A Fleet-Based Machine Learning Approach for Automatic Detection of Deviations between Measurements and Reality
2019 30TH IEEE INTELLIGENT VEHICLES SYMPOSIUM (IV19)(2019)
摘要
Deviations between system current measurements and reality can cause severe problems in the power train of electric vehicles (EVs). Among others, these are inaccurate performance coordination and unnecessary power limitations during driving or charging. In this work, we propose a fleet-based framework to detect such deviations. Our main assumption is that the real value is the mean of all identically constructed EVs' measurements for the same input. Under this assumption, we train individual on-board models to predict the current of the electric machine (EM) and transmit the model parameters to a back-end. There, we compare individual deviations of the predicted current against the fleet in the same scenario. We use the results to classify three fault sources. As models we choose two different Machine Learning algorithms: State Models and Long Short-Term Memory Neural Networks (LSTMs). These are evaluated on an artificial fleet of 34 EVs derived from real drive data containing three different kinds of faults. Results show that our proposed approach correctly classifies major measurement faults. Additionally, both models offer similar classifications. LSTMs are more accurate, whereas state models are less computationally complex, and thus better suited for electronic control units (ECUs),
更多查看译文
关键词
electric machine,fault sources,state models,artificial fleet,drive data,measurement faults,automatic detection,power train,electric vehicles,fleet-based framework,long short-term memory neural networks,fleet-based machine learning approach,EVs measurements,machine learning algorithms,classifications
AI 理解论文
溯源树
样例
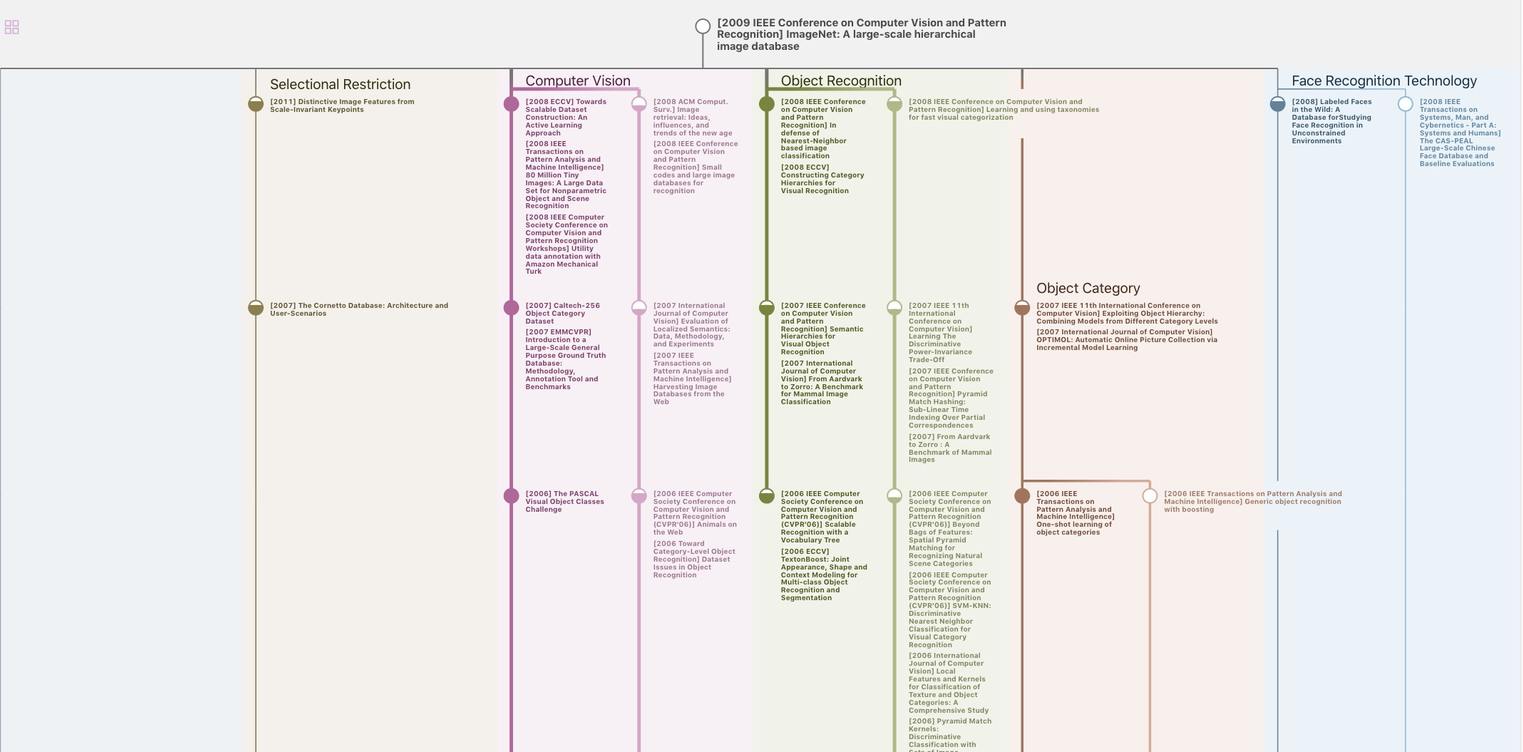
生成溯源树,研究论文发展脉络
Chat Paper
正在生成论文摘要