An Online Approach to Estimate Parameters of Phase-Type Distributions
2019 49th Annual IEEE/IFIP International Conference on Dependable Systems and Networks (DSN)(2019)
摘要
The traditional expectation-maximization (EM) algorithm is a general purpose algorithm for maximum likelihood estimation in problems with incomplete data. Several variants of the algorithm exist to estimate the parameters of phase-type distributions (PHDs), a widely used class of distributions in performance and dependability modeling. EM algorithms are typical offline algorithms because they improve the likelihood function by iteratively running through a fixed sample. Nowadays data can be generated online in most systems such that offline algorithms seem to be outdated in this environment. This paper proposes an online EM algorithm for parameter estimation of PHDs. In contrast to the offline version, the online variant adds data immediately when it becomes available and includes no iteration. Different variants of the algorithms are proposed that exploit the specific structure of subclasses of PHDs like hyperexponential, hyper-Erlang or acyclic PHDs. The algorithm furthermore incorporates current methods to detect drifts or change points in a data stream and estimates a new PHD whenever such a behavior has been identified. Thus, the resulting distributions can be applied for online model prediction and for the generation of inhomogeneous PHDs as an extension of inhomogeneous Poisson processes. Numerical experiments with artificial and measured data streams show the applicability of the approach.
更多查看译文
关键词
stochastic modeling,phase-type distributions,expectation-maximization algorithm,online algorithm,parameter estimation
AI 理解论文
溯源树
样例
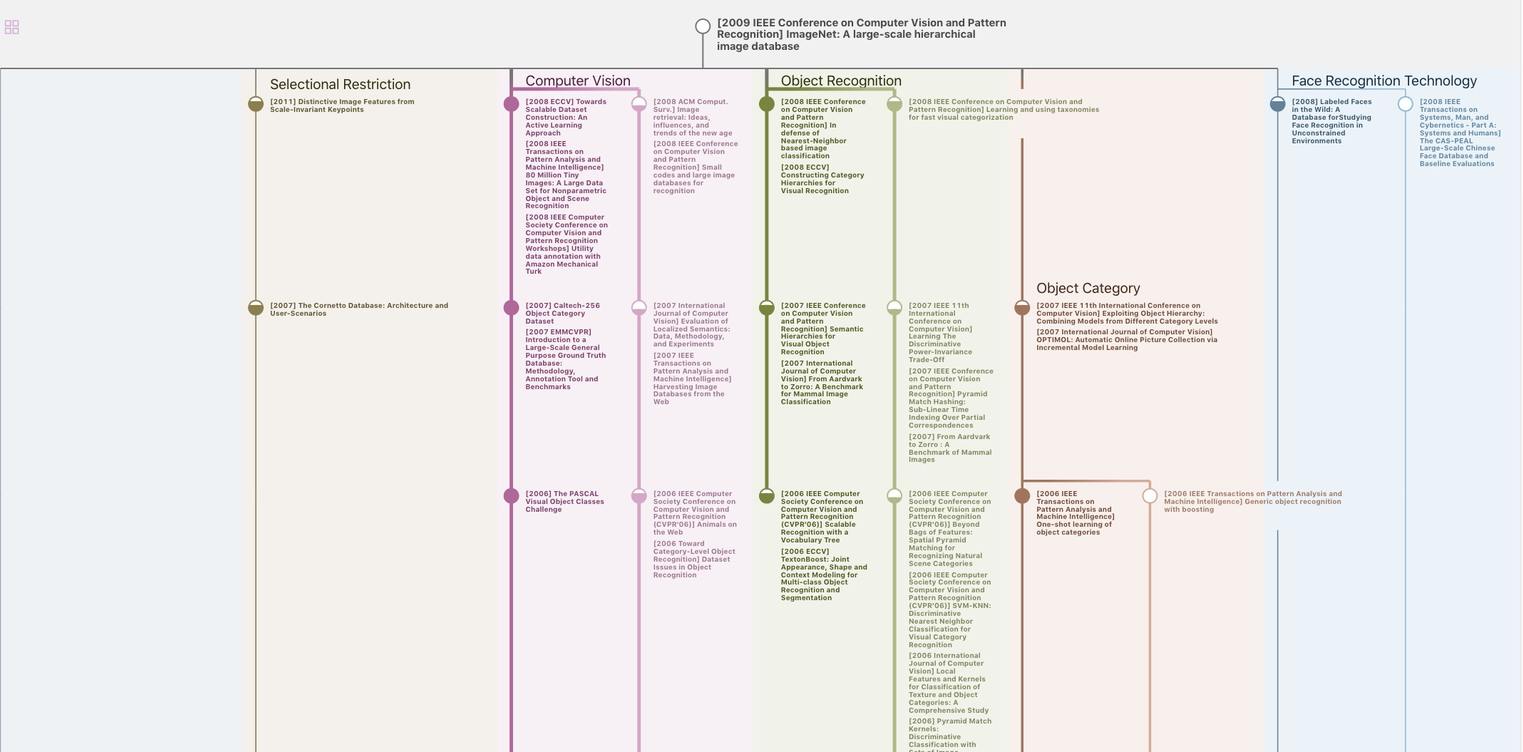
生成溯源树,研究论文发展脉络
Chat Paper
正在生成论文摘要