An Ontology-based Two-Stage Approach to Medical Text Classification with Feature Selection by Particle Swarm Optimisation
2019 IEEE Congress on Evolutionary Computation (CEC)(2019)
摘要
Document classification (DC) is the task of assigning pre-defined labels to unseen documents by utilizing a model trained on the available labeled documents. DC has attracted much attention in medical fields recently because many issues can be formulated as a classification problem. It can assist doctors in decision making and correct decisions can reduce the medical expenses. Medical documents have special attributes that distinguish them from other texts and make them difficult to analyze. For example, many acronyms and abbreviations, and short expressions make it more challenging to extract information. The classification accuracy of the current medical DC methods is not satisfactory. The goal of this work is to enhance the input feature sets of the DC method to improve the accuracy. To approach this goal, a novel two-stage approach is proposed. In the first stage, a domain-specific dictionary, namely the Unified Medical Language System (UMLS), is employed to extract the key features belonging to the most relevant concepts such as diseases or symptoms. In the second stage, PSO is applied to select more related features from the extracted features in the first stage. The performance of the proposed approach is evaluated on the 2010 Informatics for Integrating Biology and the Bedside (i2b2) data set which is a widely used medical text dataset. The experimental results show substantial improvement by the proposed method on the accuracy of classification.
更多查看译文
关键词
Medical Text Classification,Particle Swarm Optimization,Feature Selection,Conceptualization,Ontology
AI 理解论文
溯源树
样例
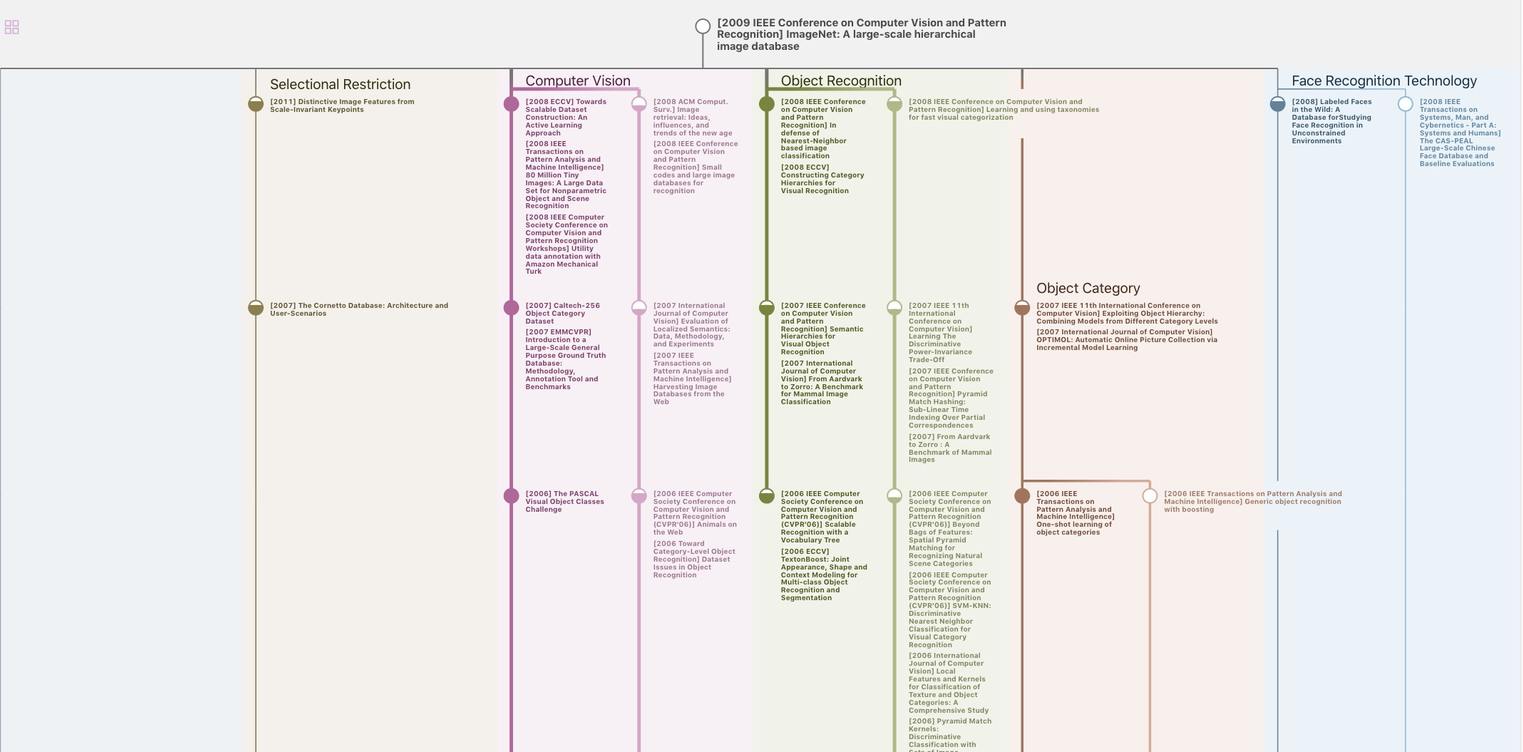
生成溯源树,研究论文发展脉络
Chat Paper
正在生成论文摘要