Inferring Substitutable Products with Deep Network Embedding.
IJCAI(2019)
摘要
On E-commerce platforms, understanding the relationships (e.g., substitute and complement) among products from user's explicit feedback, such as users' online transactions, is of great importance to boost extra sales. However, the significance of such relationships is usually neglected by existing recommender systems. In this paper, we propose a semi-supervised deep embedding model, namely, Substitute Products Embedding Model (SPEM), which models the substitutable relationship between products by preserving the second-order proximity, negative first-order proximity and semantic similarity in a product co-purchasing graph based on user's purchasing behaviours. With SPEM, the learned representations of two substitutable products align closely in the latent embedding space. Extensive experiments on seven real-world datasets are conducted, and the results verify that our model outperforms state-of-the-art base-lines.
更多查看译文
AI 理解论文
溯源树
样例
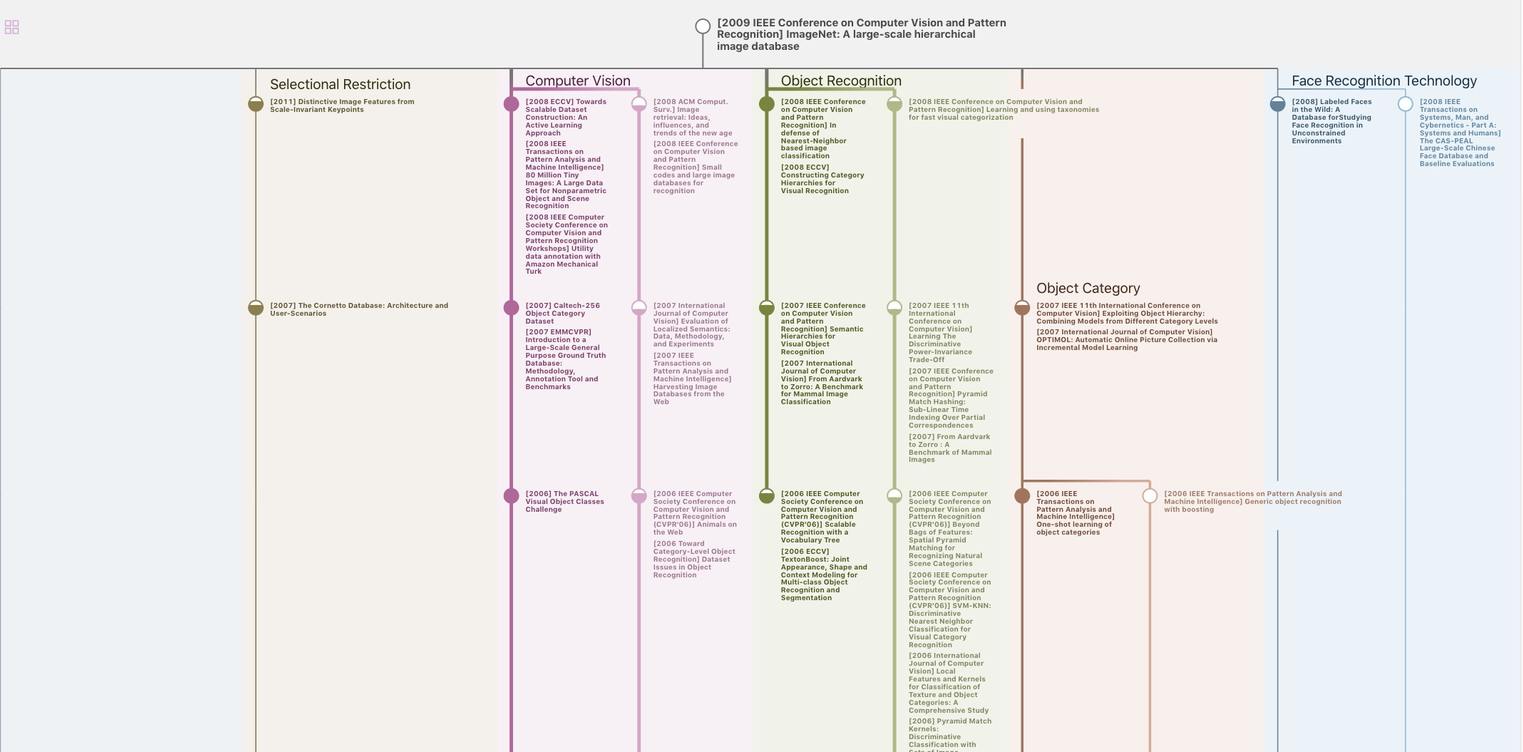
生成溯源树,研究论文发展脉络
Chat Paper
正在生成论文摘要