Decentralized Ensemble Learning Based on Sample Exchange among Multiple Agents.
BSCI(2019)
摘要
Ensemble learning aims to train and combine multiple base learners in the hope of improving the overall performance. Existing ensemble algorithms rely on a centralized framework where each base learner has access to the entire training dataset. We combine the technology of blockchains which is mainly used for data validation with ensemble learning and propose a decentralized framework where data are distributed among multiple base learners, who exchange their respective data to improve the collective predictive abilities. We develop two realizations of this framework, based on static and dynamic decision trees, respectively. We evaluate our methods over 20 real-world datasets and compare them against other centralized ensemble methods. Experimental results show that the proposed method obtains improved accuracy scores through sample exchange and achieves competitive performance with state-of-the-art ensemble methods whereas the base learners store only a small fraction of the samples.
更多查看译文
关键词
Multi-Agent, Decentralize Ensebmle Learning, Interaction
AI 理解论文
溯源树
样例
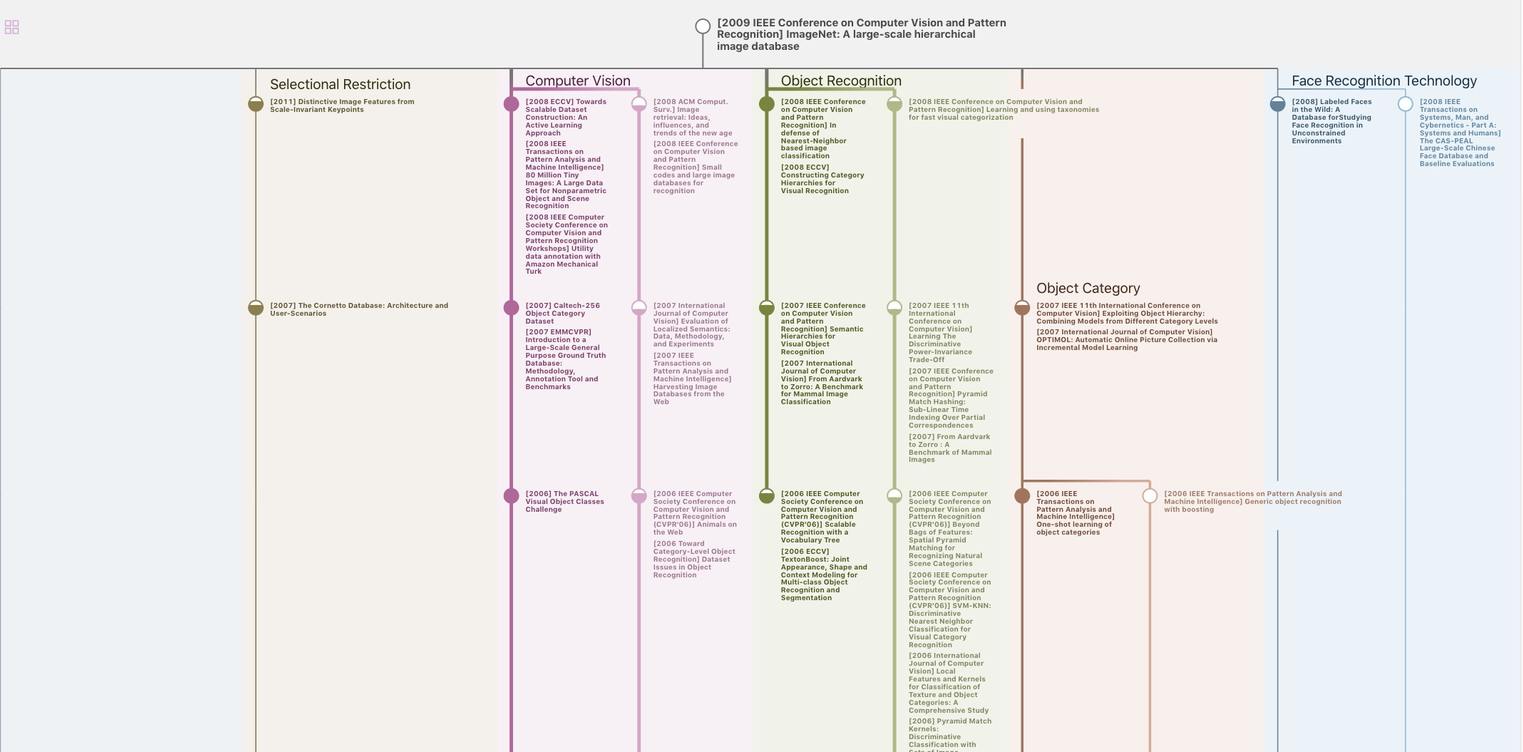
生成溯源树,研究论文发展脉络
Chat Paper
正在生成论文摘要