Inductive Context-aware Process Discovery
2019 International Conference on Process Mining (ICPM)(2019)
摘要
Discovery plays a key role in data-driven analysis of business processes. The vast majority of contemporary discovery algorithms aims at the identification of control-flow constructs. The increase in data richness, however, enables discovery that incorporates the context of process execution beyond the control-flow perspective. A "control-flow first" approach, where context data serves for refinement and annotation, is limited and fails to detect fundamental changes in the control-flow that depend on context data. In this work, we thus propose a novel approach for combining the control-flow and data perspectives under a single roof by extending inductive process discovery. Our approach provides criteria under which context data, handled through unsupervised learning, take priority over control-flow in guiding process discovery. The resulting model is a process tree, in which some operators carry data semantics instead of control-flow semantics. We evaluate the approach using synthetic and real-world datasets and show that the resulting models are superior to state-of-the-art discovery methods in terms of measures that are based on multi perspective alignments.
更多查看译文
关键词
Business Process Discovery,Unsupervised Learning,Data Analysis
AI 理解论文
溯源树
样例
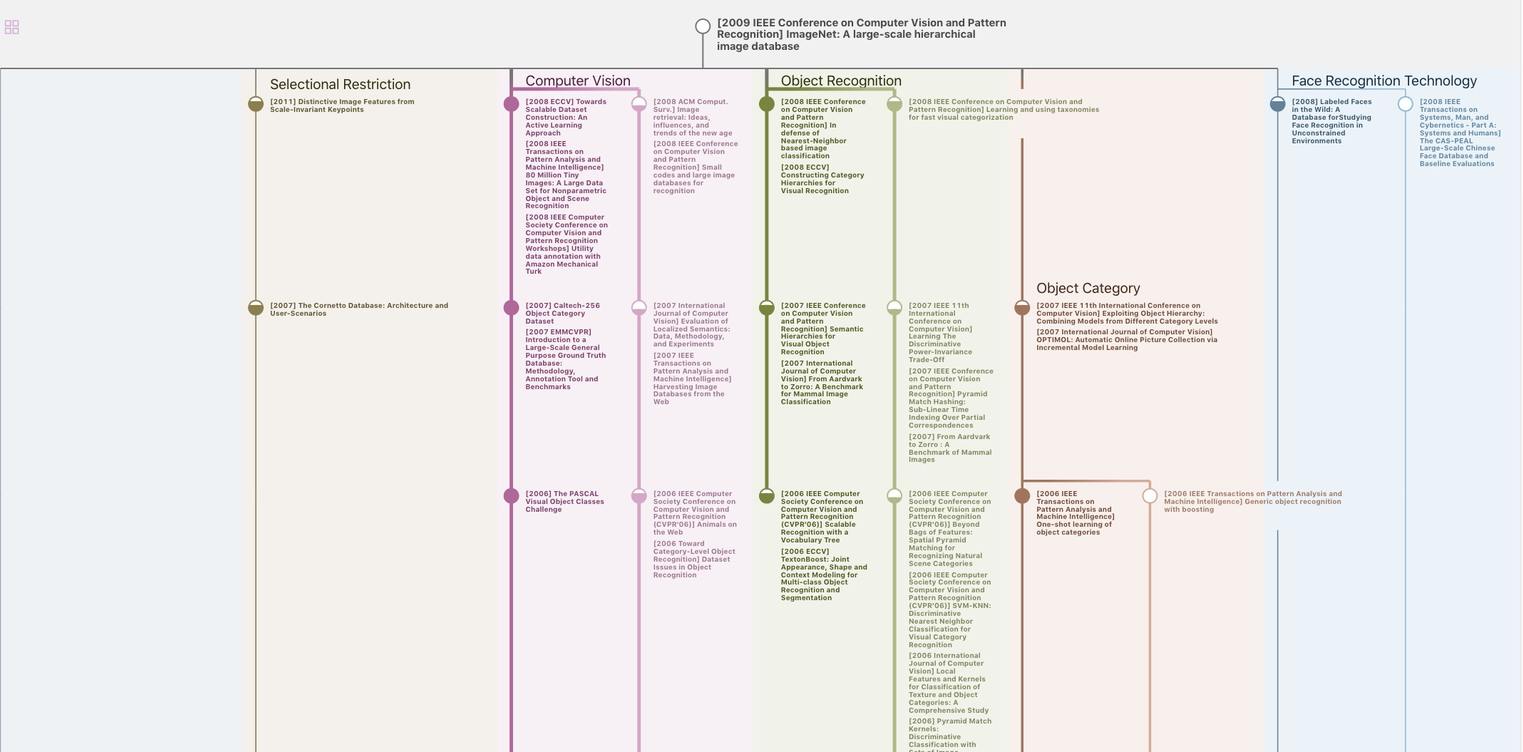
生成溯源树,研究论文发展脉络
Chat Paper
正在生成论文摘要