Few-Shot Learning with Deep Triplet Networks for Brain Imaging Modality Recognition
DART/MIL3ID@MICCAI(2019)
摘要
Image modality recognition is essential for efficient imaging workflows in current clinical environments, where multiple imaging modalities are used to better comprehend complex diseases. Emerging biomarkers from novel, rare modalities are being developed to aid in such understanding, however the availability of these images is often limited. This scenario raises the necessity of recognising new imaging modalities without them being collected and annotated in large amounts. In this work, we present a few-shot learning model for limited training examples based on Deep Triplet Networks. We show that the proposed model is more accurate in distinguishing different modalities than a traditional Convolutional Neural Network classifier when limited samples are available. Furthermore, we evaluate the performance of both classifiers when presented with noisy samples and provide an initial inspection of how the proposed model can incorporate measures of uncertainty to be more robust against out-of-sample examples.
更多查看译文
关键词
Brain imaging,Modality recognition,Few-shot learning,Triplet loss,Uncertainty,Noise
AI 理解论文
溯源树
样例
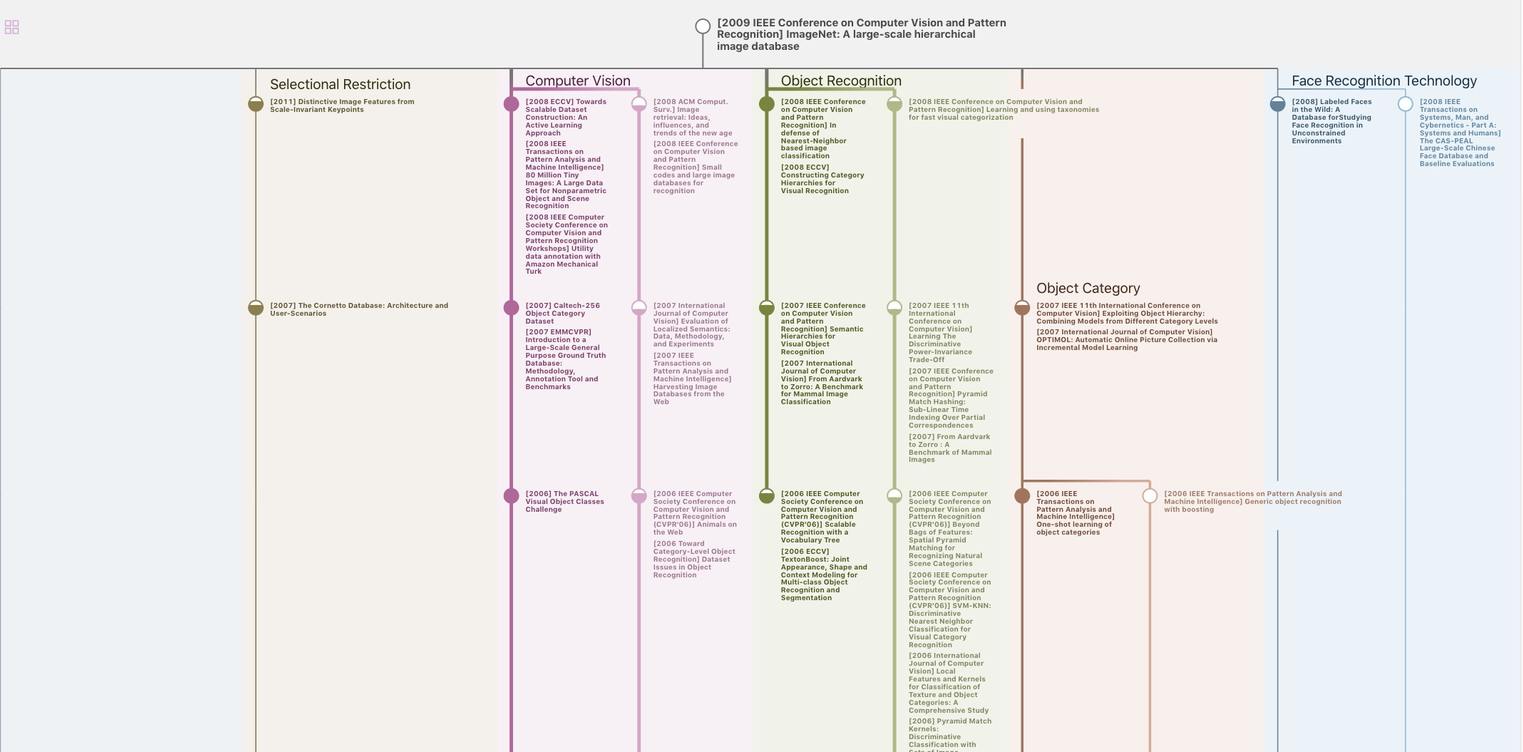
生成溯源树,研究论文发展脉络
Chat Paper
正在生成论文摘要