A Privacy-preserving Method to Optimize Distributed Resource Allocation
SIAM JOURNAL ON OPTIMIZATION(2020)
摘要
We consider a resource allocation problem involving a large number of agents with individual constraints subject to privacy, and a central operator whose objective is to optimize a global, possibly nonconvex, cost while satisfying the agents' constraints, for instance, an energy operator in charge of the management of energy consumption flexibilities of many individual consumers. We provide a privacy-preserving algorithm that computes the optimal allocation of resources, and in which each agent's private information (constraints and individual solution profile) is never revealed either to the central operator or to a third party. Our method relies on an aggregation procedure: we compute iteratively a global allocation of resources, and gradually ensure existence of a disaggregation, that is, individual profiles satisfying agents' private constraints, by a protocol involving the generation of polyhedral cuts and secure multiparty computations. To obtain these cuts, we use an alternate projection method, which is implemented locally by each agent, preserving her privacy needs. We address especially the case in which the local and global constraints define a transportation polytope. Then, we provide theoretical convergence estimates together with numerical results, showing that the algorithm can be effectively used to solve the allocation problem in high dimension, while addressing privacy issues.
更多查看译文
关键词
resource allocation,privacy,alternate projections,aggregation
AI 理解论文
溯源树
样例
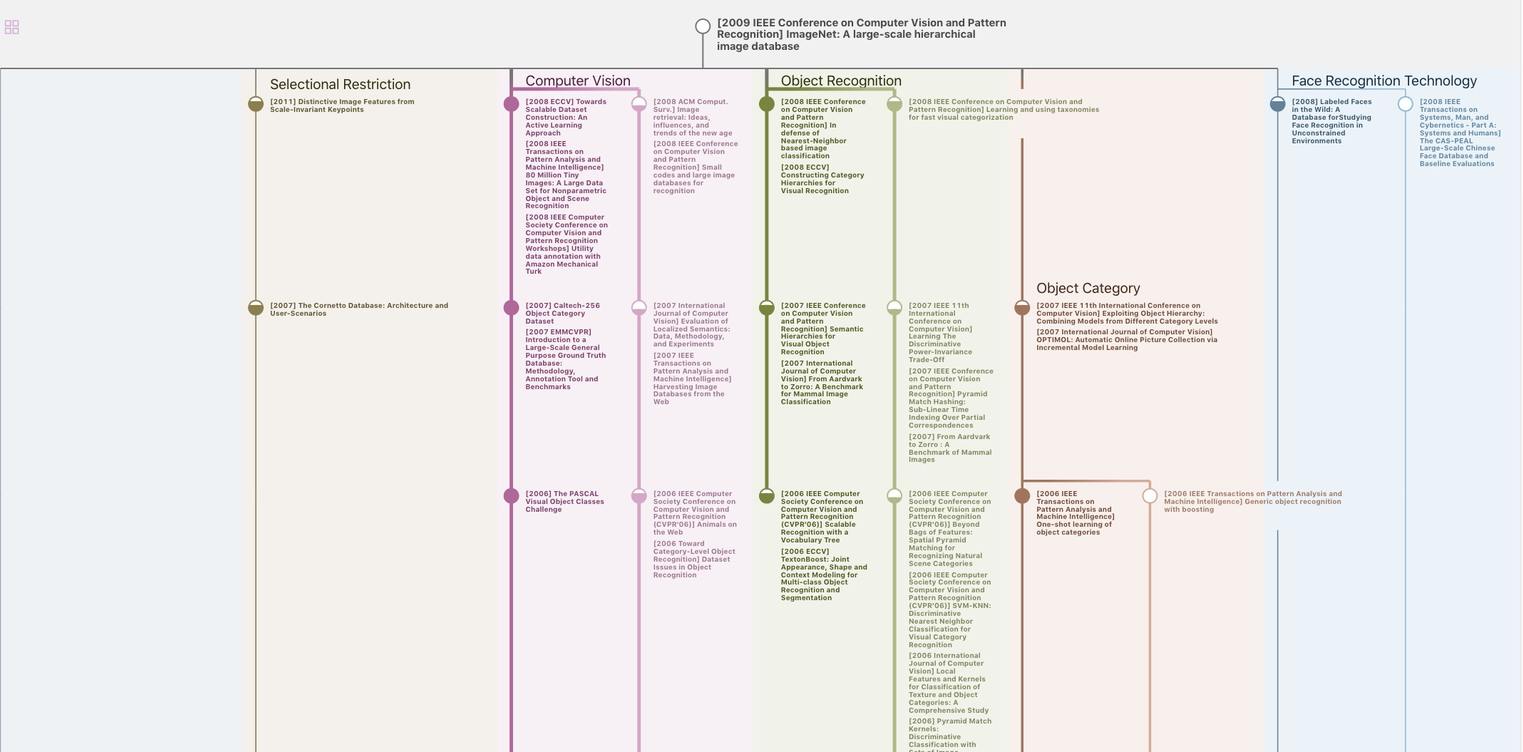
生成溯源树,研究论文发展脉络
Chat Paper
正在生成论文摘要