Similarity-Aware Deep Adversarial Learning For Facial Age Estimation
2019 IEEE INTERNATIONAL CONFERENCE ON MULTIMEDIA AND EXPO (ICME)(2019)
摘要
In this paper, we propose a similarity-aware deep adversarial learning (SADAL) approach for facial age estimation. Instead of making access to limited training samples which likely leads to sub-optima, our SADAL seeks sets of unobserved and plausible hard-examples based on existing training samples, which typically reinforces the discriminativeness of the learned feature descriptor for ages. Motivated by the fact that age labels are usually correlated in the real-world applications, we carefully develop a similarity-aware function in our approach, which dynamically measures each face pair with different weights based on different age value gaps. During the learning process, we jointly optimize both procedures of generating hard-examples and learning age estimator via a sequence of adversarial-game iterations. As a result, the smoothing aging pattern is exploited in the reconstructed hardexample space for robust age estimation. Experimental results on two standard benchmarking datasets show that our approach achieves superior performance compared with most state-of-the-art age estimation methods.
更多查看译文
关键词
Facial age estimation, deep adversarial learning, biometrics
AI 理解论文
溯源树
样例
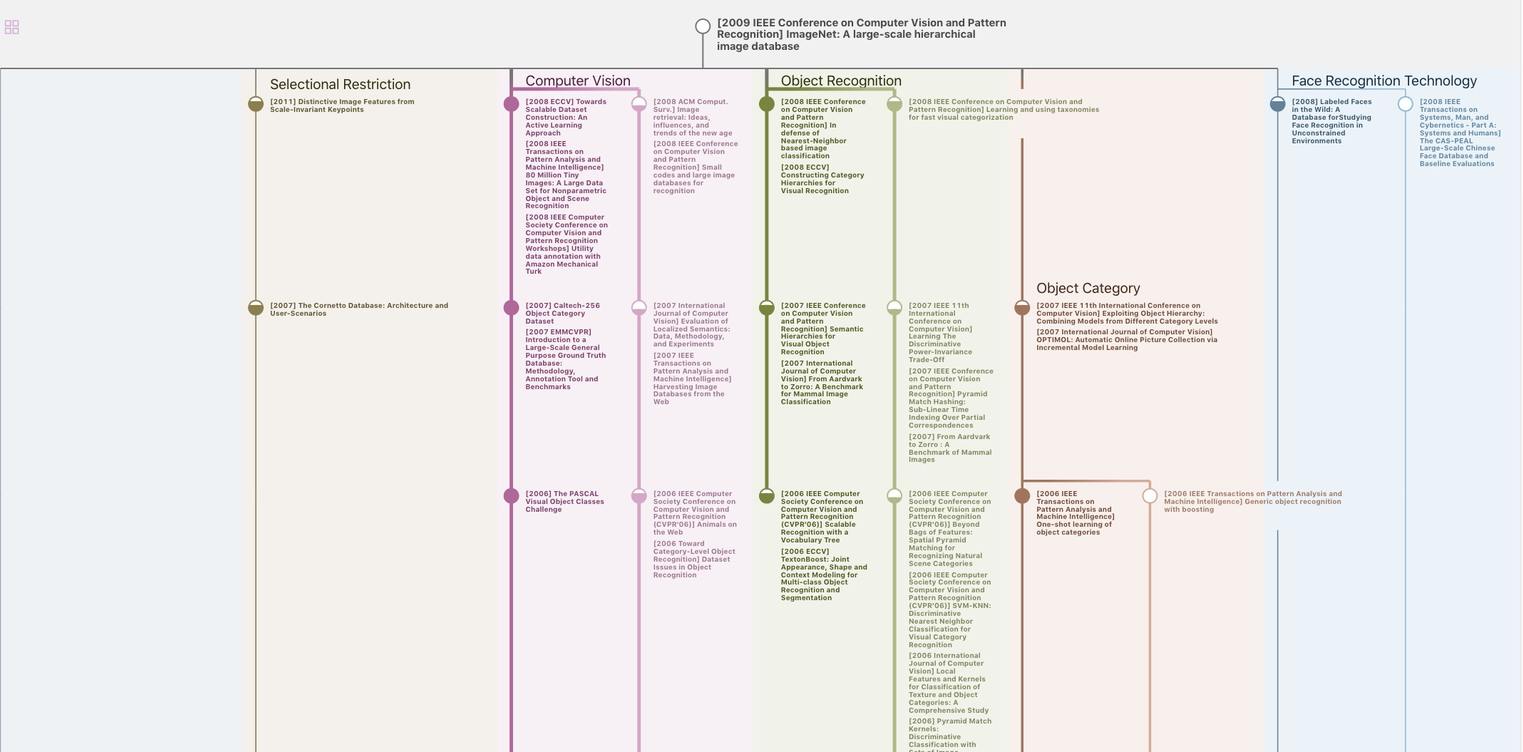
生成溯源树,研究论文发展脉络
Chat Paper
正在生成论文摘要