Diagnosing Vehicles with Automotive Batteries
The 25th Annual International Conference on Mobile Computing and Networking(2019)
摘要
The automotive industry is increasingly employing software- based solutions to provide value-added features on vehicles, especially with the coming era of electric vehicles and autonomous driving. The ever-increasing cyber components of vehicles (i.e., computation, communication, and control), however, incur new risks of anomalies, as demonstrated by the millions of vehicles recalled by different manufactures. To mitigate these risks, we design B-Diag, a battery-based diagnostics system that guards vehicles against anomalies with a cyber-physical approach, and implement B-Diag as an add-on module of commodity vehicles attached to automotive batteries, thus providing vehicles an additional layer of protection. B-Diag is inspired by the fact that the automotive battery operates in strong dependency with many physical components of the vehicle, which is observable as correlations between battery voltage and the vehicle's corresponding operational parameters, e.g., a faster revolutions-per-minute (RPM) of the engine, in general, leads to a higher battery voltage. B-Diag exploits such physically-induced correlations to diagnose vehicles by cross-validating the vehicle information with battery voltage, based on a set of data-driven norm models constructed online. Such a design of B-Diag is steered by a dataset collected with a prototype system when driving a 2018 Subaru Crosstrek in real-life over 3 months, covering a total mileage of about 1, 400 miles. Besides the Crosstrek, we have also evaluated B-Diag with driving traces of a 2008 Honda Fit, a 2018 Volvo XC60, and a 2017 Volkswagen Passat, showing B-Diag detects vehicle anomalies with >86% (up to 99%) averaged detection rate.
更多查看译文
关键词
batteries-as-sensors, cpses, vehicle diagnostics
AI 理解论文
溯源树
样例
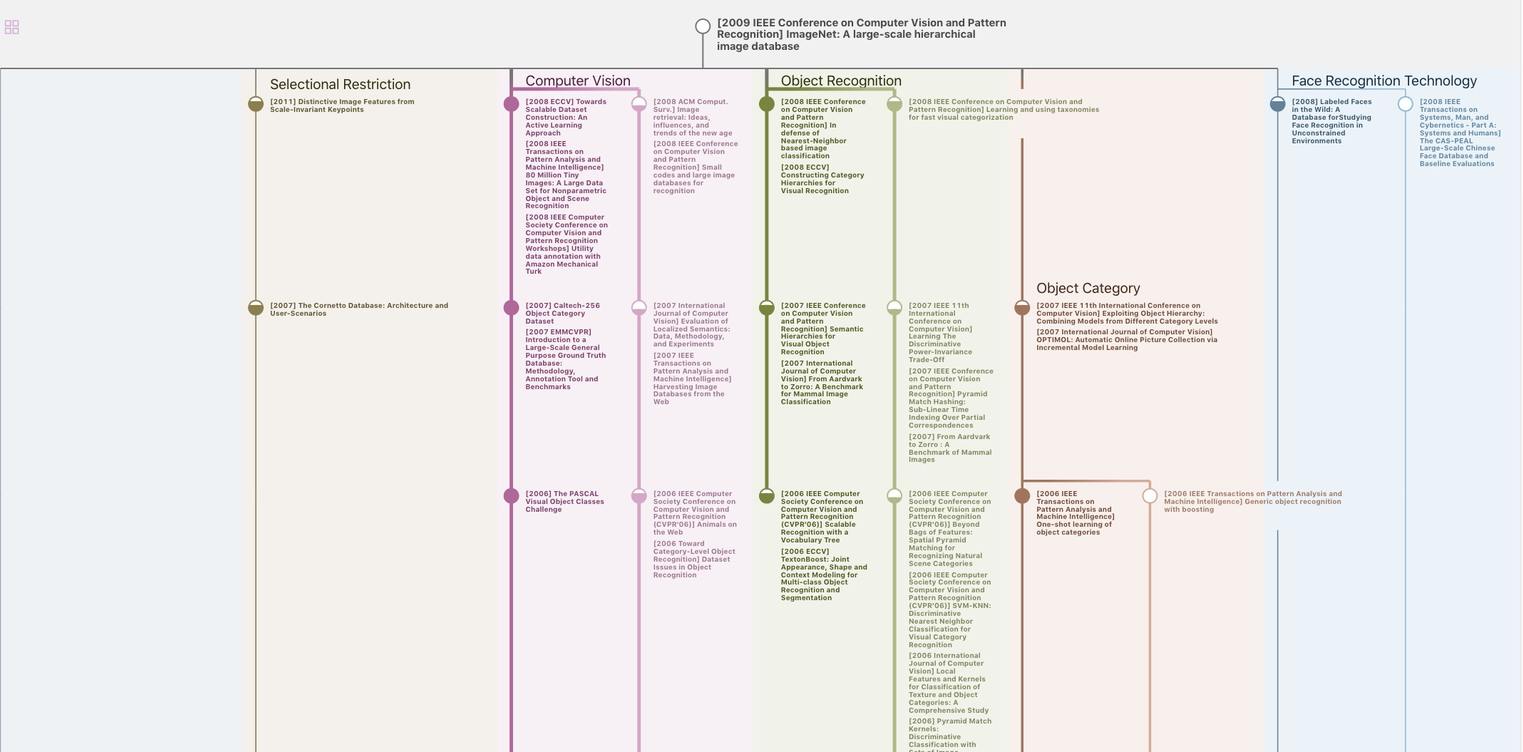
生成溯源树,研究论文发展脉络
Chat Paper
正在生成论文摘要