Balancing Prediction Errors for Robust Sentiment Classification.
ACM Transactions on Knowledge Discovery from Data(2019)
摘要
Sentiment classification is a popular text mining task in which textual content (e.g., a message) is assigned a polarity label (typically positive or negative) reflecting the sentiment expressed in it. Sentiment classification is used widely in applications like customer feedback analysis where robustness and correctness of results are critical. In this article, we highlight that prediction accuracy alone is not sufficient for assessing the performance of a sentiment classifier; it is also important that the classifier is not biased toward positive or negative polarity, thus distorting the distribution of positive and negative messages in the predictions. We propose a measure, called Polarity Bias Rate, for quantifying this bias in a sentiment classifier. Second, we present two methods for removing this bias in the predictions of unsupervised and supervised sentiment classifiers. Our first method, called Bias-Aware Thresholding (BAT), shifts the decision boundary to control the bias in the predictions. Motivated from cost-sensitive learning, BAT is easily applicable to both lexicon-based unsupervised and supervised classifiers. Our second method, called Balanced Logistic Regression (BLR) introduces a bias-remover constraint into the standard logistic regression model. BLR is an automatic bias-free supervised sentiment classifier.
We evaluate our methods extensively on seven real-world datasets. The experiments involve two lexicon-based and two supervised sentiment classifiers and include evaluation on multiple train-test data sizes. The results show that bias is controlled effectively in predictions. Furthermore, prediction accuracy is also increased in many cases, thus enhancing the robustness of sentiment classification.
更多查看译文
关键词
Sentiment analysis,bias-aware sentiment analysis,fairness in learning,lexicon-based methods,supervised methods
AI 理解论文
溯源树
样例
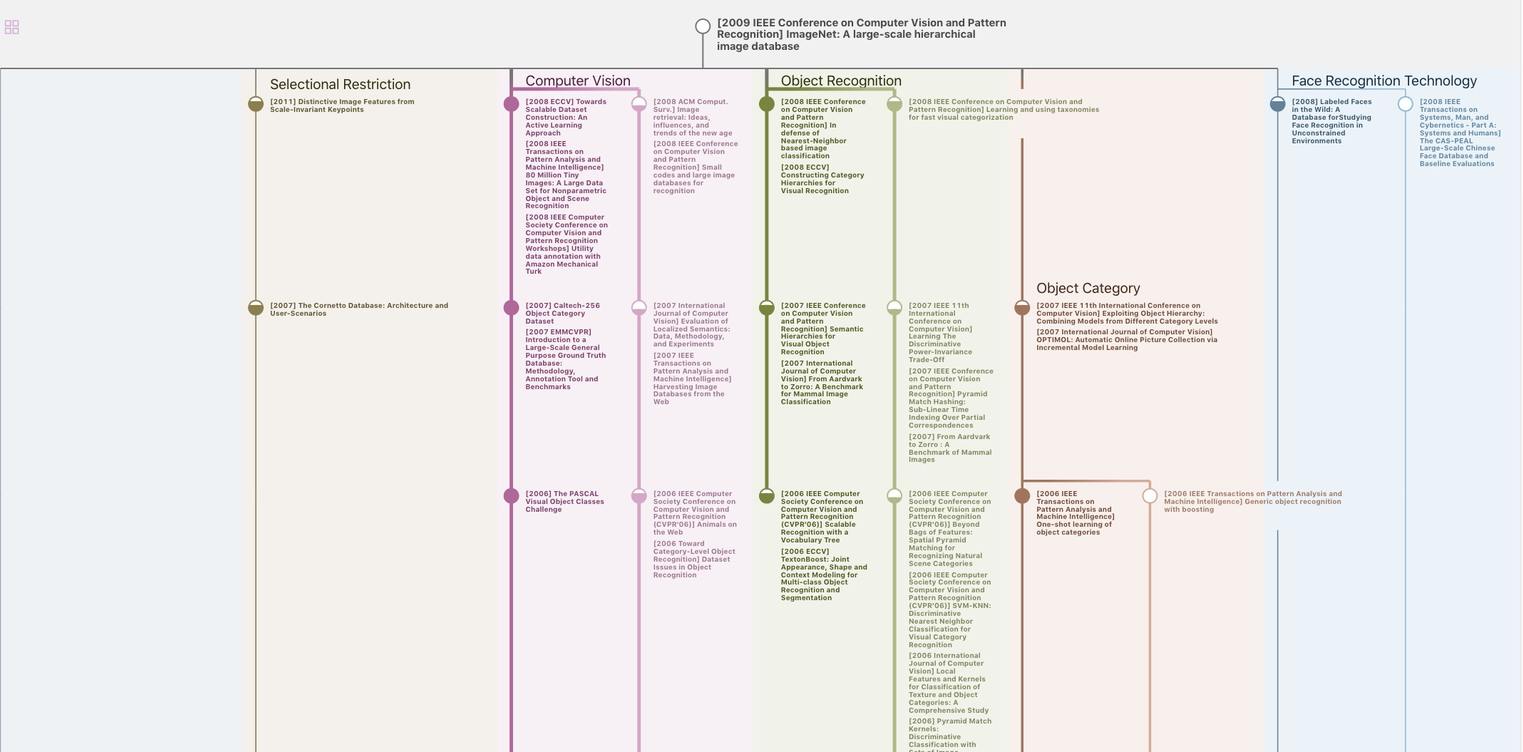
生成溯源树,研究论文发展脉络
Chat Paper
正在生成论文摘要