Residual Magnifier: A Dense Information Flow Network for Super Resolution
2019 IEEE International Conference on Multimedia and Expo (ICME)(2019)
摘要
Recently, deep learning methods have been successfully applied to single image super-resolution tasks. However, some networks with extreme depth failed to achieve better performance because of the insufficient utilization of the local residual information extracted at each stage. To solve the above question, we propose a Dense Information Flow Network (DIF-Net), which can fully extract and utilize the local residual information at each stage to accomplish a better reconstruction. Specifically, we present a Two-stage Residual Extraction Block (TREB) to extract the shallow and deep local residual information at each stage. The dense connection mechanism is introduced throughout the model and within TREBs to dramatically increase the information flow. Meanwhile this mechanism prevents the shallow features extracted earlier from being diluted. Finally, we propose a lightweight subnet (residual enhancer) to efficiently recycle the overflow residual information from the backbone net for detail enhancement of the residual image. Experimental results demonstrate that the proposed method performs favorably against the state-of-the-art methods with relatively-less parameters.
更多查看译文
关键词
Single image super-resolution, Enhanced residual information, Dense connection
AI 理解论文
溯源树
样例
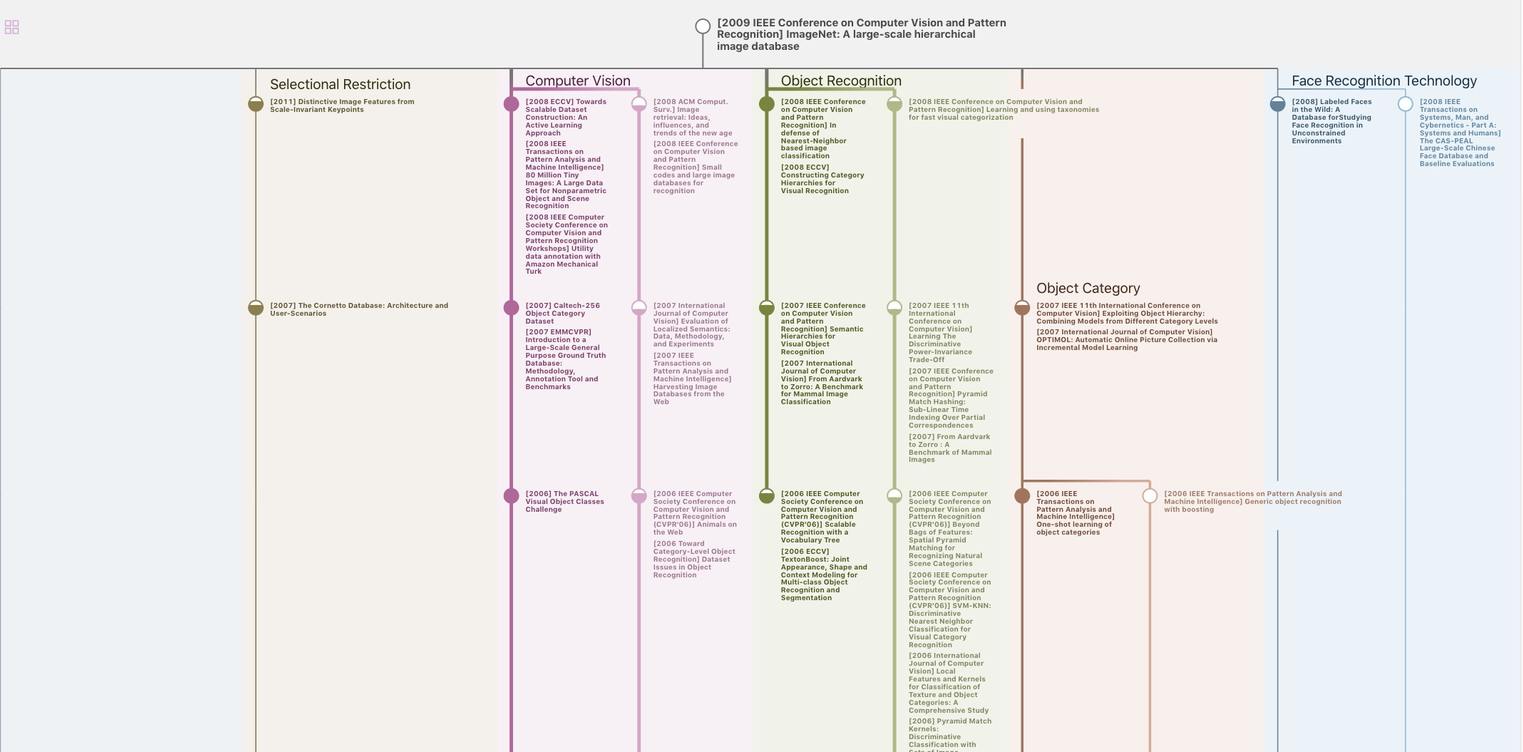
生成溯源树,研究论文发展脉络
Chat Paper
正在生成论文摘要