Explaining Entity Resolution Predictions: Where are we and What needs to be done?
Proceedings of the Workshop on Human-In-the-Loop Data Analytics(2019)
摘要
Entity resolution (ER) seeks to identify the set of tuples in a dataset that refer to the same real-world entity. It is one of the fundamental and well studied problems in data integration with applications in diverse domains such as banking, insurance, e-commerce, and so on. Machine Learning and Deep Learning based methods provide the state-of-the-art results. For practitioners, it is often challenging to understand why the classifier made a particular prediction. While there has been extensive work in the ML community on explaining classifier predictions, we found that a direct application of those techniques is not appropriate for ER. There is a huge gap between the needs of lay ER practitioners and the explanation community. In this paper, we provide a comprehensive taxonomy of these challenges, discuss research opportunities and propose preliminary solutions.
更多查看译文
AI 理解论文
溯源树
样例
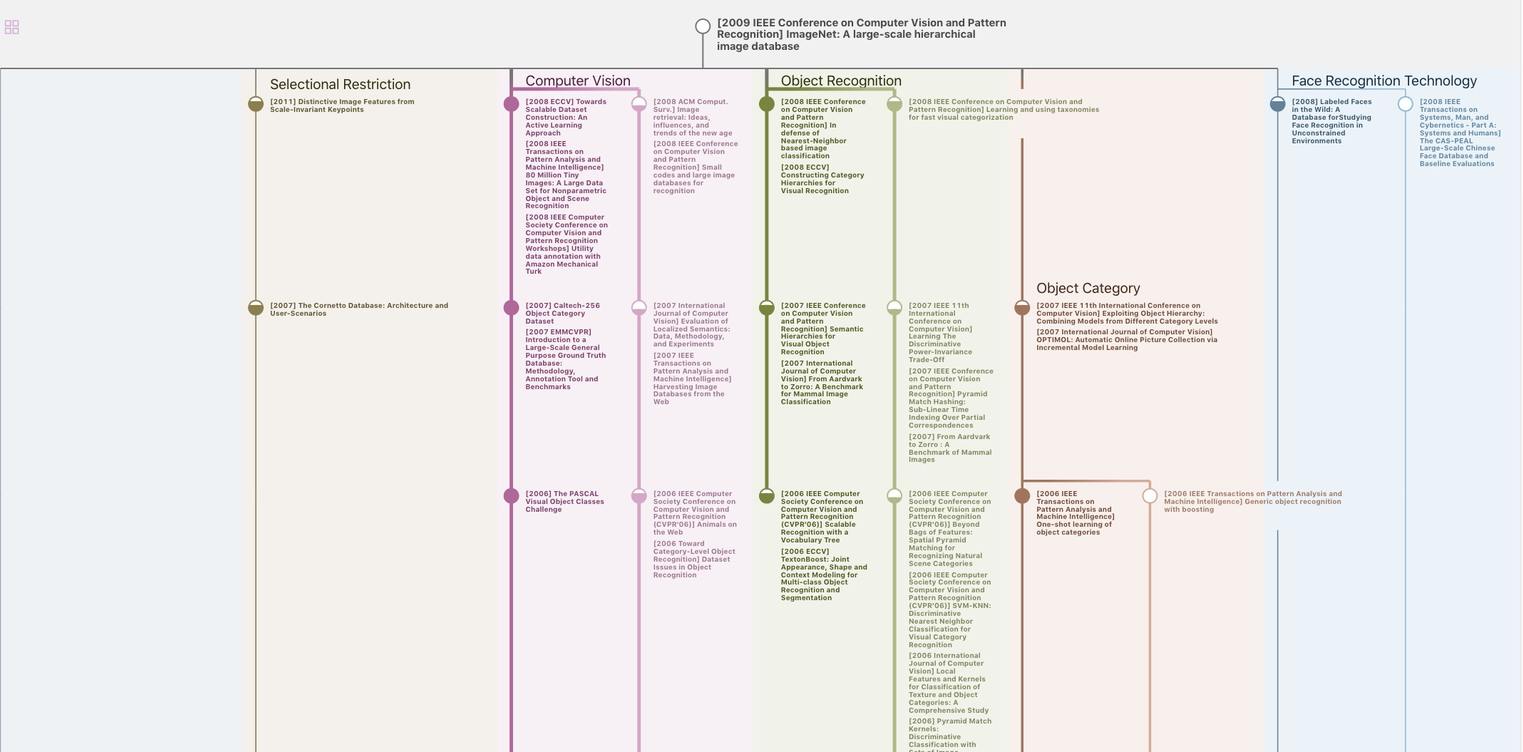
生成溯源树,研究论文发展脉络
Chat Paper
正在生成论文摘要