A Deep Reinforcement Learning Method For Mobile Robot Collision Avoidance Based On Double Dqn
2019 IEEE 28TH INTERNATIONAL SYMPOSIUM ON INDUSTRIAL ELECTRONICS (ISIE)(2019)
摘要
We propose a deep reinforcement learning method based on Double Q-learning Network(DDQN) to enable mobile robots to learn collision avoidance and navigation capabilities autonomously. Information such as target position, obstacle size and position is taken as input, and the direction of movement of the robot is taken as an output. Traditional mobile robots usually requires real-time accurate and fast Simultaneous Localization And Mapping(SLAM) technology for global navigation. We aim at the scenario that after an initial globally feasible path is established, the path could be split into finite segments of sub-goals, and the proposed method focuses on using deep reinforcement learning to control the robots reaching the subgoals in sequence. Experiments show that the proposed method can navigate the mobile robots to desired target position without colliding with any obstacle and other moving robots, and the method is successfully implied on a physical robot platform. In addition, the method is a non-global path planning method, which greatly reduces the computational cost.
更多查看译文
关键词
DDQN, collision avoidance, deep reinforcement, local path planning, SLAM
AI 理解论文
溯源树
样例
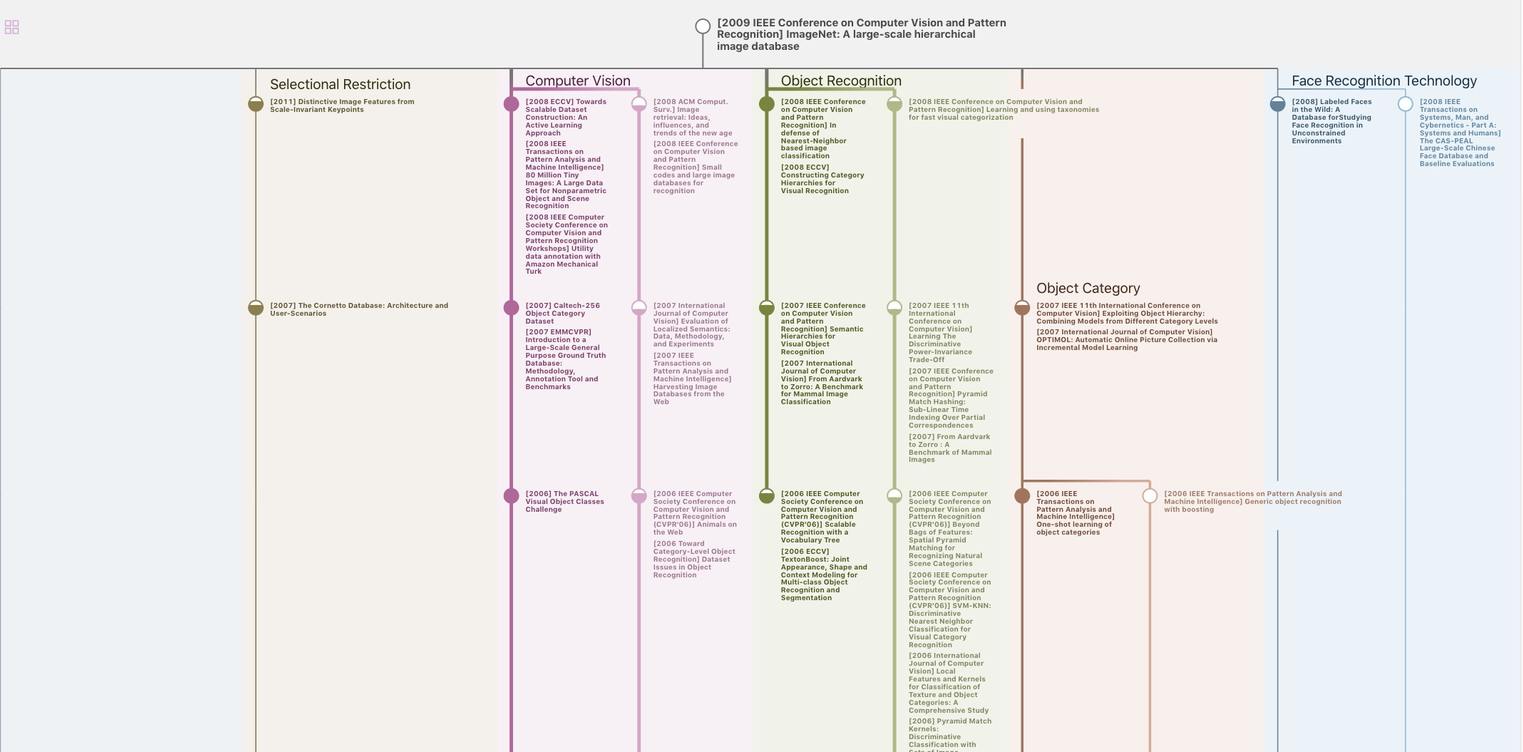
生成溯源树,研究论文发展脉络
Chat Paper
正在生成论文摘要