Neural Blind Deconvolution Using Deep Priors
2020 IEEE/CVF CONFERENCE ON COMPUTER VISION AND PATTERN RECOGNITION (CVPR)(2020)
摘要
Blind deconvolution is a classical yet challenging low-level vision problem with many real-world applications. Traditional maximum a posterior (MAP) based methods rely heavily on fixed and handcrafted priors that certainly are insufficient in characterizing clean images and blur kernels, and usually adopt specially designed alternating minimization to avoid trivial solution. In contrast, existing deep motion deblurring networks learn from massive training images the mapping to clean image or blur kernel, but are limited in handling various complex and large size blur kernels. To connect MAP and deep models, we in this paper present two generative networks for respectively modeling the deep priors of clean image and blur kernel, and propose an unconstrained neural optimization solution to blind deconvolution. In particular, we adopt an asymmetric Autoencoder with skip connections for generating latent clean image, and a fully-connected network (FCN) for generating blur kernel. Moreover, the Soft-Max nonlinearity is applied to the output layer of FCN to meet the nonnegative and equality constraints. The process of neural optimization can be explained as a kind of "zero-shot" self-supervised learning of the generative networks, and thus our proposed method is dubbed Self-Deblur. Experimental results show that our Self-Deblur can achieve notable quantitative gains as well as more visually plausible deblurring results in comparison to state-of-the-art blind deconvolution methods on benchmark datasets and real-world blurry images. The source code is publicly available at https: //github . com/csdwren/SelfDeblur.
更多查看译文
关键词
blur kernel,unconstrained neural optimization solution,fully-connected network,generative networks,blind deconvolution methods,real-world blurry images,neural blind deconvolution,low-level vision problem,posterior based methods,MAP,deep motion deblurring networks,maximum a posterior based methods,unconstrained neural optimization,asymmetric autoencoder,SoftMax nonlinearity,zero-shot self-supervised learning,SelfDeblur
AI 理解论文
溯源树
样例
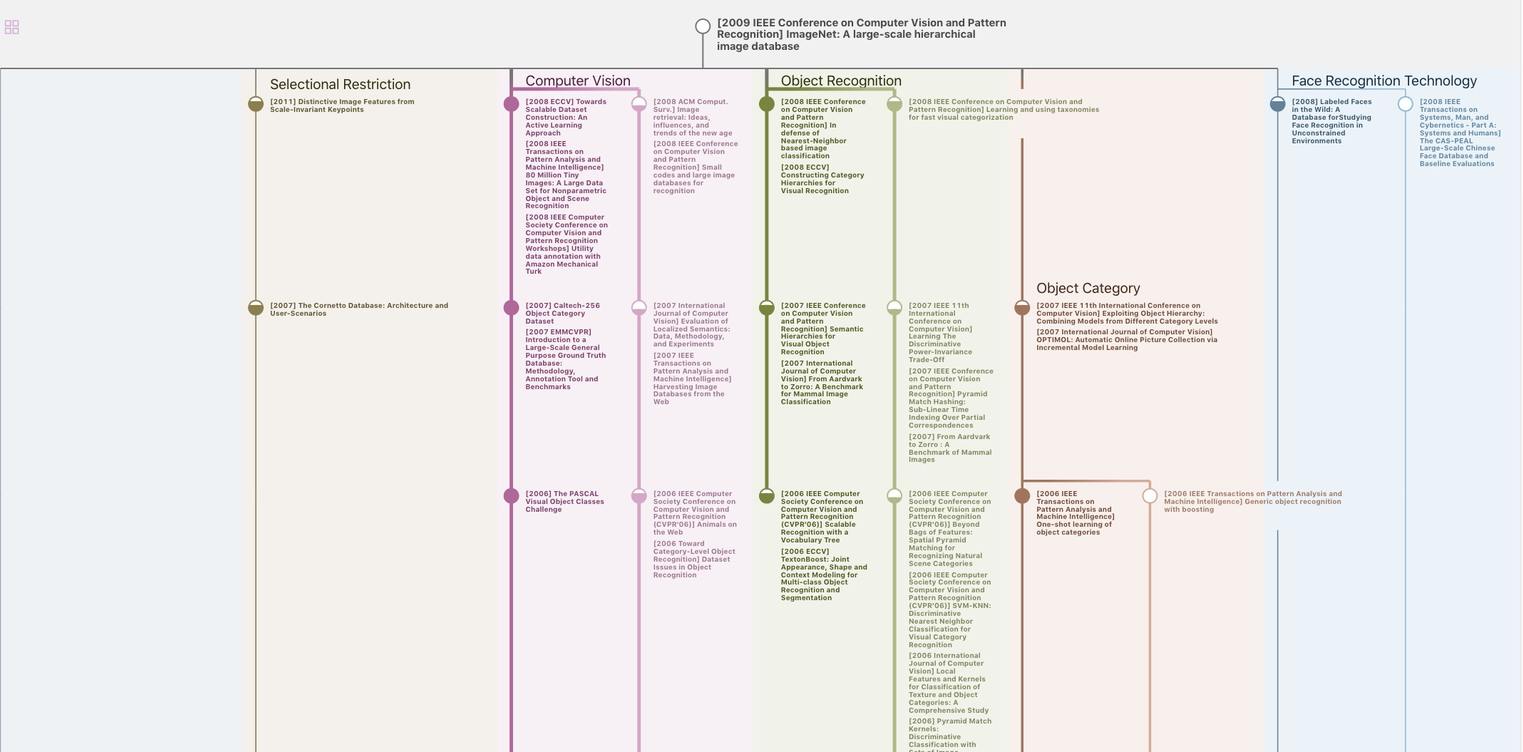
生成溯源树,研究论文发展脉络
Chat Paper
正在生成论文摘要