Learning Deep Landmarks for Imbalanced Classification
IEEE Transactions on Neural Networks and Learning Systems(2020)
摘要
We introduce a deep imbalanced learning framework called learning DEep Landmarks in laTent spAce (DELTA). Our work is inspired by the shallow imbalanced learning approaches to rebalance imbalanced samples before feeding them to train a discriminative classifier. Our DELTA advances existing works by introducing the new concept of rebalancing samples in a deeply transformed latent space, where latent points exhibit several desired properties including compactness and separability. In general, DELTA simultaneously conducts feature learning, sample rebalancing, and discriminative learning in a joint, end-to-end framework. The framework is readily integrated with other sophisticated learning concepts including latent points oversampling and ensemble learning. More importantly, DELTA offers the possibility to conduct imbalanced learning with the assistancy of structured feature extractor. We verify the effectiveness of DELTA not only on several benchmark data sets but also on more challenging real-world tasks including click-through-rate (CTR) prediction, multi-class cell type classification, and sentiment analysis with sequential inputs.
更多查看译文
关键词
Classification,deep learning,imbalanced learning
AI 理解论文
溯源树
样例
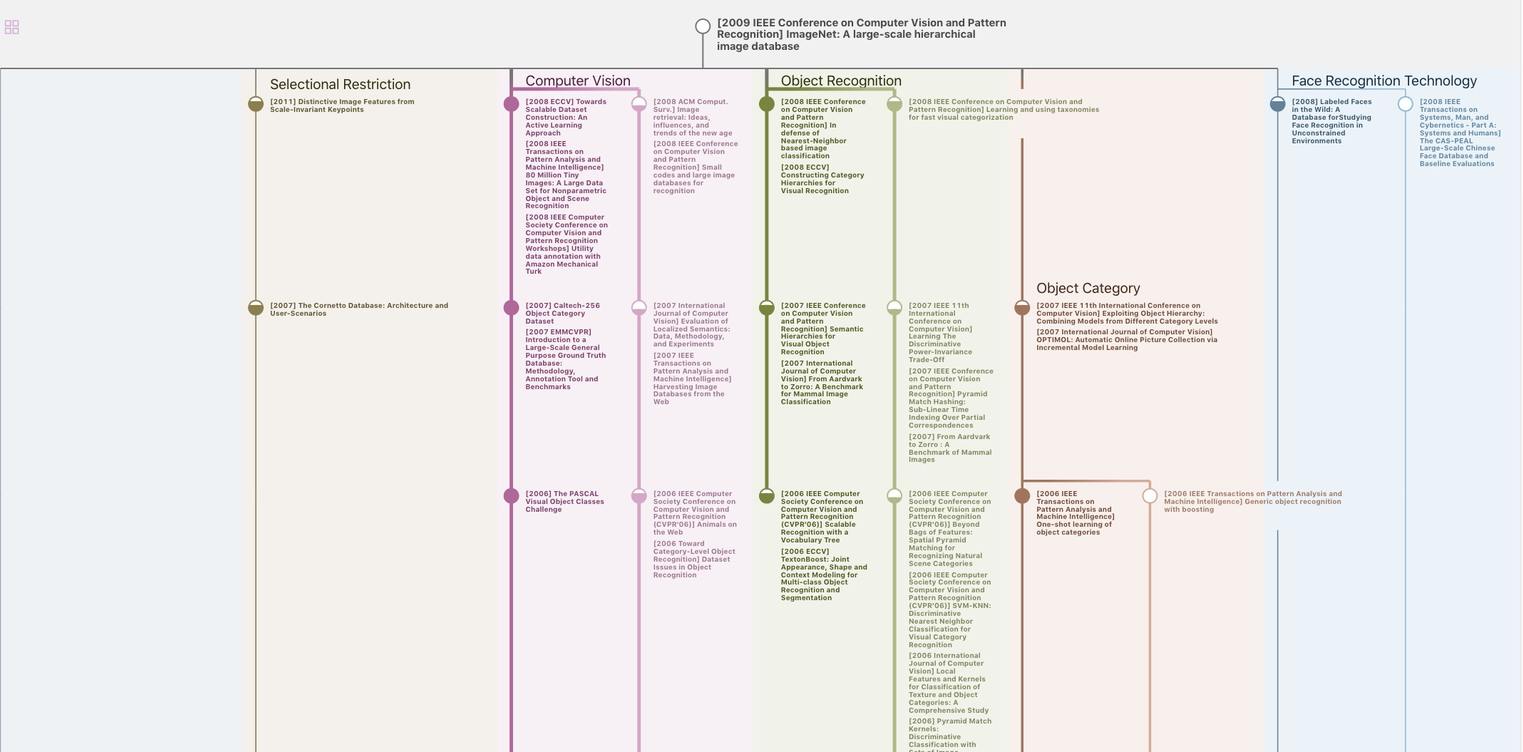
生成溯源树,研究论文发展脉络
Chat Paper
正在生成论文摘要