DMFD: Non-Intrusive Dependency Inference and Flow Ratio Model for Performance Anomaly Detection in Multi-Tier Cloud Applications
2019 IEEE 12th International Conference on Cloud Computing (CLOUD)(2019)
摘要
More and more complex applications are deployed in virtualized Cloud environments. Cloud applications usually consist of multiple virtual components and the data flow among these components tends to be highly complex and unpredictable. Cloud providers have no visibility into tenants' virtual components and rely on non-intrusive knowledge to infer the service performance. The scale, complexity, and heterogeneity make Cloud environments prone to different kinds of performance anomalies. An automatic anomaly detection mechanism is necessary to help avoid service failure at an early stage of anomalies. We propose DMFD, a decentralized and non-intrusive anomaly detection system for Cloud applications. DMFD considers each virtual component as a black box, and works without knowledge from underlying operating system or applications. DMFD infers the dependency model to characterize the complex interaction behavior among multiple components, and further builds an adaptive data flow ratio model for anomaly detection. The data flow model explores a simple and common relationship that the number of outgoing response data packets changes accordingly with the number of incoming request data packets at each component. We evaluate DMFD with several different kinds of Cloud applications in our data center. The results reveal that DMFD has high precision and low false positive/negative in detecting different kinds of performance anomalies under varying workload scenarios.
更多查看译文
关键词
Anomaly Detection, Dependency Model, Multi-tier Cloud Applications, Flow Ratio Model
AI 理解论文
溯源树
样例
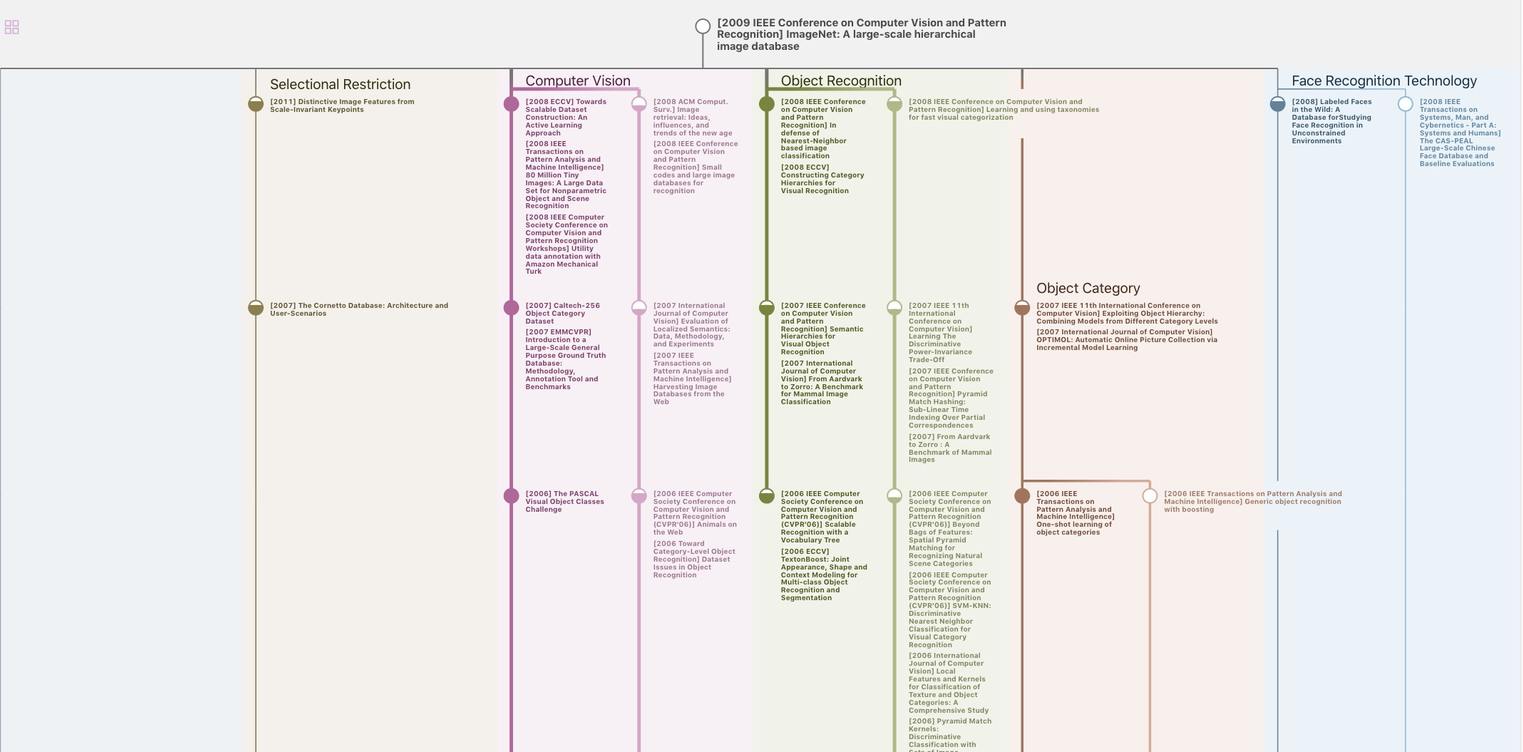
生成溯源树,研究论文发展脉络
Chat Paper
正在生成论文摘要