A Novel Hyperspectral Endmember Extraction Algorithm Based on Online Robust Dictionary Learning.
REMOTE SENSING(2019)
摘要
Due to the sparsity of hyperspectral images, the dictionary learning framework has been applied in hyperspectral endmember extraction. However, current endmember extraction methods based on dictionary learning are not robust enough in noisy environments. To solve this problem, this paper proposes a novel endmember extraction approach based on online robust dictionary learning, termed EEORDL. Because of the large scale of the hyperspectral image (HSI) data, an online scheme is introduced to reduce the computational time of dictionary learning. In the proposed algorithm, a new form of the objective function is introduced into the dictionary learning process to improve the robustness for noisy HSI data. The experimental results, conducted with both synthetic and real-world hyperspectral datasets, illustrate that the proposed EEORDL outperforms the state-of-the-art approaches under different signal-to-noise ratio (SNR) conditions, especially for high-level noise.
更多查看译文
关键词
online dictionary learning,robust functions,endmember extraction,spectral unmixing,hyperspectral images (HSI)
AI 理解论文
溯源树
样例
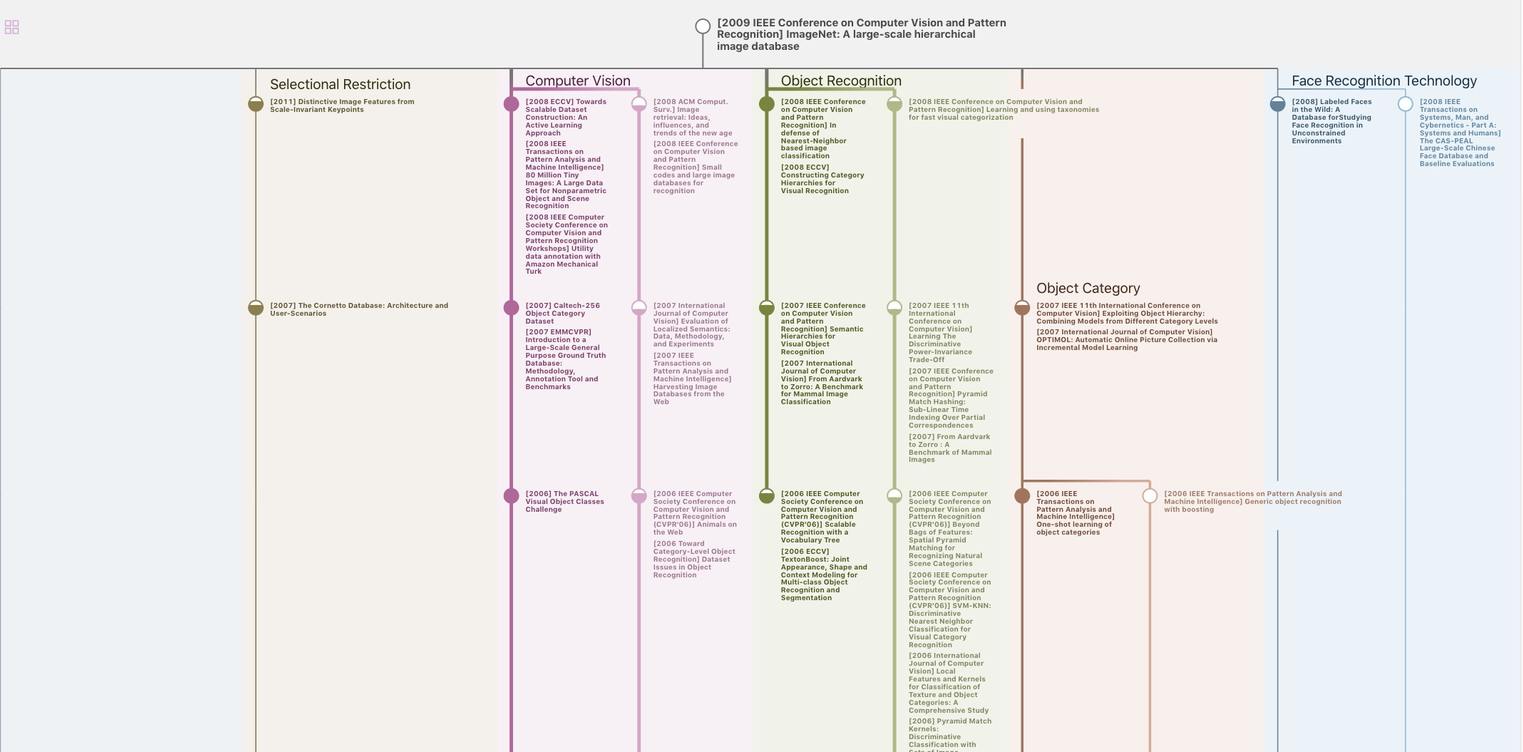
生成溯源树,研究论文发展脉络
Chat Paper
正在生成论文摘要