Warm Dense Matter Simulation Via Electron Temperature Dependent Deep Potential Molecular Dynamics
Physics of Plasmas(2020)
摘要
Simulating warm dense matter that undergoes a wide range of temperatures and densities is challenging. Predictive theoretical models, such as quantum-mechanics-based first-principles molecular dynamics (FPMD), require a huge amount of computational resources. Herein, we propose a deep learning based scheme called electron temperature dependent deep potential molecular dynamics (TDDPMD), which can be readily applied to study larger systems with longer trajectories, yielding more accurate properties. We take warm dense beryllium (Be) as an example with the training data from FPMD simulations spanning a wide range of temperatures (0.4-2500eV) and densities (3.50-8.25g/cm(3)). The TDDPMD method well reproduces the principal Hugoniot curve and radial distribution functions from the FPMD method. Furthermore, it depicts the reflection point of the Hugoniot curve more smoothly and provides more converged diffusion coefficients. We also show the new model can yield static structure factors and dynamic structure factors of warm dense Be.
更多查看译文
关键词
warm dense matter simulation,electron temperature,molecular
AI 理解论文
溯源树
样例
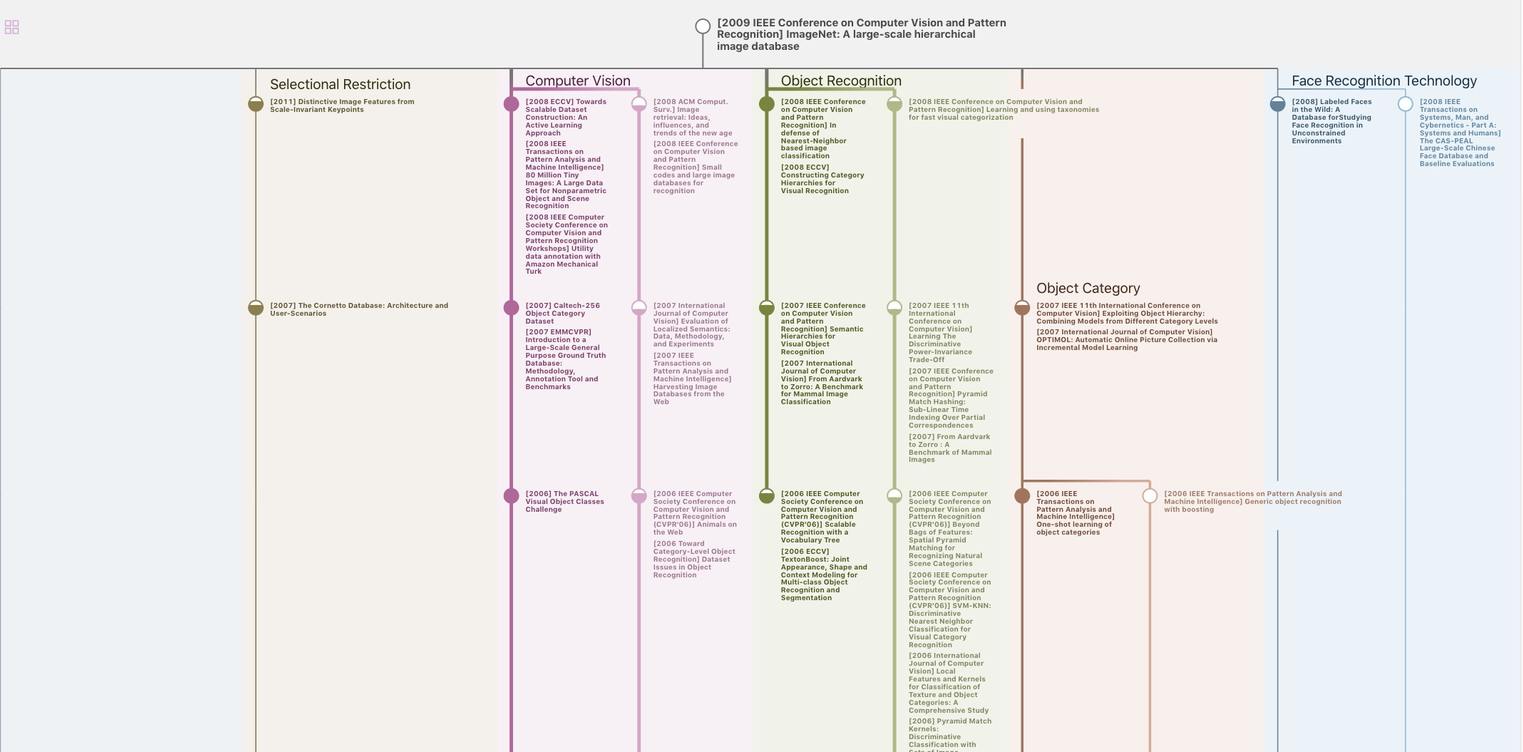
生成溯源树,研究论文发展脉络
Chat Paper
正在生成论文摘要