Certainty of outlier and boundary points processing in data mining
2019 27TH IRANIAN CONFERENCE ON ELECTRICAL ENGINEERING (ICEE 2019)(2018)
摘要
Data certainty is one of the issues in the real-world applications which is caused by unwanted noise in data. Recently, more attentions have been paid to overcome this problem. We proposed a new method based on neutrosophic set (NS) theory to detect boundary and outlier points as challenging points in clustering methods. Generally, firstly, a certainty value is assigned to data points based on the proposed definition in NS. Then, certainty set is presented for the proposed cost function in NS domain by considering a set of main clusters and noise cluster. After that, the proposed cost function is minimized by gradient descent method. Data points are clustered based on their membership degrees. Outlier points are assigned to noise cluster and boundary points are assigned to main clusters with almost same membership degrees. To show the effectiveness of the proposed method, two types of datasets including 3 datasets in Scatter type and 4 datasets in UCI type are used. Results demonstrate that the proposed cost function handles boundary and outlier points with more accurate membership degrees and outperforms existing state of the art clustering methods.
更多查看译文
关键词
Outlier detection, Boundary handling, Neutrosophic set, Fuzzy clustering, Certainty
AI 理解论文
溯源树
样例
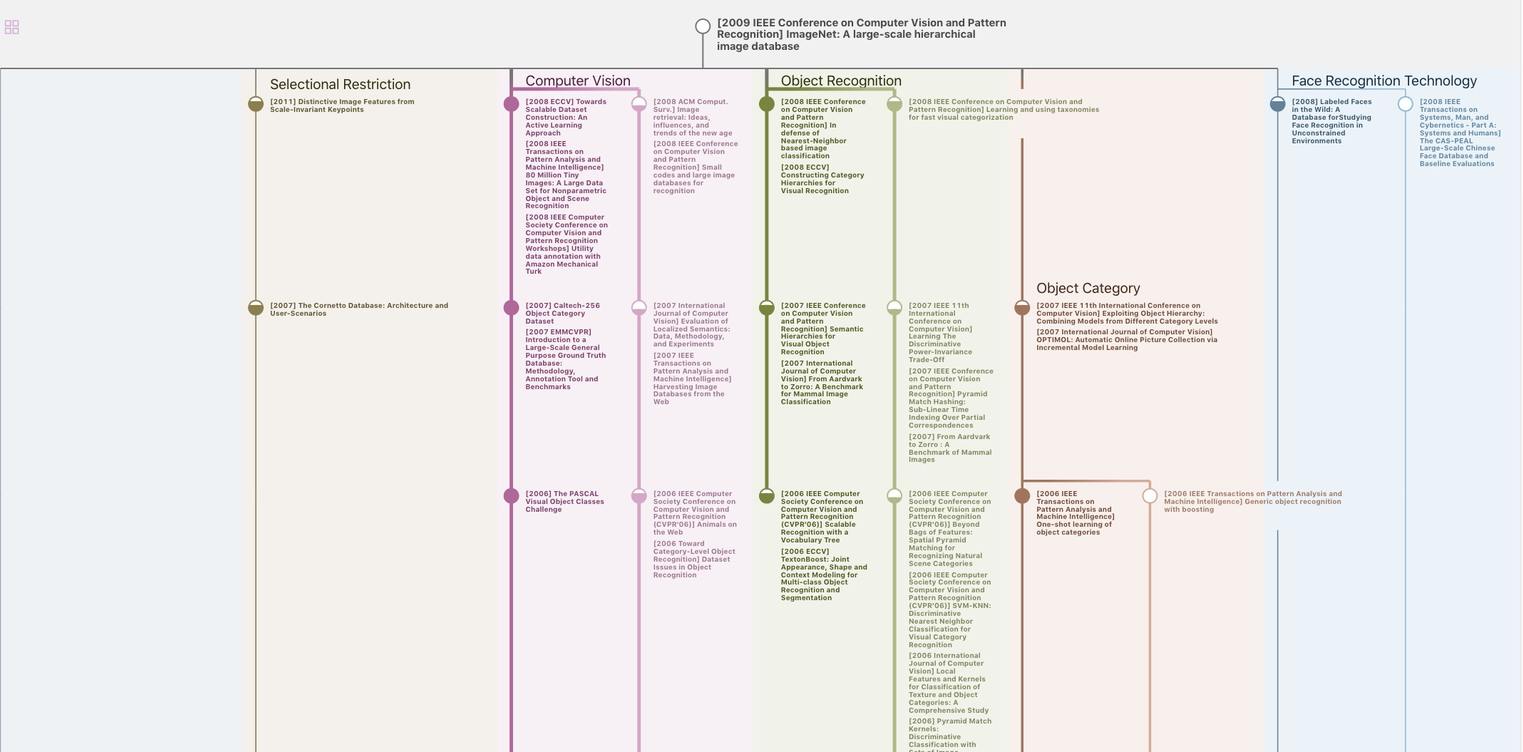
生成溯源树,研究论文发展脉络
Chat Paper
正在生成论文摘要