Extending the Scope of Robust Quadratic Optimization
INFORMS JOURNAL ON COMPUTING(2022)
摘要
We derive computationally tractable formulations of the robust counterparts of convex quadratic and conic quadratic constraints that are concave in matrix-valued uncertain parameters. We do this for a broad range of uncertainty sets. Our results provide extensions to known results from the literature. We also consider hard quadratic constraints: those that are convex in uncertain matrix-valued parameters. For the robust counterpart of such constraints, we derive inner and outer tractable approximations. As an application, we show how to construct a natural uncertainty set based on a statistical confidence set around a sample mean vector and covariance matrix and use this to provide a tractable reformulation of the robust counterpart of an uncertain portfolio optimization problem. We also apply the results of this paper to norm approximation problems. Summary of Contribution: This paper develops new theoretical results and algorithms that extend the scope of a robust quadratic optimization problem. More specifically, we derive computationally tractable formulations of the robust counterparts of convex quadratic and conic quadratic constraints that are concave in matrix-valued uncertain parameters. We also consider hard quadratic constraints: those that are convex in uncertain matrix-valued parameters. For the robust counterpart of such constraints, we derive inner and outer tractable approximations.
更多查看译文
关键词
robust optimization, quadratic optimization, inner approximation, outer approximation, mean-variance uncertainty
AI 理解论文
溯源树
样例
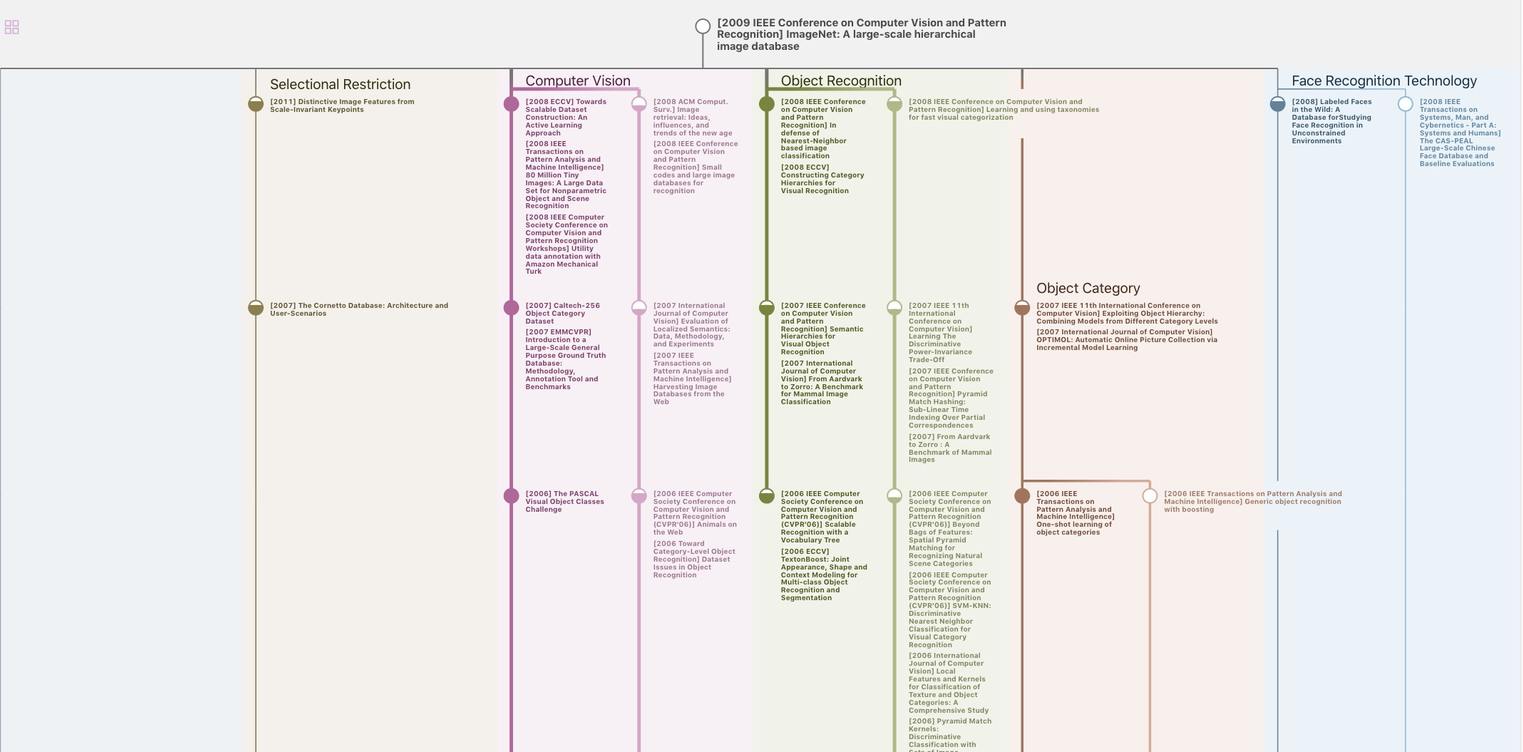
生成溯源树,研究论文发展脉络
Chat Paper
正在生成论文摘要