Machine Learning-based Prediction of Test Power
2019 IEEE European Test Symposium (ETS)(2019)
摘要
With the increase in circuit complexity, the gap between circuit development time and analysis time has widened. A large database is required in order to perform essential analysis tasks such as power, thermal, and IR-drop analysis, which, in turn, leads to long run times. This work focuses on test power analysis. Due to the large number of test patterns for modern designs and the excessive power analysis run time for each test, it is not feasible to obtain complete power profiles for all the tests. However, test power-safety is essential to produce reliable manufacturing test results and prevent yield loss and chip damage. Accurate power profiling can typically be done for a small subset of pre-selected tests only. An essential task is therefore to determine those tests, which potentially provide the worst-case scenarios with respect to test power. We propose machine learning-based power prediction for test selection. The prediction is applied in two different ways. First, we predict the activity of a test to identify tests with high power consumption. Second, the switching activity and the power information are related to the layout of the chip to identify local hot spots. Various machine learning-based algorithms are used to evaluate this approach. Additionally, the algorithms are compared against each other. The results indicate high prediction accuracy and effectiveness. This makes these algorithms well suited for worst-case test selection.
更多查看译文
关键词
circuit complexity,circuit development time,IR-drop analysis,test power analysis,test power-safety,pre-selected tests,machine learning-based power prediction,high power consumption,power information,power profiles,reliable manufacturing test,chip layout,local hot spot identification
AI 理解论文
溯源树
样例
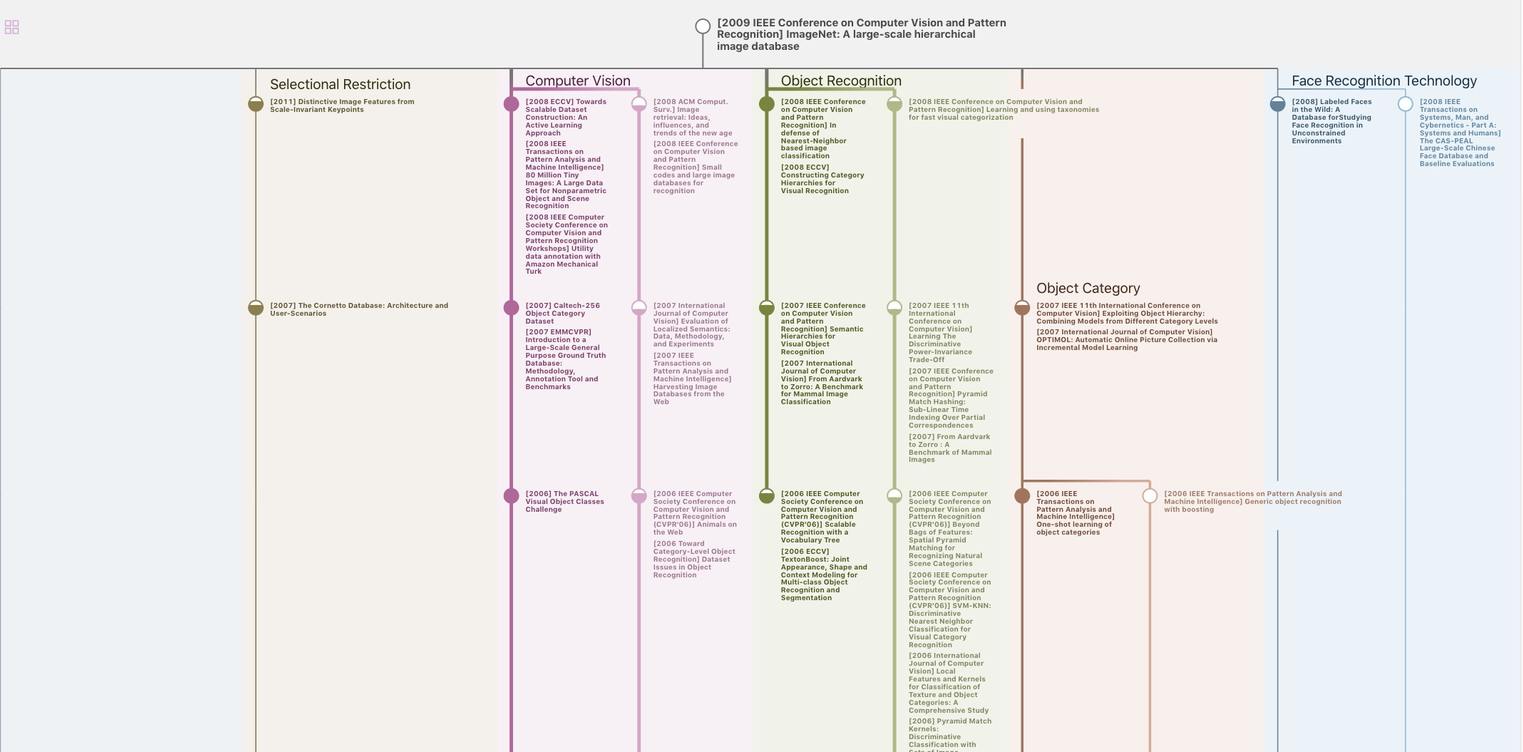
生成溯源树,研究论文发展脉络
Chat Paper
正在生成论文摘要