Part-Preserving Pose Manipulation For Person Image Synthesis
2019 IEEE INTERNATIONAL CONFERENCE ON MULTIMEDIA AND EXPO (ICME)(2019)
摘要
Manipulating person images under diverse poses, which transfers a person from one pose to another desired pose, is an interesting yet challenging task due to large non-rigid spatial deformation. Most existing works fail to preserve the fine-grained appearance consistency along with the pose changes due to the lack of explicit constraints and spatial modeling, leading to unrealistic results with severe artifacts. In this paper, we propose a novel Part-Preserving Generative Adversarial Network (PP-GAN) to achieve good manipulation quality by explicitly enforcing rich structure constraints over generative modeling. PP-GAN is proposed to decompose the challenging spatial transformation of the whole body into fine-grained part-level transformations, which are then integrated via human joint structure constraint. Given arbitrary poses, PP-GAN integrates human joint structure and region-level part cues as inputs to perform explicit generative modeling. Besides, we introduce a parsing-consistent loss to enforce semantic consistency among images with diverse poses, which guides the image synthesis from a semantic perspective. Extensive qualitative and quantitative evaluations on two benchmarks show that our PP-GAN significantly outperforms the state-of-the-art baselines in generating more realistic and plausible image synthesis results. PP-GAN successfully preserves part-level characteristics even for most challenging pose changes while prior works are easy to fail.
更多查看译文
关键词
Person Image Synthesis, Generative Adversarial Network, Human Parsing
AI 理解论文
溯源树
样例
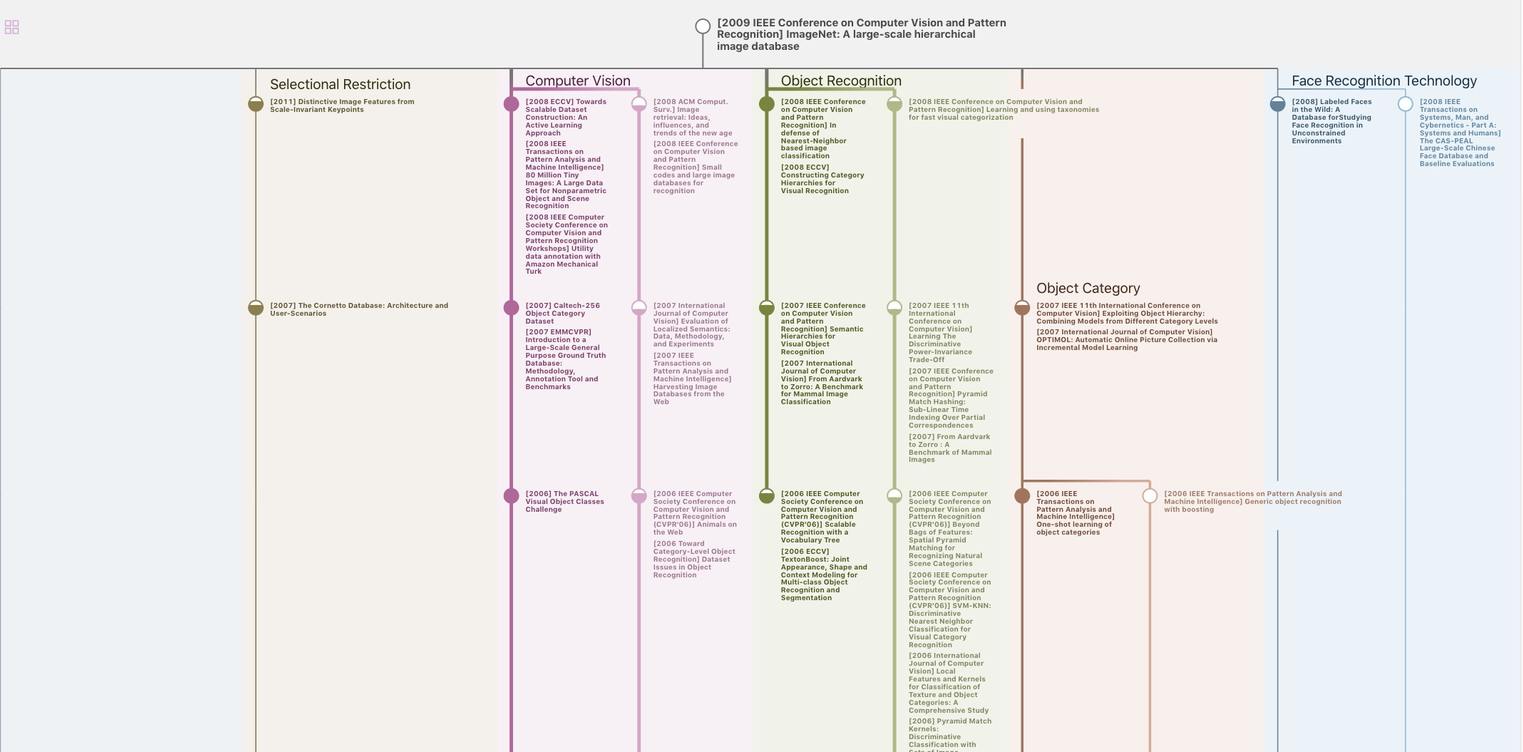
生成溯源树,研究论文发展脉络
Chat Paper
正在生成论文摘要