Multi-layered Learning for Information Extraction from Adverse Drug Event Narratives.
BIOMEDICAL ENGINEERING SYSTEMS AND TECHNOLOGIES, BIOSTEC 2018(2019)
摘要
Recognizing named entities in Adverse Drug Reactions narratives is a crucial step towards extracting valuable patient information from unstructured text and transforming the information into an easily processable structured format. This motivates using advanced data analytics to support data-driven pharmacovigilance. Yet existing biomedical named entity recognition (NER) tools are limited in their ability to identify certain entity types from these domain-specific narratives, resulting in poor accuracy. To address this shortcoming, we propose our novel methodology called Tiered Ensemble Learning System with Diversity (TELS-D), an ensemble approach that integrates a rich variety of named entity recognizers to procure the final result. There are two specific challenges faced by biomedical NER: the classes are imbalanced and the lack of a single best performing method. The first challenge is addressed through a balanced, under-sampled bagging strategy that depends on the imbalance level to overcome this highly skewed data problem. To address the second challenge, we design an ensemble of heterogeneous entity recognizers that leverages a novel ensemble combiner. Our experimental results demonstrate that for biomedical text datasets: (i) a balanced learning environment combined with an ensemble of heterogeneous classifiers consistently improves the performance over individual base learners and (ii) stacking-based ensemble combiner methods outperform simple majority voting based solutions by 0.3 in F1-score.
更多查看译文
关键词
Pharmacovigilance,Adverse Drug Reaction,Class imbalance,Ensemble learning
AI 理解论文
溯源树
样例
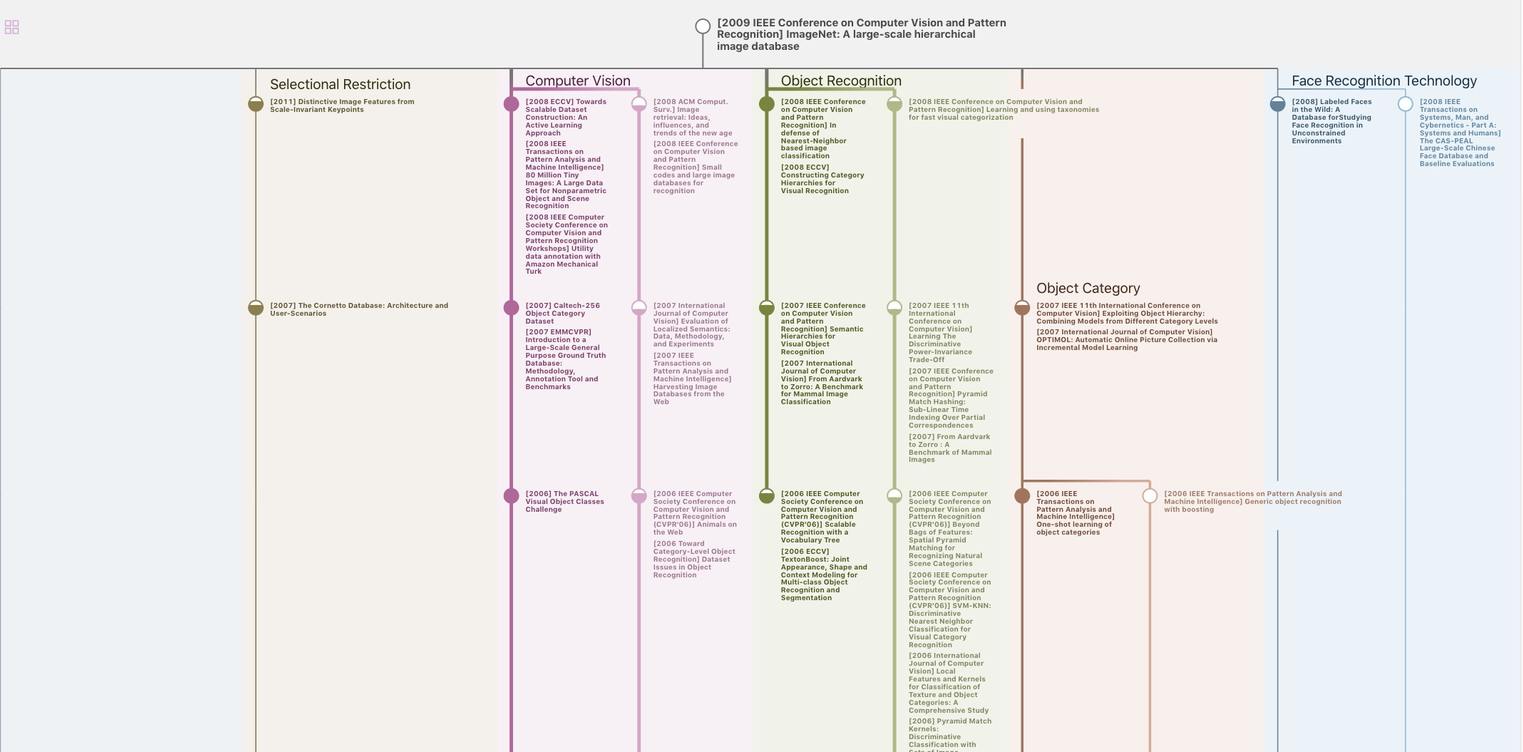
生成溯源树,研究论文发展脉络
Chat Paper
正在生成论文摘要