Deep Pyramid Variation Learning for Image Interpolation
2019 IEEE International Conference on Multimedia and Expo (ICME)(2019)
摘要
Previous learning-based interpolation methods do not consider multi-scale structural information, which is generally effective for image modeling. In this paper, we design a deep network based on a novel pyramid variation learning approach with multi-scale structure modeling. An image is represented as multi-dimensional features. Besides two spatial dimensions, the features include a neighboring variation dimension where every pixel is encoded as the variation to its nearest low-resolution pixel, and a scale dimension along which the feature maps generated by a gradual down-sampling process are stacked. Thus, these multi-dimensional features are constructed to model local dependency and multi-scale similarity jointly. Inspired by this feature design, we build an end-to-end trainable Recurrent Multi-Path Aggregation Network (RMPAN) for image interpolation, where the scale dimension is unfolded to form a multi-path aggregation network to apply joint filters at different scales recurrently. Location aware sampling layers are used in RMPAN to transform feature maps into different scales with only location changes in each convolution path, which aggregate the context information without resolution loss. Comprehensive experiments demonstrate that our method leads to a superior performance and offers new state-of-the-art benchmark.
更多查看译文
关键词
Deep learning, image interpolation, multi-scale similarity, pyramid structure, variation learning
AI 理解论文
溯源树
样例
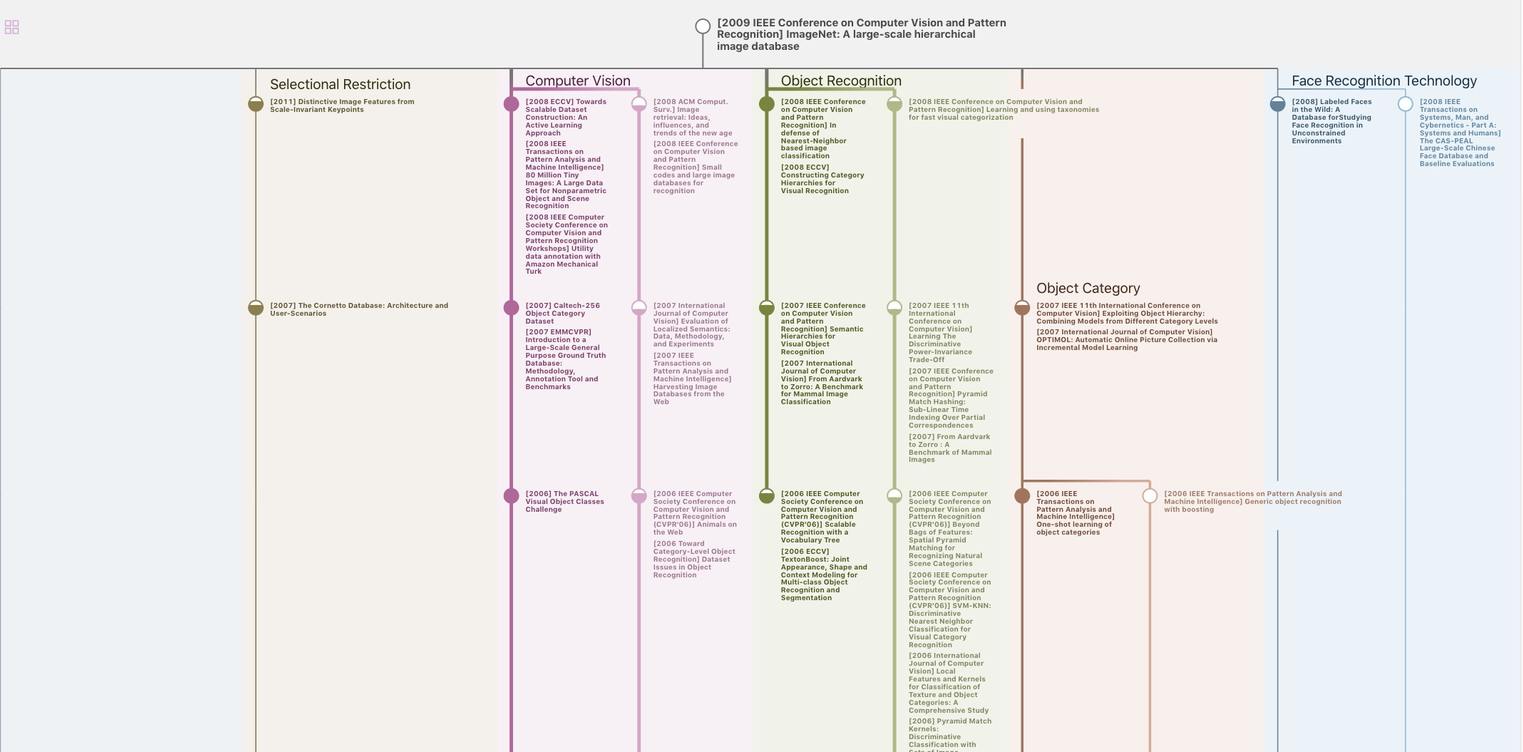
生成溯源树,研究论文发展脉络
Chat Paper
正在生成论文摘要