Fast multi-component analysis using a joint sparsity constraint for MR fingerprinting.
MAGNETIC RESONANCE IN MEDICINE(2020)
摘要
Purpose To develop an efficient algorithm for multi-component analysis of magnetic resonance fingerprinting (MRF) data without making a priori assumptions about the exact number of tissues or their relaxation properties. Methods Different tissues or components within a voxel are potentially separable in MRF because of their distinct signal evolutions. The observed signal evolution in each voxel can be described as a linear combination of the signals for each component with a non-negative weight. An assumption that only a small number of components are present in the measured field of view is usually imposed in the interpretation of multi-component data. In this work, a joint sparsity constraint is introduced to utilize this additional prior knowledge in the multi-component analysis of MRF data. A new algorithm combining joint sparsity and non-negativity constraints is proposed and compared to state-of-the-art multi-component MRF approaches in simulations and brain MRF scans of 11 healthy volunteers. Results Simulations and in vivo measurements show reduced noise in the estimated tissue fraction maps compared to previously proposed methods. Applying the proposed algorithm to the brain data resulted in 4 or 5 components, which could be attributed to different brain structures, consistent with previous multi-component MRF publications. Conclusions The proposed algorithm is faster than previously proposed methods for multi-component MRF and the simulations suggest improved accuracy and precision of the estimated weights. The results are easier to interpret compared to voxel-wise methods, which combined with the improved speed is an important step toward clinical evaluation of multi-component MRF.
更多查看译文
关键词
joint sparsity constraint,MR fingerprinting,multi-component analysis,NNLS,partial volume effect,Sparsity Promoting Iterative Joint NNLS (SPIJN)
AI 理解论文
溯源树
样例
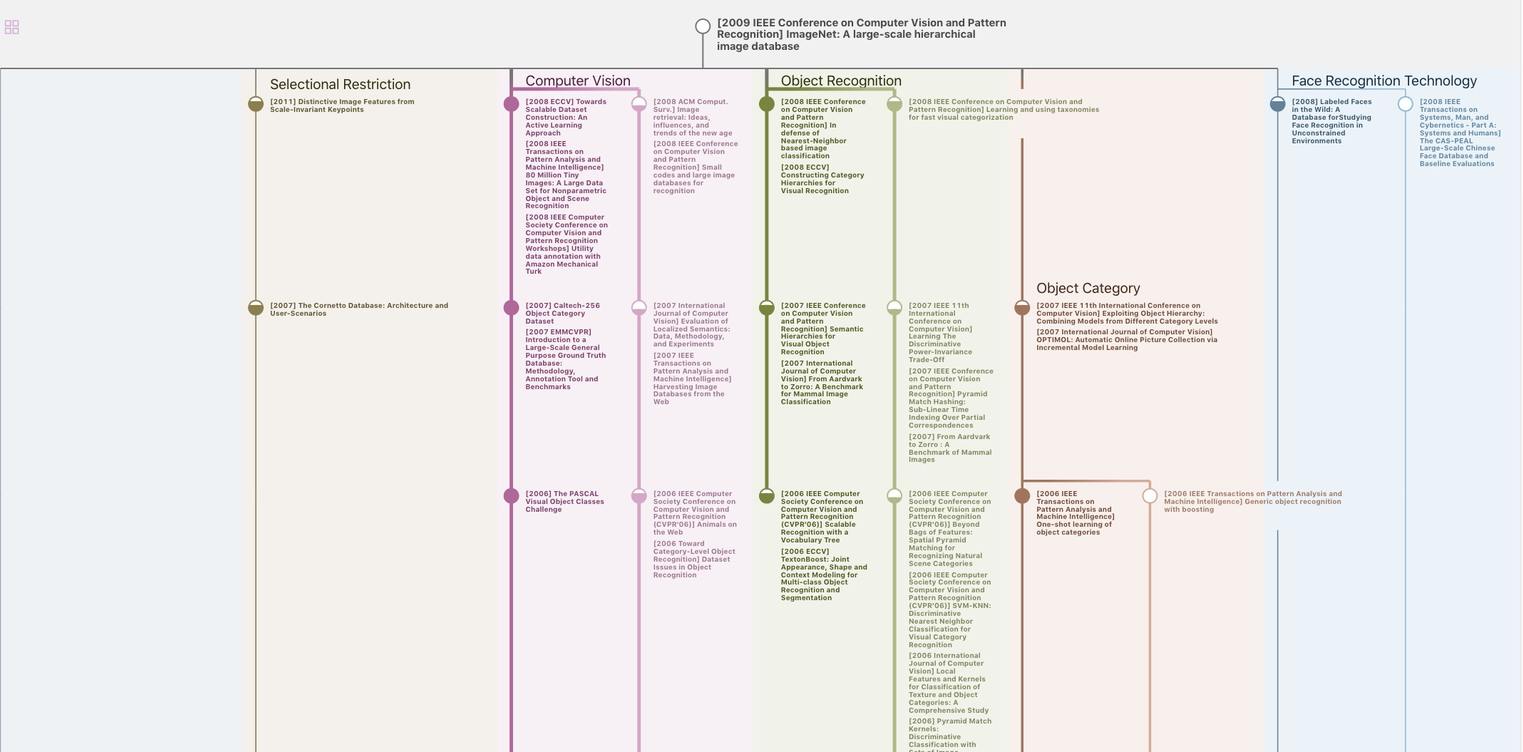
生成溯源树,研究论文发展脉络
Chat Paper
正在生成论文摘要