From Target to Drug: Generative Modeling for Multimodal Structure-Based Ligand Design.
MOLECULAR PHARMACEUTICS(2019)
摘要
Chemical space is impractically large, and conventional structure-based virtual screening techniques cannot be used to simply search through the entire space to discover effective bioactive molecules. To address this shortcoming, we propose a generative adversarial network to generate, rather than search, diverse three-dimensional ligand shapes complementary to the pocket. Furthermore, we show that the generated molecule shapes can be decoded using a shape-captioning network into a sequence of SMILES enabling directly the structure-based de novo drug design. We evaluate the quality of the method by both structure(docking) and ligand-based [quantitative structure-activity relationship (QSAR)] virtual screening methods. For both evaluation approaches, we observed enrichment compared to random sampling from initial chemical space of ZINC drug-like compounds.
更多查看译文
关键词
structure based drug design,deep learning,generative modeling
AI 理解论文
溯源树
样例
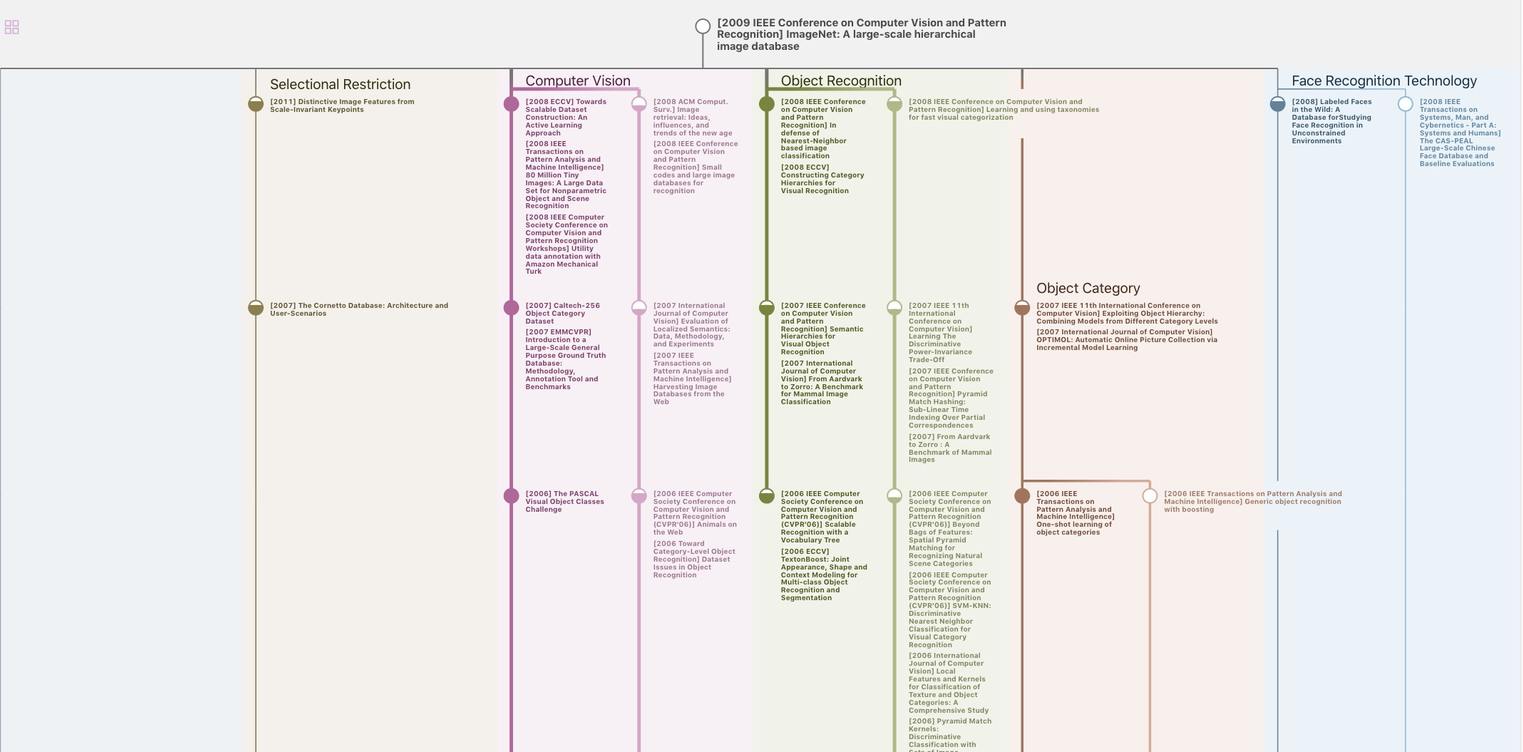
生成溯源树,研究论文发展脉络
Chat Paper
正在生成论文摘要