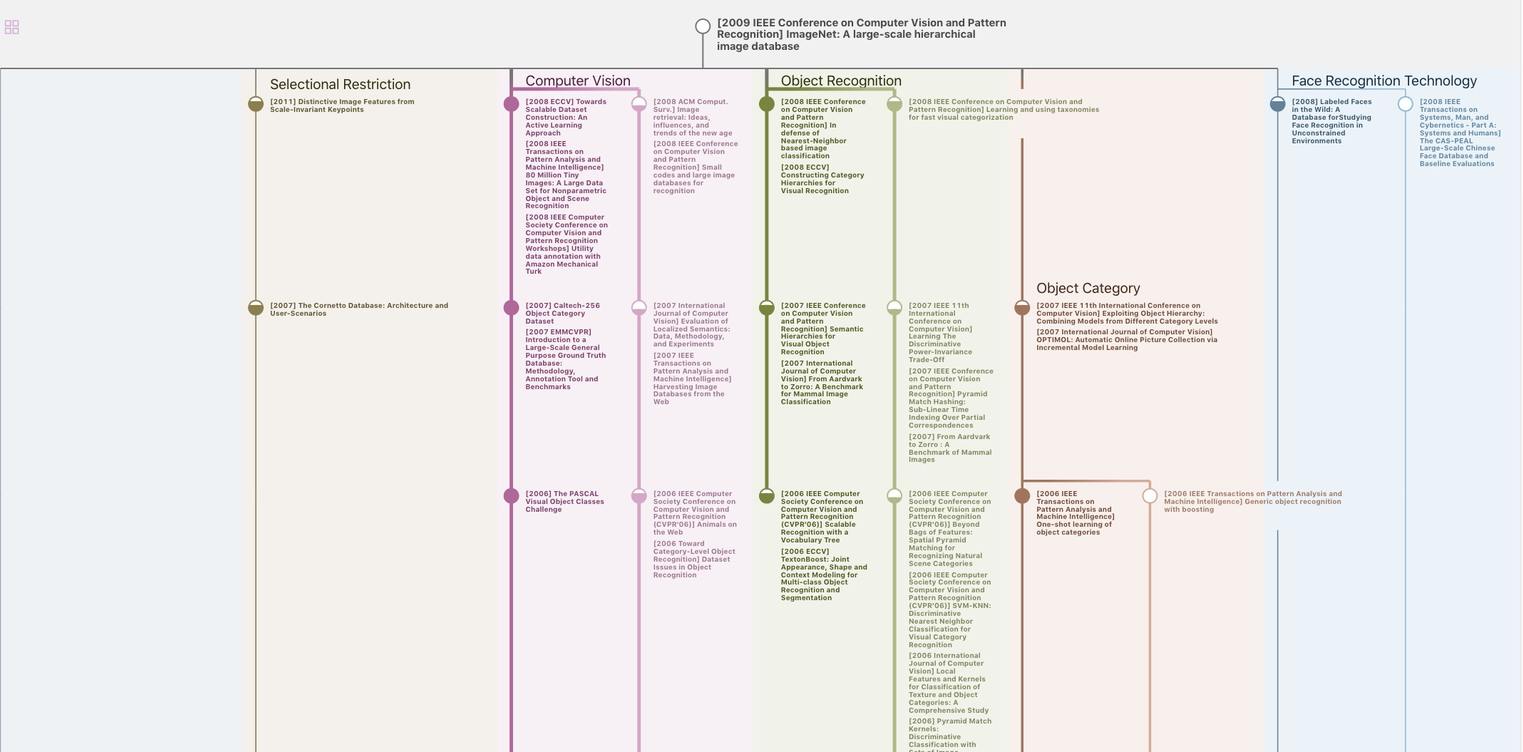
生成溯源树,研究论文发展脉络
Chat Paper
正在生成论文摘要