Mono-Sf: Multi-View Geometry Meets Single-View Depth For Monocular Scene Flow Estimation Of Dynamic Traffic Scenes
2019 IEEE/CVF INTERNATIONAL CONFERENCE ON COMPUTER VISION (ICCV 2019)(2019)
摘要
Existing 3D scene flow estimation methods provide the 3D geometry and 3D motion of a scene and gain a lot of interest, for example in the context of autonomous driving. These methods are traditionally based on a temporal series of stereo images. In this paper, we propose a novel monocular 3D scene flow estimation method, called Mono-SF. Mono-SF jointly estimates the 3D structure and motion of the scene by combining multi-view geometry and single-view depth information. Mono-SF considers that the scene flow should be consistent in terms of warping the reference image in the consecutive image based on the principles of multi-view geometry. For integrating single-view depth in a statistical manner, a convolutional neural network, called ProbDepthNet, is proposed. ProbDepthNet estimates pixel-wise depth distributions from a single image rather than single depth values. Additionally, as part of ProbDepthNet, a novel recalibration technique for regression problems is proposed to ensure well-calibrated distributions. Our experiments show that Mono-SF outperforms state-of-the-art monocular baselines and ablation studies support the Mono-SF approach and ProbDepthNet design.
更多查看译文
关键词
multiview geometry meets single-view depth,monocular scene flow estimation,dynamic traffic scenes,scene flow estimation methods,stereo images,monocular 3D,single-view depth information,reference image,consecutive image,pixel-wise depth distributions,single depth values,Mono-SF approach
AI 理解论文
溯源树
样例
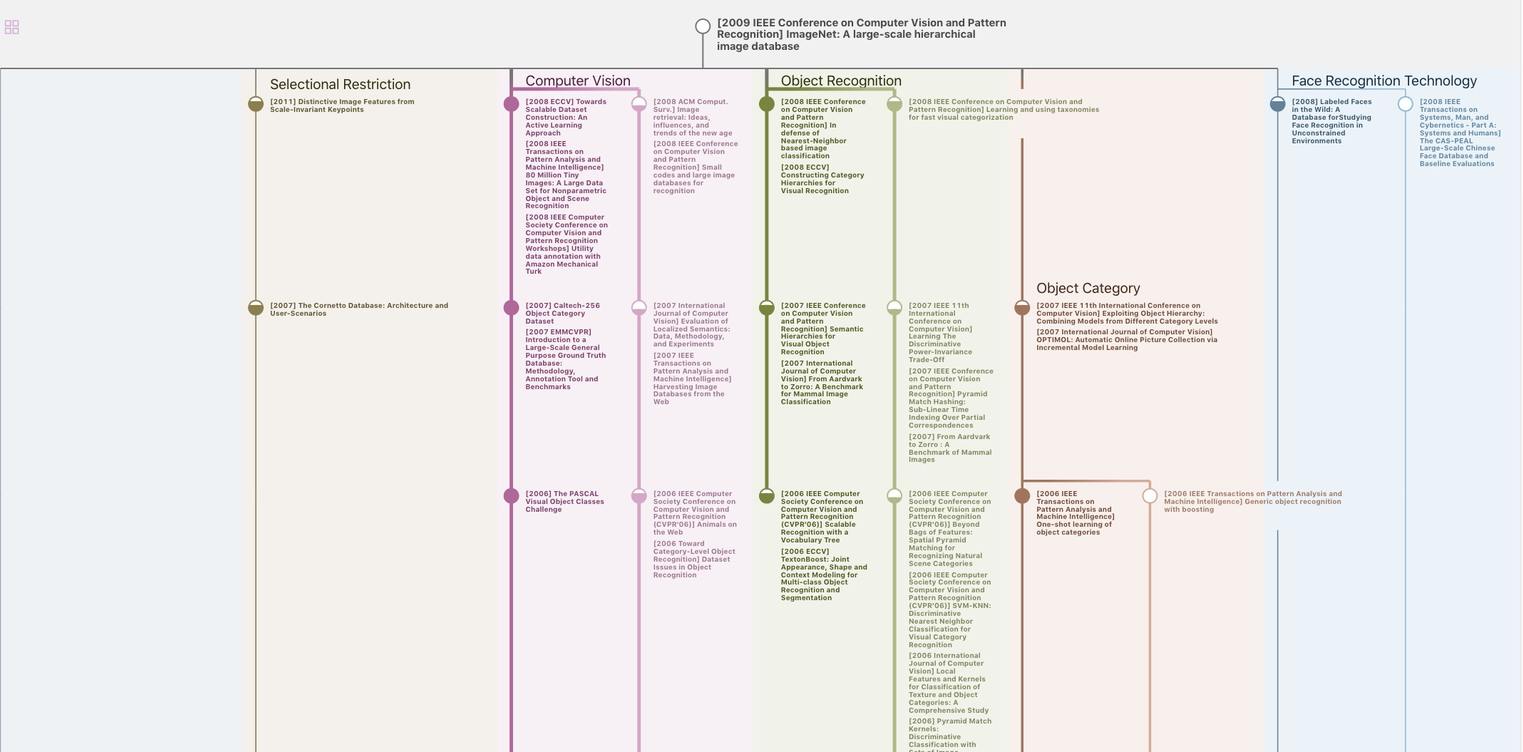
生成溯源树,研究论文发展脉络
Chat Paper
正在生成论文摘要