Increasing the Generalisaton Capacity of Conditional VAEs
ARTIFICIAL NEURAL NETWORKS AND MACHINE LEARNING - ICANN 2019: DEEP LEARNING, PT II(2019)
摘要
We address the problem of one-to-many mappings in supervised learning, where a single instance has many different solutions of possibly equal cost. The framework of conditional variational autoencoders describes a class of methods to tackle such structured-prediction tasks by means of latent variables. We propose to incentivise informative latent representations for increasing the generalisation capacity of conditional variational autoencoders. To this end, we modify the latent variable model by defining the likelihood as a function of the latent variable only and introduce an expressive multimodal prior to enable the model for capturing semantically meaningful features of the data. To validate our approach, we train our model on the Cornell Robot Grasping dataset, and modified versions of MNIST and Fashion-MNIST obtaining results that show a significantly higher generalisation capability.
更多查看译文
关键词
Structured prediction,Latent variable models,Conditional variational autoencoders,Empirical bayes
AI 理解论文
溯源树
样例
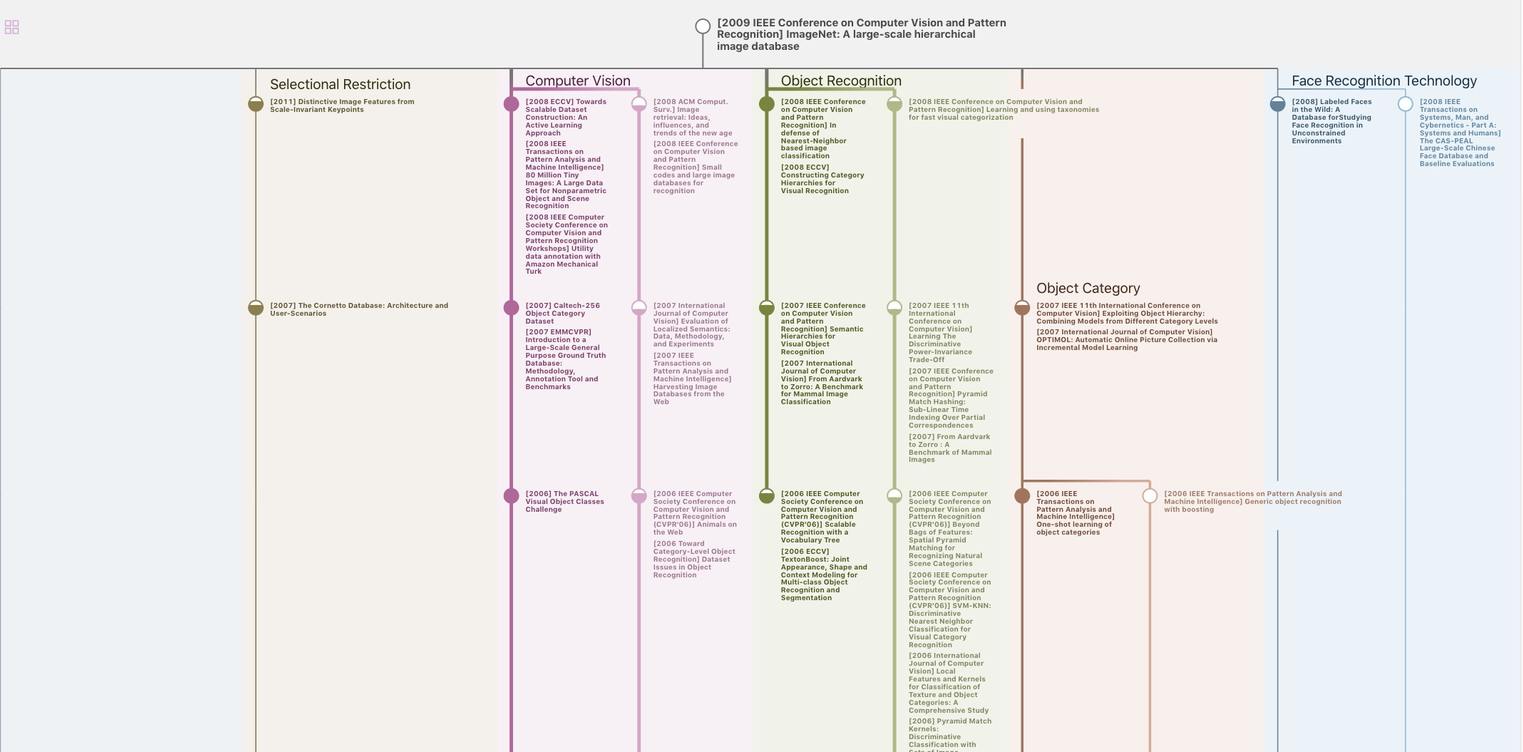
生成溯源树,研究论文发展脉络
Chat Paper
正在生成论文摘要