Beyond Error Propagation: Language Branching Also Affects the Accuracy of Sequence Generation
IEEE/ACM Transactions on Audio, Speech, and Language Processing(2019)
摘要
Sequence generation tasks, such as neural machine translation (NMT) and abstractive summarization, usually suffer from exposure bias as well as the error propagation problem due to the autoregressive training and generation. Many previous works have discussed the relationship between error propagation and the
accuracy drop
problem (i.e., the right part of the generated sentence is often worse than its left part in left-to-right decoding models). In this paper, taking NMT as a typical sequence generation task, we measure the accuracy of the generated sentence with various metrics and conduct a series of analyses to deeply understand the accuracy drop problem. We obtain several interesting findings. First, The role of error propagation on accuracy drop is overstated in the literature, although it is indeed a cause to the accuracy drop problem. Second, Characteristics of a language play a more important role in causing the accuracy drop problem: the left part of the generated sentence in a right-branching language (e.g., English) is more likely to be more accurate than its right part, while the right part is more accurate for a left-branching language (e.g., Japanese). Our discoveries are also confirmed on other generation tasks (e.g., image captioning, abstractive summarization and language modeling) with multiple left/right-branching languages, as well as in various model structures.
更多查看译文
关键词
Task analysis,Decoding,Training,Measurement,Linguistics,Speech processing,Maximum likelihood estimation
AI 理解论文
溯源树
样例
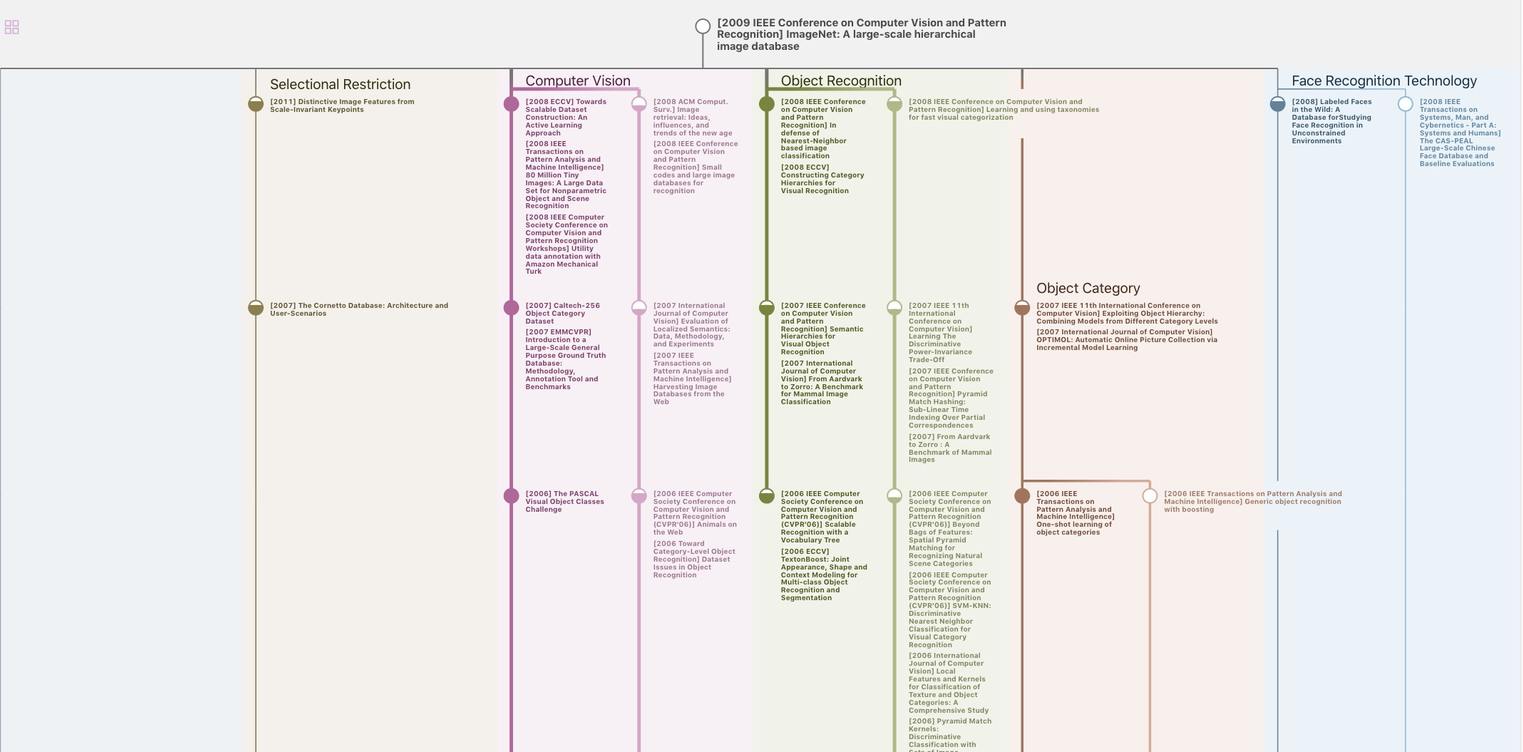
生成溯源树,研究论文发展脉络
Chat Paper
正在生成论文摘要