An Investigation of Interpretable Deep Learning for Adverse Drug Event Prediction
2019 IEEE 32ND INTERNATIONAL SYMPOSIUM ON COMPUTER-BASED MEDICAL SYSTEMS (CBMS)(2019)
摘要
A variety of deep learning architectures have been developed for the goal of predictive modelling in regards to detecting health diagnoses in medical records. Several models have placed strong emphases on temporal attention mechanisms and decay factors as a means to include highly temporally relevant information regarding the recency of medical event occurrence while facilitating medical code-level interpretability. In this study we utilise such models with a novel Electronic Patient Record (EPR) data set consisting of both diagnoses and medication data for the purpose of Adverse Drug Event (ADE) prediction. As such, a main contribution of this work is an empirical evaluation of two state-of-the-art deep learning architectures in terms of objective performance metrics for ADE prediction. We also assess the importance of attention mechanisms in regards to their usefulness for medical code-level interpretability, which may facilitate novel insights pertaining to the nature of ADE occurrence within the health care domain.
更多查看译文
关键词
Deep Learning, Attention mechanism, Adverse Drug Events, Medical records
AI 理解论文
溯源树
样例
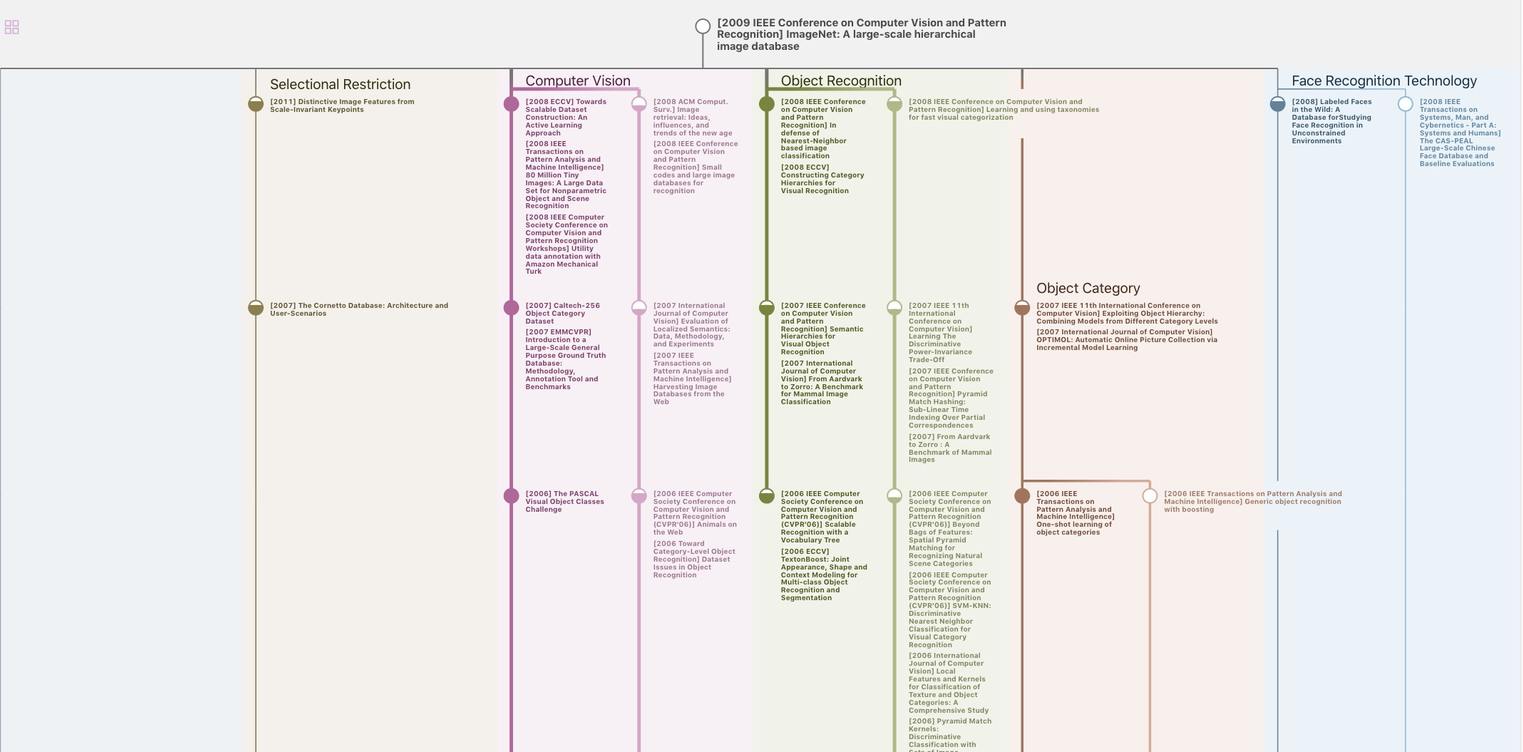
生成溯源树,研究论文发展脉络
Chat Paper
正在生成论文摘要