Improving Haptic Adjective Recognition With Unsupervised Feature Learning
2019 INTERNATIONAL CONFERENCE ON ROBOTICS AND AUTOMATION (ICRA)(2019)
摘要
Humans can form an impression of how a new object feels simply by touching its surfaces with the densely innervated skin of the fingertips. Many haptics researchers have recently been working to endow robots with similar levels of haptic intelligence, but these efforts almost always employ hand-crafted features, which are brittle, and concrete tasks, such as object recognition. We applied unsupervised feature learning methods, specifically K-SVD and Spatio-Temporal Hierarchical Matching Pursuit (ST-HMP), to rich multi-modal haptic data from a diverse dataset. We then tested the learned features on 19 more abstract binary classification tasks that center on haptic adjectives such as smooth and squishy. The learned features proved superior to traditional hand-crafted features by a large margin, almost doubling the average F-1 score across all adjectives. Additionally, particular exploratory procedures (EPs) and sensor channels were found to support perception of certain haptic adjectives, underlining the need for diverse interactions and multi-modal haptic data.
更多查看译文
关键词
unsupervised feature learning,densely innervated skin,haptics researchers,haptic intelligence,concrete tasks,object recognition,feature learning methods,haptic adjectives,diverse interactions,abstract binary classification tasks,spatio-temporal hierarchical matching pursuit,haptic adjective recognition
AI 理解论文
溯源树
样例
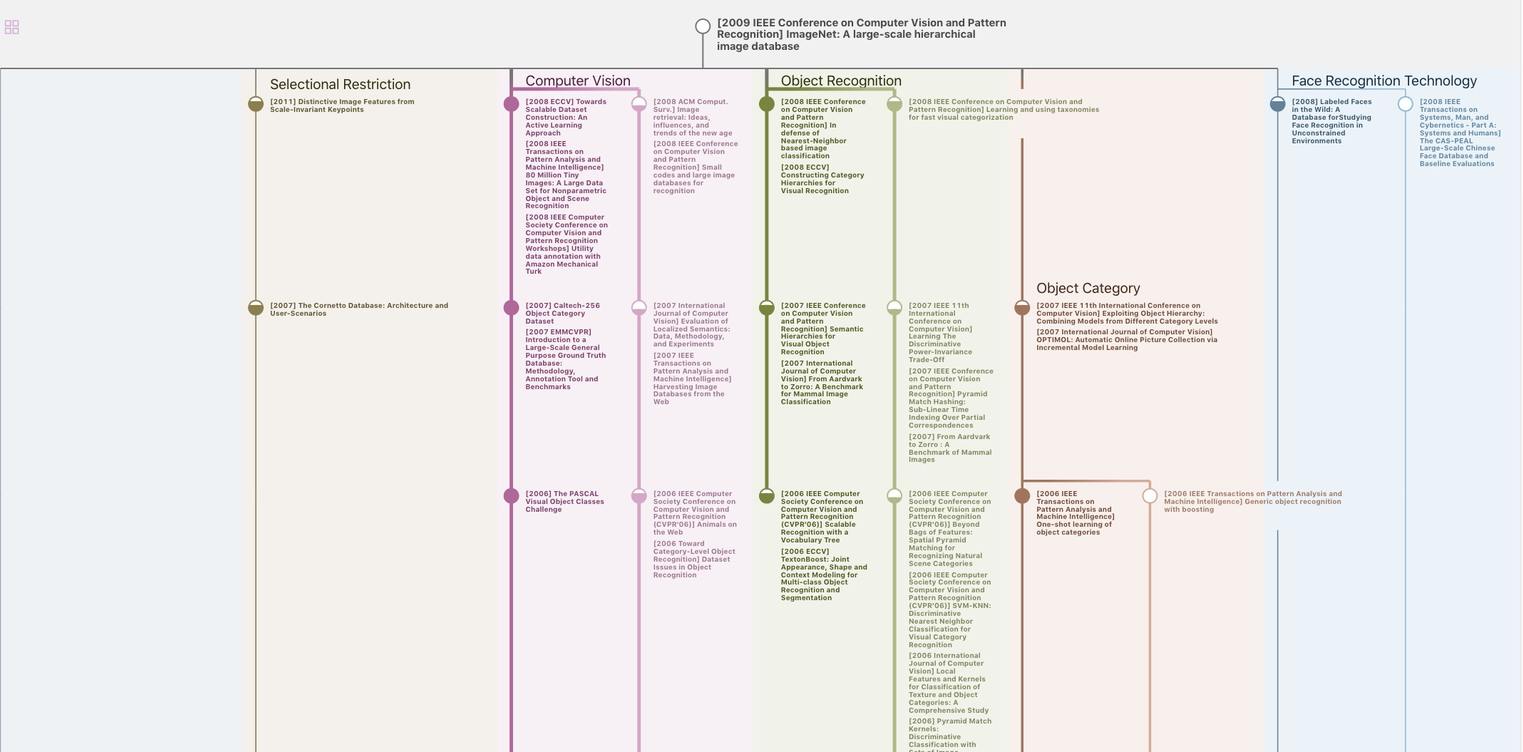
生成溯源树,研究论文发展脉络
Chat Paper
正在生成论文摘要