Incidental Supervision from Question-Answering Signals
arxiv(2019)
摘要
Human annotations are costly for many natural language processing (NLP) tasks, especially for those requiring NLP expertise. One promising solution is to use natural language to annotate natural language. However, it remains an open problem how to get supervision signals or learn representations from natural language annotations. This paper studies the case where the annotations are in the format of question-answering (QA) and proposes an effective way to learn useful representations for other tasks. We also find that the representation retrieved from question-answer meaning representation (QAMR) data can almost universally improve on a wide range of tasks, suggesting that such kind of natural language annotations indeed provide unique information on top of modern language models.
更多查看译文
AI 理解论文
溯源树
样例
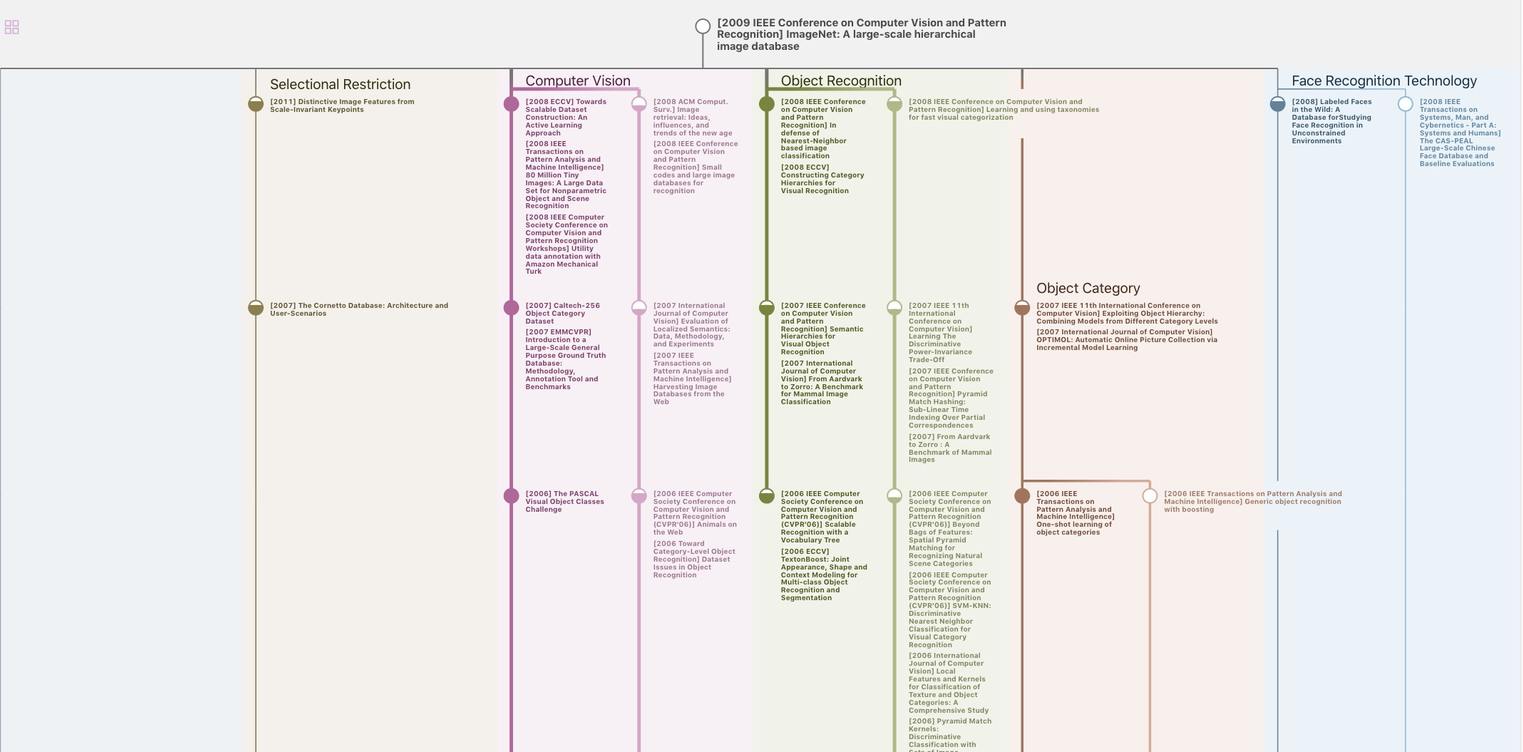
生成溯源树,研究论文发展脉络
Chat Paper
正在生成论文摘要