Deep Self-Learning From Noisy Labels
2019 IEEE/CVF INTERNATIONAL CONFERENCE ON COMPUTER VISION (ICCV 2019)(2019)
摘要
ConvNets achieve good results when training from clean data, but learning from noisy labels significantly degrades performances and remains challenging. Unlike previous works constrained by many conditions, making them infeasible to real noisy cases, this work presents a novel deep self-learning framework to train a robust network on the real noisy datasets without extra supervision. The proposed approach has several appealing benefits. (1) Different from most existing work, it does not rely on any assumption on the distribution of the noisy labels, making it robust to real noises. (2) It does not need extra clean supervision or accessorial network to help training. (3) A self-learning framework is proposed to train the network in an iterative end-to-end manner, which is effective and efficient. Extensive experiments in challenging benchmarks such as Clothing1M and Food101-N show that our approach outperforms its counterparts in all empirical settings.
更多查看译文
关键词
robust network,noisy labels,clean data,deep self-learning framework,ConvNets
AI 理解论文
溯源树
样例
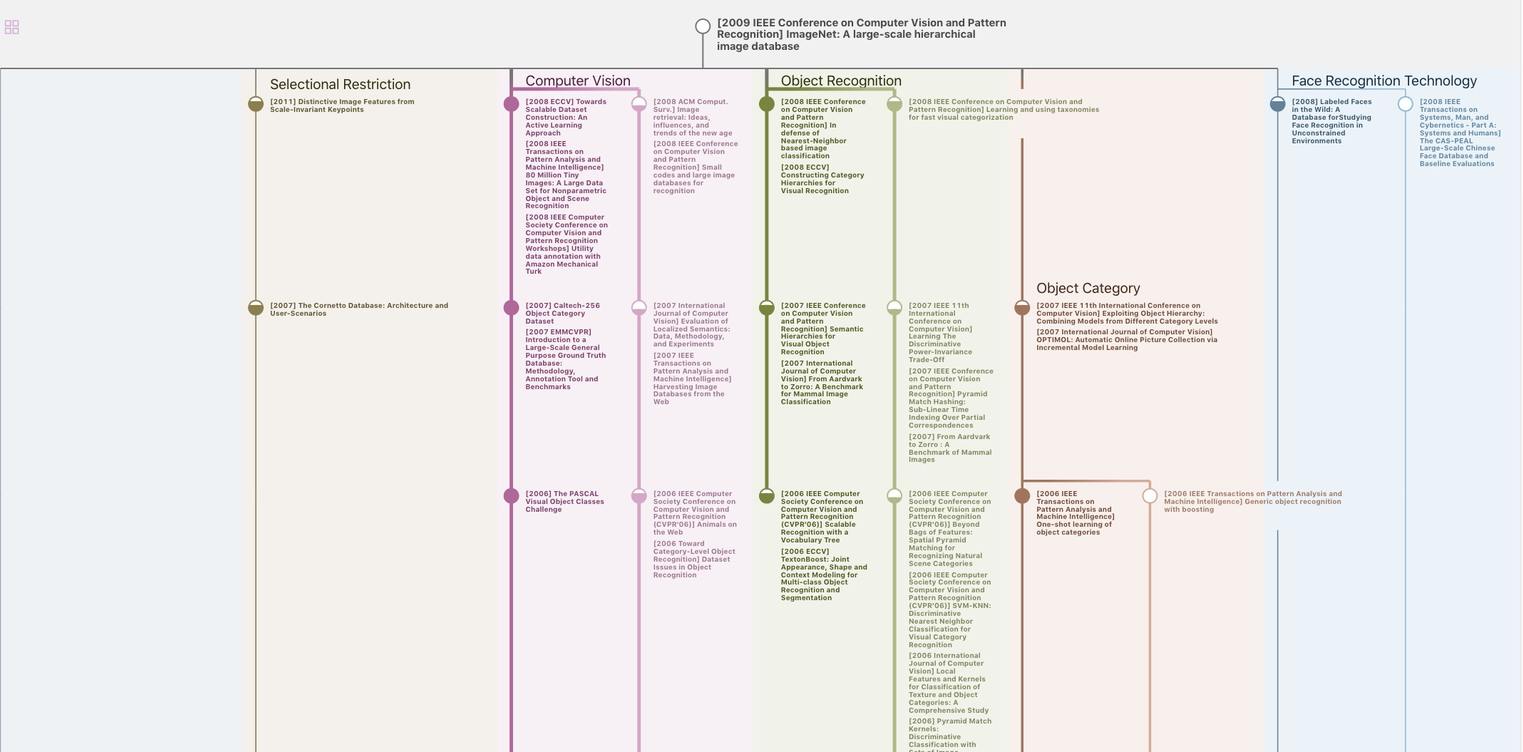
生成溯源树,研究论文发展脉络
Chat Paper
正在生成论文摘要