Multilevel Composite Stochastic Optimization Via Nested Variance Reduction
SIAM JOURNAL ON OPTIMIZATION(2021)
摘要
We consider multilevel composite optimization problems where each mapping in the composition is the expectation over a family of randomly chosen smooth mappings or the sum of some finite number of smooth mappings. We present a normalized proximal approximate gradient method where the approximate gradients are obtained via nested stochastic variance reduction. In order to find an approximate stationary point where the expected norm of its gradient mapping is less than epsilon, the total sample complexity of our method is O(epsilon(-3)) in the expectation case and O(N + root N epsilon(-2)) in the finite-sum case where N is the total number of functions across all composition levels. In addition, the dependence of our total sample complexity on the number of composition levels is polynomial, rather than exponential as in previous work.
更多查看译文
关键词
composite stochastic optimization, proximal gradient method, variance reduction
AI 理解论文
溯源树
样例
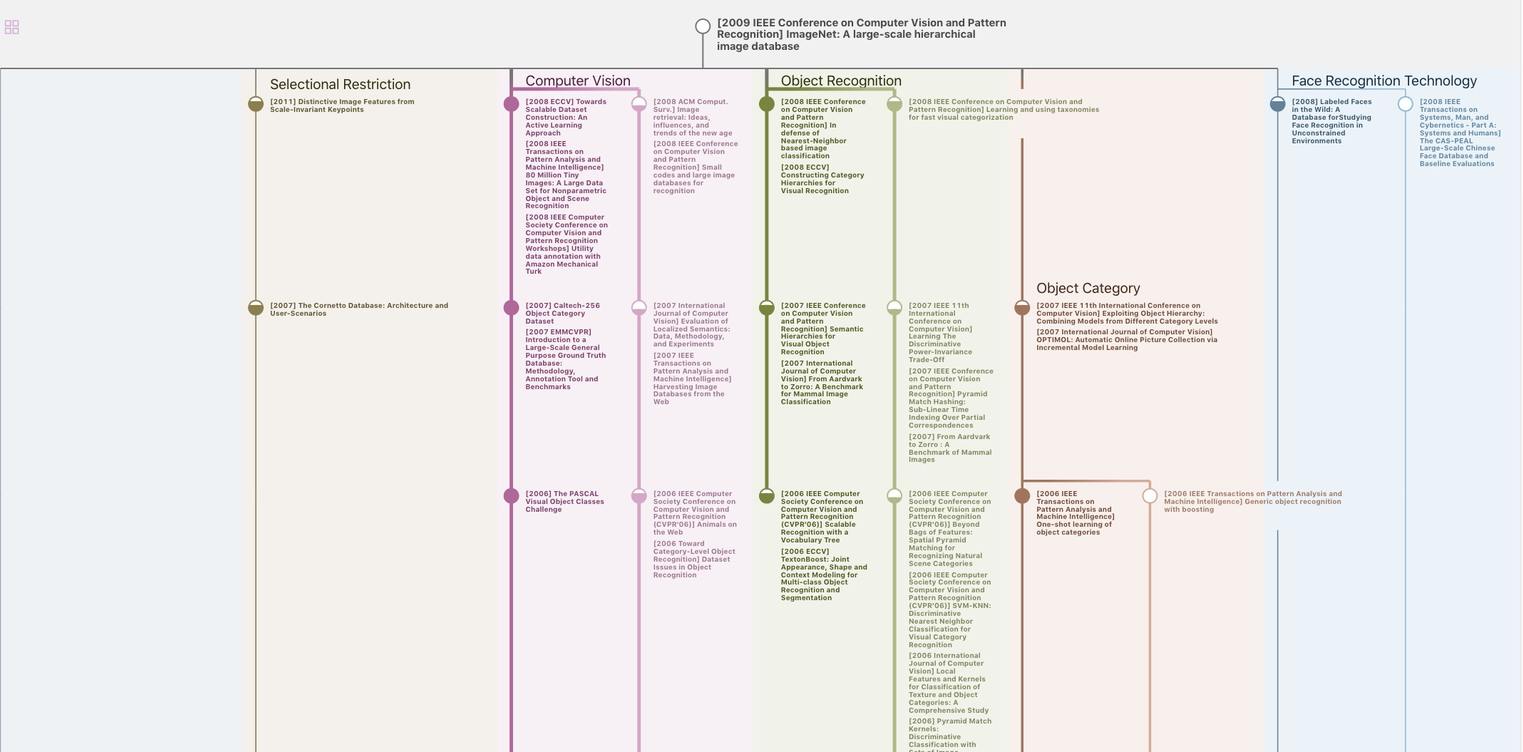
生成溯源树,研究论文发展脉络
Chat Paper
正在生成论文摘要