Model-Based Convolutional De-Aliasing Network Learning for Parallel MR Imaging
MEDICAL IMAGE COMPUTING AND COMPUTER ASSISTED INTERVENTION - MICCAI 2019, PT III(2019)
摘要
Parallel imaging has been an essential technique to accelerate MR imaging. Nevertheless, the acceleration rate is still limited due to the ill-condition and challenges associated with the undersampled reconstruction. In this paper, we propose a model-based convolutional de-aliasing network with adaptive parameter learning to achieve accurate reconstruction from multi-coil undersampled k-space data. Three main contributions have been made: a de-aliasing reconstruction model was proposed to accelerate parallel MR imaging with deep learning exploring both spatial redundancy and multi-coil correlations; a split Bregman iteration algorithm was developed to solve the model efficiently; and unlike most existing parallel imaging methods which rely on the accuracy of the estimated multi-coil sensitivity, the proposed method can perform parallel reconstruction from undersampled data without explicit sensitivity calculation. Evaluations were conducted on in vivo brain dataset with a variety of undersampling patterns and different acceleration factors. Our results demonstrated that this method could achieve encouraging performance in both quantitative and qualitative analysis, compared to three state-of-the-art methods.
更多查看译文
关键词
Parallel MR imaging,Deep learning,Bregman iteration
AI 理解论文
溯源树
样例
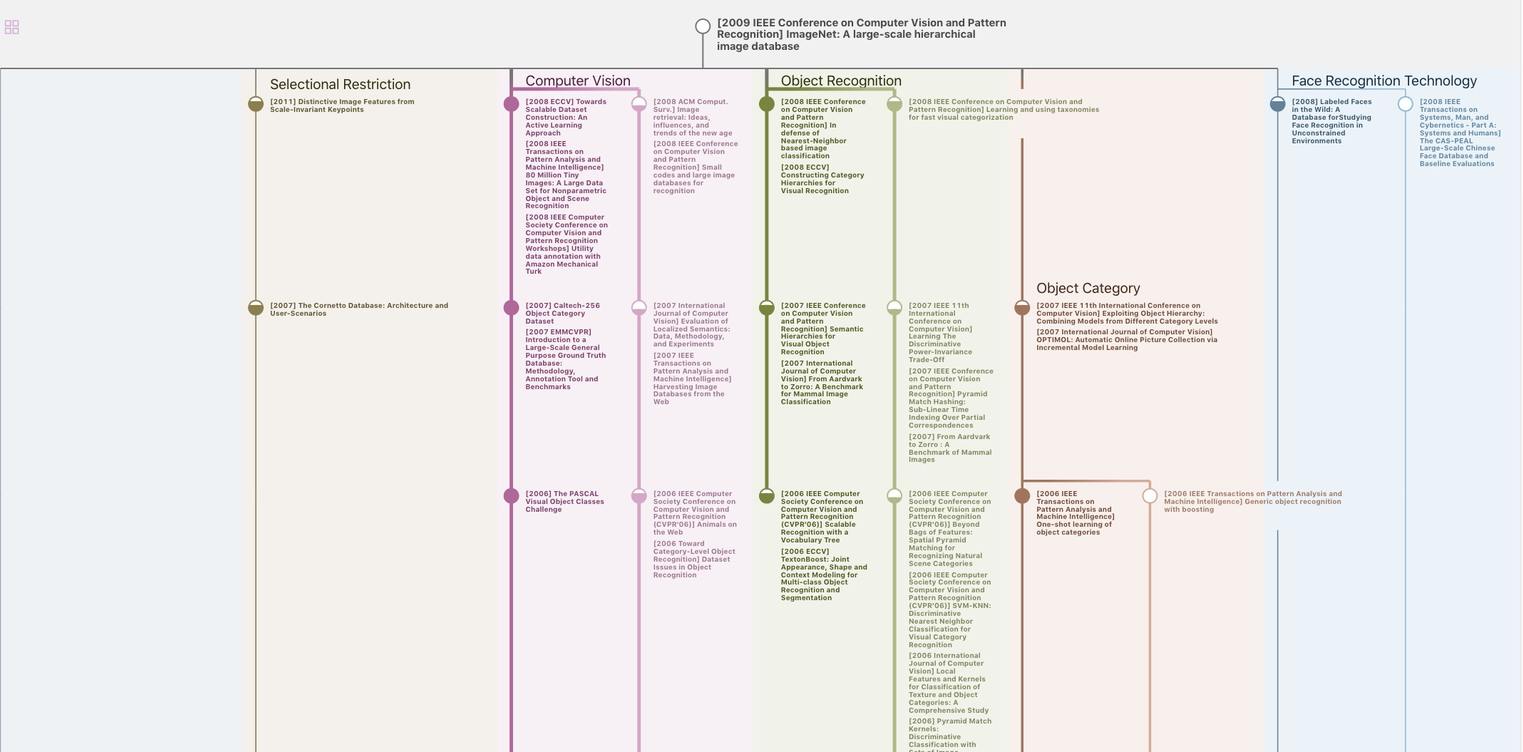
生成溯源树,研究论文发展脉络
Chat Paper
正在生成论文摘要