Neural Rule Grounding for Low-Resource Relation Extraction
arxiv(2019)
摘要
While deep neural models have gained successes on information extraction tasks, they become less reliable when the amount of labeled data is limited. In this paper, we study relation extraction (RE) under low-resource setting, where only some (hand-built) labeling rules are provided for learning a neural model over a large, unlabeled corpus. To overcome the low-coverage issue of current bootstrapping methods (i.e., hard grounding of rules), we propose a Neural Rule Grounding (REGD) framework for jointly learning a relation extraction module (with flexible neural architecture) and a sentence-rule soft matching module. The soft matching module extends the coverage of rules on semantically similar instances and augments the learning on unlabeled corpus. Experiments on two public datasets demonstrate the effectiveness of REGD when compared with both rule-based and semi-supervised baselines. Additionally, the learned soft matching module is able to predict on new relations with unseen rules, and can provide interpretation on matching results.
更多查看译文
关键词
neural rule grounding,relation,extraction,low-resource
AI 理解论文
溯源树
样例
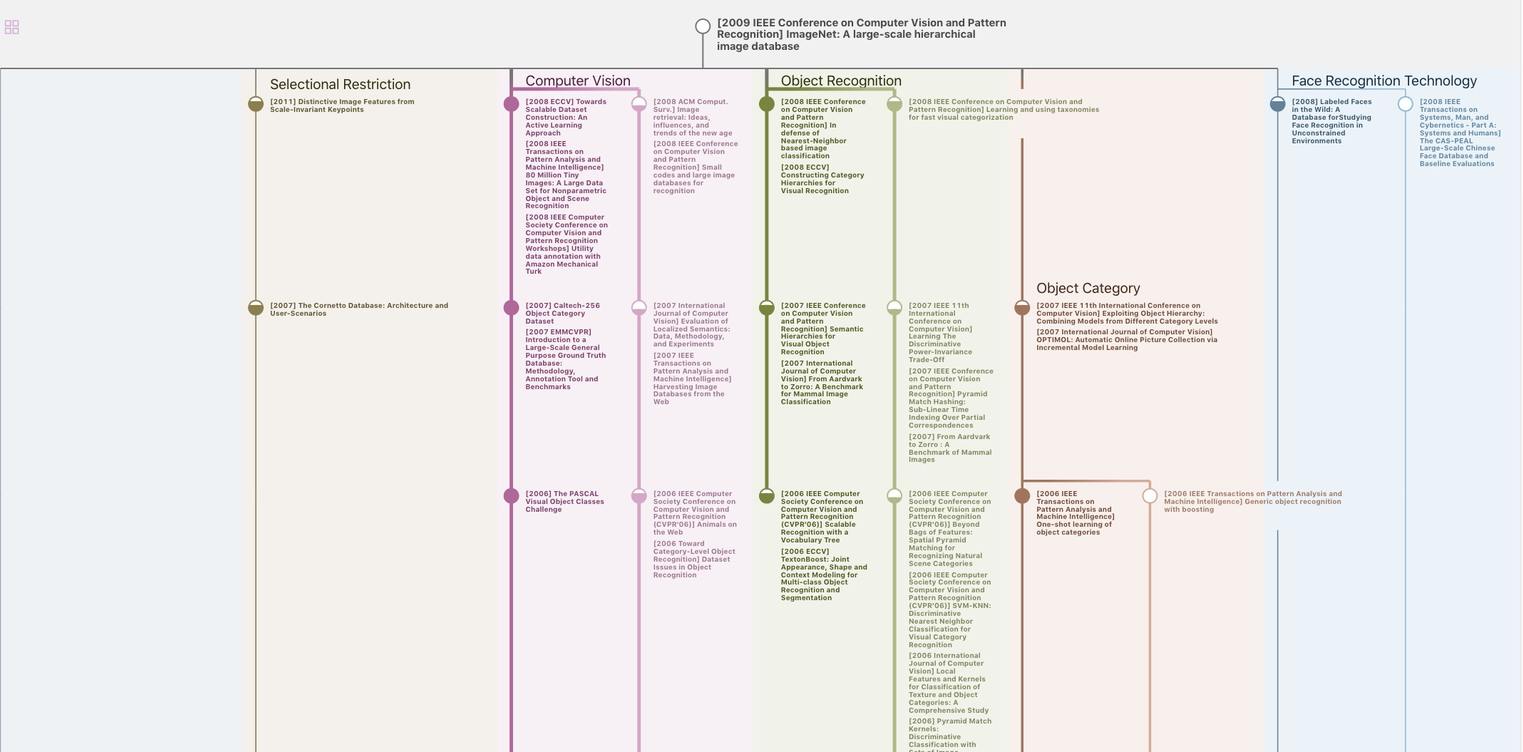
生成溯源树,研究论文发展脉络
Chat Paper
正在生成论文摘要